Contact
Juliane Mai
Department of Earth and Environmental Science
Introduction
Model intercomparison projects test and compare the simulated outputs of various models over the same study domain. The Laurentian Great Lakes region is a domain of high public interest and is a challenging region to model because of its transboundary datasets, climatic and land use conditions, and socio-economic importance to the USA and Canada.
This large-scale hydrologic model intercomparison study included a wide range of researchers who applied models in a highly standardized experimental setup using the same geophysical datasets, forcings, routing scheme and performance evaluation across locations in the Great Lakes region. Unlike most hydrologically focused model intercomparisons, this study not only compared models’ capability to simulate streamflow, but also evaluated the quality of simulated actual evapotranspiration, surface soil moisture and snow water equivalent estimates.
Methodology
The study domain was the the St. Lawrence River watershed which includes the Great Lakes and Ottawa River basins that include about 21% of the world's surface fresh water and 34 million people in the USA and Canada (Figure 1).
Figure 1: Study domain and streamflow gauging locations
The study compared the performance of 13 models of various types, including data driven, land-surface and hydrologic models, conceptual and physically-based, and locally, regionally, and globally calibrated (Table 1). All study models relied on the same set of meteorologic forcings and geophysical datasets, with some models requiring more inputs that others. All models except one used a common routing scheme that contained information for the routing simulation. The Raven modeling framework was used as the routing module by all but one of the models. Models were calibrated and validated temporally, spatially and spatiotemporally to streamflow observations made available by either Water Survey Canada or the U.S. Geological Survey.
Table 1: List of participating models
In order to assess model performance beyond streamflow, actual evapotranspiration, surface soil moisture and snow water equivalent outputs were compared to reference datasets provided by ERA5-Land and GLEAM. Comparisons were performed in two ways; by aggregating model outputs and the reference dataset to the basin level or by re-gridding model outputs to the reference grid and comparing the model simulations at each grid-cell. In addition, a multi-objective analysis to assess a models’ performance across all four variables (streamflow, actual evapotranspiration, surface soil moisture and snow water equivalent) was performed using a Pareto analysis.
Outcomes
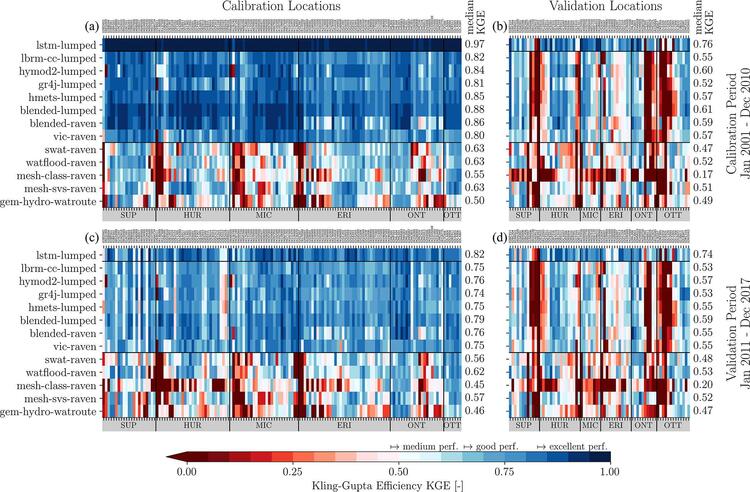
Figure 2: Model performance for streamflow. Panel (a) shows the 141 calibration stations; panel (b) shows the spatial validation, panel (c) shows the temporal validation and panel (d) shows the spatiotemporal validation across the locations (top x axis) and the 13 models (left y axis). The horizontal black lines separate the machine-learning-based global LSTM model from the models that are calibrated locally and the models that are calibrated per region. The performance is quantified using the Kling–Gupta efficiency (KGE). Median performances across the stations are given for each panel (right y axis). The locations are grouped by region they are located in (bottom x axis).
The common assumption that distributed, physically based models are intrinsically of better quality when evaluating model states beyond streamflow was not confirmed in all cases. For actual evapotranspiration, a locally calibrated, conceptual model (HYMOD2-lumped) showed performance equal to more complex models. For surface soil moisture, while physically based models were generally best, the less complex and locally calibrated models led to comparable performances when surface soil moisture was standardized. For snow water equivalent, there were several locally calibrated, conceptual models that performed as well as physically based models (Figure 3).
Figure 3: Basin-wise model performance for (a) actual evapotranspiration, (b) surface soil moisture and (c) snow water equivalent. The Kling–Gupta efficiency (KGE) was used to evaluate the simulations of actual evapotranspiration and snow water equivalent, while the Pearson correlation was used for the standardized surface soil moisture. The horizontal black line separates models that are calibrated locally from models that are calibrated per region. The data-driven LSTM-lumped did not provide outputs for these variables and is hence not displayed.
Comparisons of actual evapotranspiration, surface soil moisture and snow water equivalent outputs against gridded reference datasets showed that aggregating model outputs and the reference dataset to the basin scale can lead to different conclusions than a comparison at the native grid scale. In contrast to basin-wise comparisons, the grid-cell-wise comparisons enhanced the analysis of spatial inconsistencies, which were most apparent in locally calibrated models, and could support targeted model improvements. The comparison of two approaches showed that one needs to consider underlying assumptions when aggregating gridded/distributed model outputs spatially, in particular when basins are of different sizes or variables exhibit large variability in space.
Multi-objective analysis results showed that the locally calibrated models performed better for the calibration basins compared to validation basins. The opposite happened for regionally calibrated models highlighting the stronger spatial robustness of regionally or globally calibrated frameworks.
Conclusions
This study applied a carefully designed framework to consistently compare models of different types. The framework included use of the same data to set up, force and route the models and application of the same methods to assess model performance against streamflow and additional variables. The focus on standardizing datasets and methods led to observed performance differences specific to the model or model building decisions as opposed to differences caused by the quality or inconsistency of input data.
Study results showed that the globally calibrated machine-learning-based LSTM-lumped model was superior for estimating streamflow, that locally calibrated models performed well in calibration and temporal validation and that regionally calibrated models showed stronger spatial robustness in the multi-objective evaluation. Importantly, study data and results were made available through an interactive website and FRDR platform to encourage follow-up work and facilitate the communication of the results to practitioners and stakeholders.
It is expected that the performance of locally calibrated models can be improved with a more sophisticated donor basin mapping strategy and that physically based distributed models could benefit from additional process representation better strategies to perform calibration of large domains.
Read more in Hydrology and Earth System Sciences
Mai, J., Shen, H., Tolson, B. A., Gaborit, É., Arsenault, R., Craig, J. R., Fortin, V., Fry, L. M., Gauch, M., Klotz, D., Kratzert, F., O'Brien, N., Princz, D. G., Rasiya Koya, S., Roy, T., Seglenieks, F., Shrestha, N. K., Temgoua, A. G. T., Vionnet, V., and Waddell, J. W.: The Great Lakes Runoff Intercomparison Project Phase 4: the Great Lakes (GRIP-GL), Hydrology and Earth System Sciences, 26, 2022. https://doi.org/10.5194/hess-26-3537-2022
For more information about WaterResearch, contact Julie Grant.
Great Lakes 2023-02-12 photo by satellite.imagery Iban Ameztoy - Source Terra/MODIS via Flickr.