PLENARY SPEAKERS
Ben Adcock
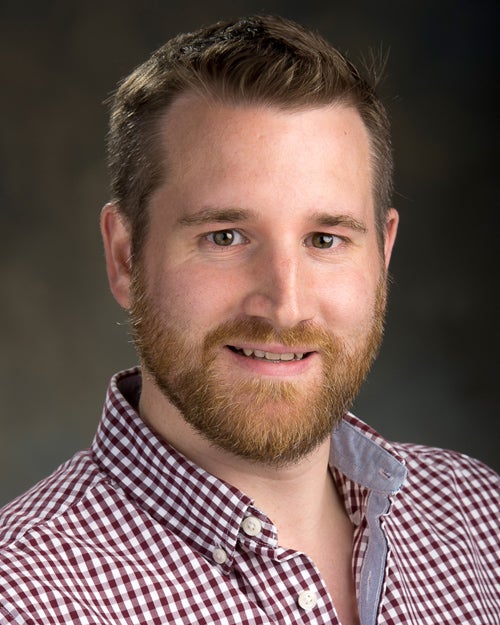
Associate Professor
Department of Mathematics
Simon Fraser University
Tackling the Curse: Polynomial and Deep Neural Network Methods for Function Approximation in High Dimensions
Many problems in computational science and engineering require the accurate approximation of a target function from data. This problem is rendered challenging by the high-dimensionality of the function, the expense of generating function samples, the presence of noise in the measurements, and the fact that the target function may take values in a function space. Developing techniques that tackle these challenges without succumbing to the famous “curse of dimensionality” has been a long-standing problem.
In the first part of this talk I will give a brief survey of a decade?s worth of progress on high-dimensional function approximation via sparse polynomial expansions. I will show how the proper use of compressed sensing tools leads to algorithms for high-dimensional approximation which, unlike other approaches, possess provably near-optimal error bounds and moderate sample complexities. In particular, these techniques mitigate the curse of dimensionality to a substantial degree. The second part of the talk will be devoted to emerging approaches based on deep neural networks and deep learning. Such tools are beginning to garner substantial attention in the scientific computing community. Nonetheless, I will present evidence of a key gap between current theory and practice. I will then discuss recent results showing that there exist deep neural networks that match the performance of best-in-class schemes, and furthermore, these can indeed be trained through realizable procedures. This highlights the potential of deep neural networks, and sheds light on achieving robust, reliable and overall improved practical performance.
This talk is based on joint work with Anyi Bao, Simone Brugiapaglia, Juan M. Cardenas, Nick Dexter, Sebastian Moraga, Yi Sui and Clayton G. Webster.
Biography: Ben Adcock is an Associate Professor in the Department of Mathematics at Simon Fraser University. He received the CAIMS/PIMS Early Career Award (2017), an Alfred P. Sloan Research Fellowship (2015) and a Leslie Fox Prize in Numerical Analysis (2011).
Professor Adcock’s work has been published in venues such SIAM Review, Proceedings of the National Academy of Sciences, Foundations of Computational Mathematics and featured on the cover of SIAM News. His research interests include numerical analysis, mathematics of data science, approximation theory and computational harmonic analysis. He received the CAIMS/PIMS Early Career Award in 2017, an Alfred P. Sloan Research Fellowship in 2015, and a Leslie Fox Prize in Numerical Analysis in 2011. He has published over 40 peer-reviewed journal articles, 15 conference proceedings, and two book chapters.
Professor Adcock is currently PIMS-SFU Site Director and a member of the CAIMS board. He is also a member of the Editorial Board for the SIAM Journal on Scientific Computing, and the SIAM Computational Science and Engineering book series. From 2015–2017 he was secretary of the SIAM Pacific Northwest Section. He is the primary organizer for the 2020 Foundations of Computational Mathematics conference.
Peter Edwin Caines
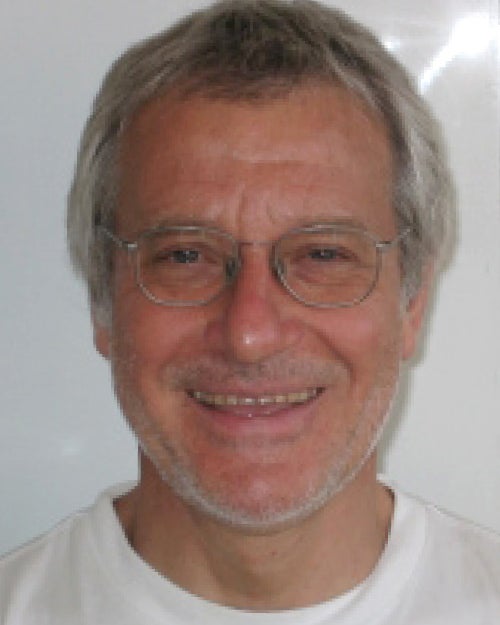
Distinguished James McGill Professor
Department of Electrical and Computer Engineering
Macdonald Chair in the Department of Electrical and Computer Engineering
McGill University
Graphon Mean Field Games: A Dynamical Equilibrium Theory for Large Populations on Complex Networks
The complexity of large population multi-agent dynamical systems, such as occur in economics, communication systems, and environmental and transportation systems, makes centralized control infeasible and classical game theoretic solutions intractable.
In this talk we first present the Mean Field Game (MFG) theory of large population systems. Going to the infinite population limit, individual agent feedback strategies exist which yield Nash equilibria. These are given by the MFG equations consisting of (i) a McKean-Vlasov-Hamilton-Jacobi-Bellman equation generating the Nash values and the best response control actions, and (ii) a McKean-Vlasov-Fokker-Planck–Kolmogorov equation for the probability distribution of the states of the population, otherwise known as the mean field. The applications of MFG theory now extend from economics and finance to epidemiology and physics.
Next we introduce Graphon Mean Field Game and Control theory. Very large scale networks linking dynamical agents are now ubiquitous, with examples being given by electrical power grids and social media networks. In this setting, the emergence of the graphon theory of infinite networks has enabled the formulation of the Graphon Mean Field Game equations, and, in recent work, we have established conditions for the existence and uniqueness of solutions to the GMFG equations. As in the special case of MFG theory, it is the simplicity of the infinite population GMFG strategies which permits, in principle, their application to otherwise intractable problems involving large populations on large complex networks.
Biography: Peter E. Caines received the BA in mathematics from Oxford University in 1967 and the PhD in systems and control theory in 1970 from Imperial College, University of London, supervised by David Q. Mayne, FRS. In 1980, he joined McGill University, Montreal, where he is Distinguished James McGill Professor and Macdonald Chair in the Department of Electrical and Computer Engineering.
In 2000, his paper on adaptive control with G. C. Goodwin and P. J. Ramadge (IEEE TAC, 1980) was recognized by the IEEE Control Systems Society as one of the 25 seminal control theory papers of the 20th century. He received the IEEE Control Systems Society Bode Lecture Prize in 2009, is a Fellow of IFAC, CIFAR, SIAM, IEEE, the IMA (UK) and the Royal Society of Canada (2003), and is a member of Professional Engineers Ontario. Peter Caines is the author of Linear Stochastic Systems (Wiley, 1988), which was republished as a SIAM Classic in June 2018, and is a Senior Editor of Nonlinear Analysis — Hybrid Systems. His research interests include stochastic systems, mean field games and control theory, systems on complex networks and hybrid systems theory, together with their applications to natural and artificial systems.
Arup Chakraborty
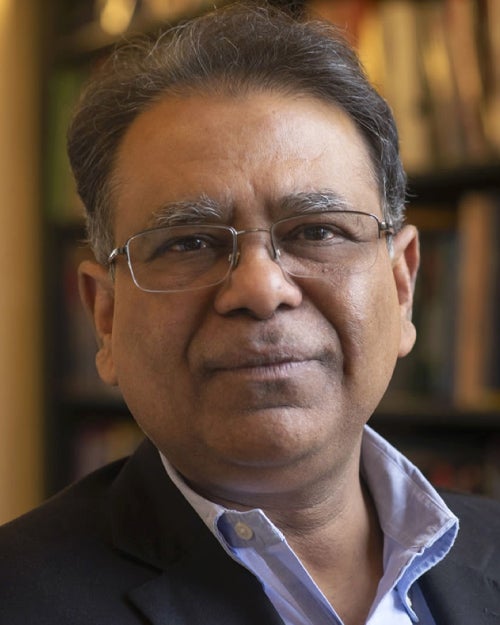
Robert T. Haslam Professor of Chemical Engineering
Professor of Physics and Chemistry
Massachusetts Institute of Technology
Vaccination Strategies for Highly Mutable Pathogens: From Statistical Physics to Monkeys
Efforts to develop effective vaccines against highly mutable pathogens have largely been unsuccessful. HIV is a prominent example. We do not have a universal vaccine that can protect us from diverse strains of influenza either.
I will describe how bringing together theory/computation (rooted in learning algorithms and statistical physics) with basic and clinical immunology can help address such challenges. Using such an approach, we translated data on HIV protein sequences to knowledge of the HIV fitness landscape ? i.e., how the virus? ability to propagate infection depends on its sequence. Predictions emerging from the fitness landscape were then tested against in vitro and clinical data. I will discuss how a potentially potent T cell-based therapeutic vaccine was designed based on these findings and tested positively for immunogenicity in rhesus macaques.
If time permits, I will also describe work aimed toward eliciting antibodies that can protect against diverse strains of highly mutable pathogens. This is a problem at the intersection of statistical physics, immunology, and learning theory.
Biography: Arup K. Chakraborty’s career has focused on work that brings together approaches from different disciplines to understand diverse phenomena and harness that knowledge toward practical ends. He is currently the Robert T. Haslam Professor of Chemical Engineering, and Professor of Physics and Chemistry at MIT. He served as the founding Director of MIT’s Institute for Medical Engineering and Science from February 2012 to January 2018. He is also a founding steering committee member of the Ragon Institute of MIT, MGH, and Harvard, and an Associate Member of the Broad Institute of MIT and Harvard.
After obtaining his PhD in chemical engineering and postdoctoral studies, he joined the faculty at the University of California at Berkeley in December 1988. He rose through the ranks, and ultimately served as the Warren and Katherine Schlinger Distinguished Professor and Chair of Chemical Engineering, Professor of Chemistry, and Professor of Biophysics at Berkeley. He was also Head of Theoretical and Computational Biology at Lawrence Berkeley National Laboratory.
In September 2005, Professor Chakraborty moved to MIT. After an early career in guiding the engineering of polymers and catalysts using quantum and statistical mechanical calculations, since 2000, his work has largely focused on bringing together immunology and the physical and engineering sciences; more specifically, the intersection of statistical mechanics and immunology. His interests span T cell signaling, T cell development and repertoire, and a mechanistic understanding of HIV evolution, antibody evolution, and vaccine design. Since 2016, he has also been deeply interested in the role of phase separation in gene regulation. He is a co-author of the recent book, Viruses, Pandemics, and Immunity, which is intended for a general audience.
Professor Chakraborty’s work at the intersection of disciplines has been recognized by numerous honors, including the NIH Director’s Pioneer Award, the E.O. Lawrence Medal for Life Sciences from the US DOE, a Guggenheim Fellowship, the Allan P. Colburn and Professional Progress awards from the AIChE, a Dreyfus Teacher-Scholar award, and a National Young investigator award.
Professor Chakraborty was elected a member of the National Academy of Sciences and the National Academy of Engineering for completely different bodies of work. He is also a member of the National Academy of Medicine, making him one of 25 individuals who are members of all three branches of the US National Academies, and the only person on this list trained as a physical scientist/engineer whose work is purely rooted in theory/computation. He is a Fellow of the American Academy of Arts & Sciences and the American Association for the Advancement of Science, and serves on the US Defense Science Board. Professor Chakraborty has received five teaching awards for his classroom teaching at Berkeley and MIT. Twenty four of his former lab members are now faculty members at universities around the world.
Stefanie Jegelka
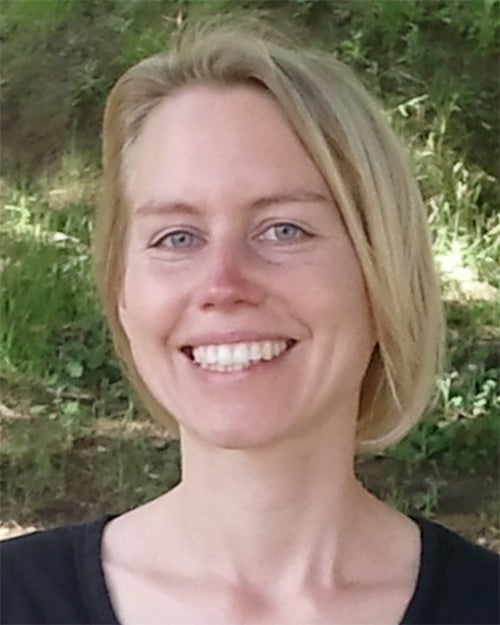
Assistant Professor
X-Consortium Career Development Associate Professor
Electrical Engineering and Computer Science
Massachusetts Institute of Technology
Representation and Learning in Graph Neural Networks
Graph Neural Networks (GNNs) have become a popular tool for learning representations of graph-structured inputs, with applications in computational chemistry, recommendation, pharmacy, reasoning, and many other areas.
In this talk, I will show recent results on representational power and learning in GNNs. First, we will address representational power and important limitations of popular message passing networks and of some recent extensions of these models. Second, we consider learning, and provide new generalization bounds for GNNs. Third, although many networks may be able to represent a task, some architectures learn it better than others. I will show results that connect the architectural structure and learning behavior, in and out of the training distribution.
This talk is based on joint work with Keyulu Xu, Jingling Li, Mozhi Zhang, Simon S. Du, Ken-ichi Kawarabayashi, Vikas Garg and Tommi Jaakkola.
Biography: Stefanie Jegelka is an X-Consortium Career Development Associate Professor in the Department of EECS at MIT. She is a member of the Computer Science and AI Lab (CSAIL), the Center for Statistics and an affiliate of IDSS and ORC.
Before joining MIT, she was a postdoctoral researcher at UC Berkeley, and obtained her PhD from ETH Zurich and the Max Planck Institute for Intelligent Systems.
Professor Jegelka has received a Sloan Research Fellowship, an NSF CAREER Award, a DARPA Young Faculty Award, a Google research award, a Two Sigma faculty research award, the German Pattern Recognition Award and a Best Paper Award at the International Conference for Machine Learning (ICML). Her research interests span the theory and practice of algorithmic machine learning.
Kees Oosterlee
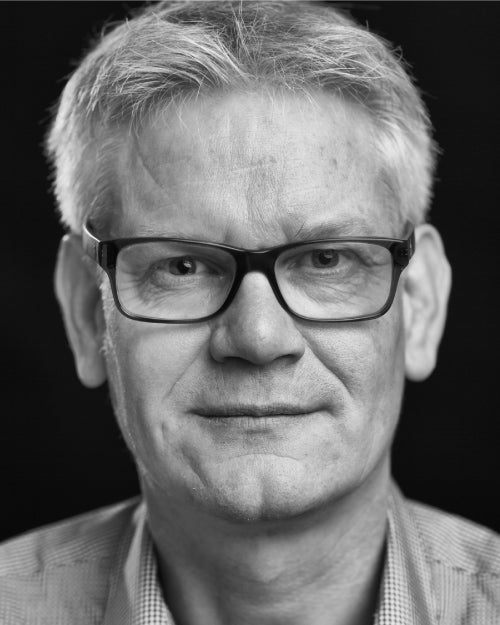
Professor
Mathematical Institute
Utrecht University
The Seven-League Scheme: Deep Learning for Large Time Step Monte Carlo Simulations of Stochastic Differential Equations
We propose an accurate data-driven numerical scheme to solve Stochastic Differential Equations (SDEs), on the basis on using large time steps. The SDE discretization is based on a polynomial chaos expansion method, and accurately determined stochastic collocation (SC) points. By an artificial neural network these SC points are learned. We then perform Monte Carlo simulations with large time steps. Error analysis confirms that this data-driven scheme results in accurate SDE solutions in the sense of strong convergence, provided the learning methodology is robust and accurate. With a variant method called the compression-decompression collocation and interpolation technique (CDC), we reduce the number of neural network functions that have to be learned, so that computational speed is enhanced. Numerical results show high quality strong convergence error results, when using large time steps, and the novel scheme outperforms some classical numerical SDE discretizations. Some applications, in financial option valuation, are presented.
Biography: Professor Kees Oosterlee has been working on computational problems in finance for 20 years now. Since 2007 he has been a part-time professor in Delft and also a scientist at the Centrum Wisknude & Informatica (CWI) in Amsterdam, where he was also a member of the management team since 2012. From this year on, he holds a chair position at Utrecht University, Mathematical Institute. His main research interests include Fourier expansions, Monte Carlo methods and, recently, also, machine learning in finance.
Professor Oosterlee is co-author of two textbooks (Multigrid, 2001 and Mathematical Modeling and Computation in Finance, 2019), and many scientific publications. Methods he co-developed include the COS method, SWIFT (Shannon Wavelet Inverse Fourier Transform method), SGBM (Stochastic Grid Bundling Method), SCMC (Stochastic Collocation Monte Carlo Method), and the Seven-League scheme (7L).
He was the editor-in-chief of the Journal of Computational Finance, 2013–2018, and has been teaching guest lectures at Oxford University, Hitotsubashi University, Japan, University of A Coruña Spain, among others.
Hansi Alice Singh
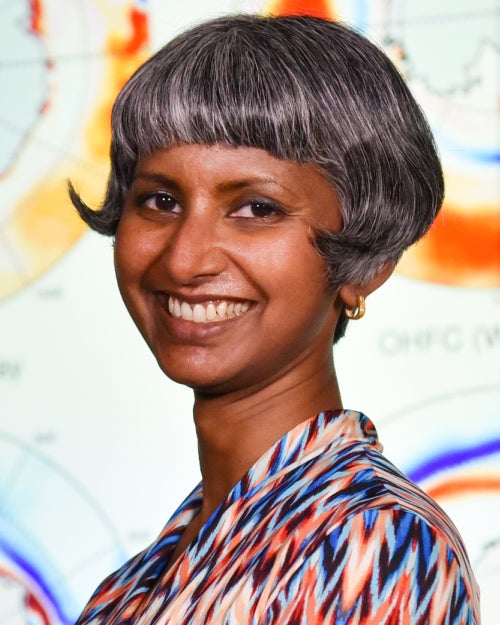
Assistant Professor
School of Earth and Ocean Sciences
University of Victoria
Adventures in the Antarctic: Using Earth System Models to Explore the Continent, Southern Ocean, and Sea Ice
Biography: Hansi Singh is an Assistant Professor in the School of Earth and Ocean Sciences at the University of Victoria. Her research is focused on the physical climate system, particularly the myriad of interactions between atmosphere, ocean, and ice that give rise to the Earth’s climate. She is especially interested in the ever-evolving climate of the polar regions, both Antarctic and Arctic, as well as the large-scale transport of atmospheric water from equator to pole. Her tools are primarily models — from global climate models executed on supercomputers to simple heuristic models that can be analyzed with pencil and paper. Her publications range over a broad set of climate and paleo-climate related topics with several high impact recent contributions in Geophysical Research Letters.
Dr. Singh is a co-chair of the Polar Climate Working Group of the Community Earth System Model, whose development hub is at the National Center for Atmospheric Research in Boulder, Colorado, USA. She received her PhD from the University of Washington in 2016, where she held a US Department of Energy Computational Science Graduate Fellowship. Prior to joining the University of Victoria, she was a Linus Pauling Distinguished Postdoctoral Fellow at Pacific Northwest National Laboratory, sponsored by US Department of Energy Office of Science.
CAIMS SPONSORS
With thanks and gratitude to our sponsors