The Data Systems Seminar Series provides a forum for presenting and discussing key issues in data systems, both current and emerging. It complements our internal meetings by welcoming insights from external colleagues.
The schedule for the 2024–25 academic year is outlined below and will be updated as additional speakers are confirmed.
Seminars are typically held on Mondays at 10:30 a.m. in DC 1302 during the winter 2025 term, unless otherwise noted. Some sessions may be held virtually on Zoom; these will be clearly marked.
The talks are open to the public.
We will post the presentation videos whenever possible. Past DSG Seminar videos can be found on the DSG YouTube channel.
The Data Systems Seminar Series is supported by
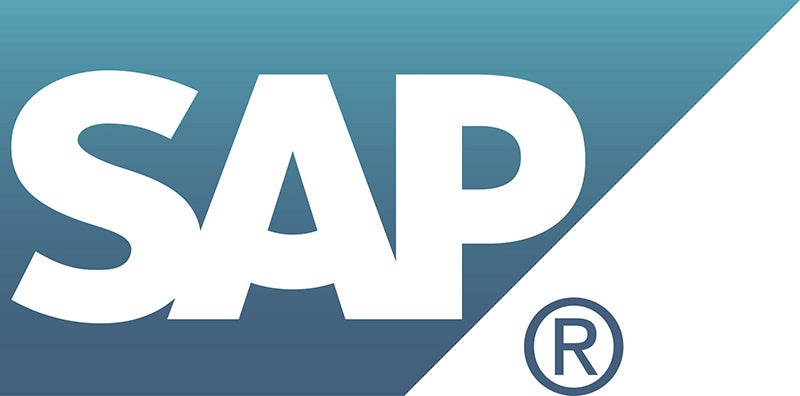
Kris Shrishak |
Arijit Khan |
Tilmann Rabl |
Miao Qiao |
Philip Bernstein |
Weiran Liu |
Jianguo Wang |
Thomas Haigh |
Ricardo Baeza-Yates |
Jeff Dalton |
Lingyang Chu |
26 September 2024; 2pm (Note special time)
Title: | Privacy and PETs: An Interaction with Human Rights Law |
Speaker: | Kris Shrishak, Enforce |
Abstract: | Privacy enhancing technologies (PETs) have been researched and promoted for the past few decades. Amidst greater public awareness of personal data collection and application of data protection regulations, the number of implementations of PETs have increased in the past few years. Given the promise and expectation of PETs to protect people’s privacy and the hope of researchers to see use-cases of PETs, what is the reality of PETs in today’s world? Are the privacy needs of people being met? This talk will take you through a journey of PETs, visiting data protection law and international human rights law along the way. |
Bio: | Dr. Kris Shrishak is a public interest technologist and a Senior Fellow at Enforce. He advises legislators on emerging technologies and global AI governance (including EU AI Act). He is regularly invited to speak at the European Parliament and has testified at the Irish Parliament. His work focuses on privacy tech, anti-surveillance, emerging technologies, and algorithmic decision making. His expert commentary appears in The New York Times, The Washington Post, the BBC, the LA Times, Süddeutsche Zeitung, Politico, The Irish Times and other leading media. He has been interviewed on TV and radio, including on CNN, the BBC, Euronews and France24. He has written for Bulletin of Atomic Scientists, Nikkei Asia and Euronews, among others. He works on the kind of cryptography that allows computing on encrypted data and proving existence of information without revealing them. These technologies, broadly known as privacy enhancing technologies (PETs), could be beneficial. However, there are risks that have not been sufficiently researched. Previously Kris was a researcher at Technical University Darmstadt in Germany where he worked on applied cryptography, PETs and Internet infrastructure security. |
7 October 2024; 10:30
Title: | User-friendly Explanations for Graph Neural Networks ![]() |
Speaker: | Arijit Khan, Aalborg University |
Abstract: | Graph data, e.g., social and biological networks, financial transactions, and knowledge graphs are pervasive in the natural world, where nodes are entities with features, and edges denote relations among them. Machine learning and recently, graph neural networks (GNNs) become ubiquitous, e.g., in cheminformatics, bioinformatics, fraud detection, question answering, and recommendation. However, GNNs are “black-box” - it remains a desirable yet nontrivial task to explain the results of high-quality GNNs for domain experts. In this talk, I shall introduce our ongoing works about how data management techniques can assist in generating user-friendly, configurable, queryable, and robust explanations for graph neural networks. |
Bio: | Arijit Khan is an Associate Professor at Aalborg University, Denmark. His PhD is from University of California, Santa Barbara, USA, and he did a post-doc in the Systems group at ETH Zurich, Switzerland. He has been an assistant professor in the School of Computer Science and Engineering, Nanyang Technological University, Singapore. His research is on data management and machine learning for the emerging problems in large graphs. He is an IEEE senior member and an ACM distinguished speaker. Arijit is the recipient of the IBM Ph.D. Fellowship (2012-13), a VLDB Distinguished Reviewer award (2022), and a SIGMOD Distinguished PC award (2024). He is the author of a book on uncertain graphs and over 80 publications in top venues including ACM SIGMOD, VLDB, IEEE TKDE, IEEE ICDE, SIAM SDM, USENIX ATC, EDBT, The Web Conference (WWW), ACM WSDM, ACM CIKM, ACM TKDD, and ACM SIGMOD Record. Dr Khan is serving as an associate editor of IEEE TKDE 2019-2024 and ACM TKDD 2023-now, proceedings chair of EDBT 2020, IEEE ICDE TKDE poster track co-chair 2023, ACM CIKM short paper track co-chair 2024, and IEEE ICDE demonstration paper track program co-chair 2025. |
21 October 2024; 10:30
Title: | Efficiency in Data Systems |
Speaker: | Tilmann Rabl, University of Potsdam |
Abstract: | For the longest time, acquiring new hardware resulted in significant software efficiency gains due to exponential improvements of hardware capabilities. Physical limits in hardware manufacturing have brought former niche designs into standard components, such as multiple cores and specialized circuits. Even with these new designs, hardware improvements are decreasing, while software and applications are still becoming increasingly complex and resource demanding. In this talk, we will discuss efficiency of data systems. We will start with a general discussion of system efficiency and look at the design of efficient architectures. Incorporating estimations on hardware and power production carbon intensity, we will then discuss hardware replacement frequencies and try to establish new rules of thumb on the ideal hardware lifecycles for database deployments and discuss implications on database development. |
Bio: | Tilmann Rabl is a professor for Data Engineering Systems at the Digital Engineering Faculty of the University of Potsdam and the Hasso Plattner Institute. His research focuses on efficiency of database and ML systems, real-time analytics, hardware efficient data processing, and benchmarking. |
28 October 2024; 10:30
Title: | Scalable Query Processing with Graphs |
Speaker: | Miao Qiao, University of Auckland |
Abstract: | Graph-based query processing faces scalability challenges. This talk explores two facets of the problem. First, when a graph grows too large for efficient querying, can query processing algorithms exhibit strongly local properties, making the search independent of the graph’s overall size? Second, in approximate nearest neighbor search using indexes of Hierarchical Navigable Small World (HNSW) graphs, how can we compress the index while maintaining equivalent query performance, when queries include attribute-based filters? To address the first question, we examine cases where dense subgraph search admits strongly local algorithms and where it does not. For the second, we present a novel compression method that transforms the n^2 HNSW graphs into a more compact structure called the 2D segment graph, enabling lossless compression while preserving query efficiency. Theory plays a central role in both solutions, shaping the performance and feasibility of scalable graph-based querying. |
Bio: | Dr. Qiao is a Senior Lecturer in Computer Science at the University of Auckland, New Zealand, a role equivalent to Associate Professor in tenure-track systems. Her research centers on big data management, with a focus on query optimization, indexing, joins, sampling, graph analysis, and graph-based nearest neighbor search. She has advanced indexing techniques for query processing in graph databases, including shortest distance and subgraph matching queries. Her recent work on range-filtering nearest neighbor search, along with an ongoing submission on its dynamic variant, has potential applications in modern vector databases, particularly for unstructured queries. |
13 December 2024; 11:00 (Note the unusual day and time)
Title: | DDS: DPU-optimized Disaggregated Storage![]() |
Speaker: | Philip Bernstein, Microsoft Research |
Abstract: |
A DPU is a network interface card (NIC) with programmable compute and memory resources. It sits on the system bus, PCIe, which is the fastest path to access SSDs, and it directly connects to the network. It therefore can process storage requests as soon as they arrive at the NIC, rather than passing them through to the host. DPUs are widely deployed in public clouds and will soon be ubiquitous. In this talk, we’ll describe DPU-Optimized Disaggregated Storage (DDS), our software platform for offloading storage operations from a host storage server to a DPU. It reduces the cost and improves the performance of supporting a database service. DDS heavily uses DMA, zero-copy, and userspace I/O to minimize overhead and thereby improve throughput. It also introduces an offload engine that can directly execute storage requests on the DPU. For example, it can offload GetPage@LSN to the DPU of an Azure SQL Hyperscale page server. This removes all host CPU consumption (saving up to 17 cores), reduces latency by 70%, and increases throughput by 75%. This is joint work with Qizhen Zhang, Badrish Chandramouli, Jason Hu, and Yiming Zheng. |
Bio: | Philip A. Bernstein is a Distinguished Scientist in the Data Systems Group in Microsoft Research. He has published over 200 papers and two books on the theory and implementation of database systems, especially on transaction processing and data integration, and has contributed to many database products. He is a Fellow of the ACM and AAAS, a winner of the E.F. Codd SIGMOD Innovations Award, and a member of the Washington State Academy of Sciences and the National Academy of Engineering. He received a B.S. degree from Cornell and M.Sc. and Ph.D. from University of Toronto. |
29 January 2025; 12:00 (Note the unusual day and time)
Title: | Efficient Simple Keyword Private Information Retrieval ![]() |
Speaker: | Weiran Liu, Alibaba Group |
Abstract: | Keyword Private Information Retrieval (Keyword PIR) enables private queries on public key-value databases. Unlike standard index-based PIR, keyword PIR presents greater challenges, since the query’s position within the database is unknown and the domain of keywords is vast. The key insight to obtain efficient keyword PIR is to construct an efficient and compact key-to-index mapping, thereby reducing the keyword PIR problem to standard PIR. In this talk, I will introduce the basic concept of (Keyword) PIR, the state-of-the-art (SOTA) index/keyword PIR constructions based on Learning With Error (LWE) assumptions, and our new constructions on more efficient Keyword PIR. Notably, our construction includes several advanced data structures coming from the database field, i.e., binary fuse filter and learned index, demonstrating that new data structures can have potential applications in crypto primitives. |
Bio: | Weiran Liu received his B.S. degree in Electronic Information and Engineering from Beihang University, China, in 2012 and his Ph.D. degree in Information and Communication Engineering from Beihang University, China, in 2017. He is currently a staff security engineer at the Department of Data Technology and Products, Alibaba Group, China. His main areas of interest include applied cryptography, fully homomorphic encryption, secure multi-party computation, and differential privacy. He has published several works on top-tier conferences such as USENIX Security, ACM CCS, SIGMOD, VLDB, ICDE, and PKC. He also contributed several books on the data security field, including “The Greate Crypto,” “A Pragmatic Introduction to Secure Multi-Party Computation (Chinese version),” and “Programming Differential Privacy (Chinese version).” He served as reviewer/extended reviewer for top-tier international conferences across different fields of studies, including ICML, NIPS, ICLR, and ASIACRYPT. |
31 March 2025; 1:30 in DC 1304 (Note the unusual time and location)
Title: | Database Systems for LLMs: Vector Databases and Beyond ![]() |
Speaker: | Jianguo Wang, Purdue University |
Abstract: |
Vector databases have recently emerged as a hot topic due to the widespread interest in LLMs, where vector databases provide the relevant context that enables LLMs to generate more accurate responses. Current vector databases can be broadly categorized into two types: specialized and integrated. Specialized vector databases are explicitly designed for managing vector data, while integrated vector databases support vector search within an existing database system. While specialized vector databases are interesting, there is a significant customer base interested in integrated vector databases for various reasons, such as reluctance to move data out, the desire to link vector embeddings with their source data, and the need for advanced vector search capabilities. However, integrated vector databases face challenges in performance and interoperability. In this talk, I will share our recent experience in building integrated vector databases within two important classes of databases: Relational Databases and Graph Databases. I will show how we address the performance and interoperability challenges, resulting in much more powerful database systems that support advanced RAGs. Next, I will present other challenges in vector databases along with our ongoing work. Finally, I will discuss the broader role of database systems in the era of LLMs and explore how to build future databases that extend beyond vector databases to better support LLMs. |
Bio: |
Jianguo Wang is an Assistant Professor of Computer Science at Purdue University. He obtained his Ph.D. from the University of California, San Diego. He has worked or interned at Zilliz, Amazon AWS, Microsoft Research, Oracle, and Samsung on various database systems. His current research interests include database systems for the cloud and LLMs, especially Disaggregated Databases and Vector Databases. He regularly publishes and serves as a program committee member at premier database conferences such as SIGMOD, VLDB, and ICDE. He also served as a panel moderator for the VLDB'24 panel on vector databases. His research has won multiple awards, including the ACM SIGMOD Research Highlight Award and the NSF CAREER Award. More information can be found at https://cs.purdue.edu/homes/csjgwang/ |
12 May 2025; 10:30
Title: | Where the Database Management System Comes From, and Why it Matters![]() |
Speaker: | Thomas Haigh, University of Wisconsin-Milwaukee |
Abstract: | For more than fifty years the database management system (DBMS) has been the essential foundation information systems of all kinds, from enterprise software to personal websites. Developed to support the integration of different applications and data types for corporate mainframes, the DBMS had technological roots in Cold War defense systems. Thomas Haigh, a leading historian of computing, looks back to the 1960s and 70s for the origins of the DBMS and at related concepts such as the data base administrator, the management information system, and the data warehouse. Today data science is a hot field, and the potential of “data history” is exciting historians of science. Haigh argues that we can’t understand either of those things without recognizing the DBMS as vital infrastructure that mediates and structures interactions between users, applications, and data. |
Bio | Thomas Haigh is a professor and chair of the history department at the University of Wisconsin-Milwaukee. After studying computer science at Manchester University, he won a Fulbright award for a Ph.D. in the history and sociology of science from the University of Pennsylvania. He has researched many topics in the history of computing, from database management systems to internet technologies. Haigh is the lead author of A New History of Modern Computing (2021) and ENIAC in Action (2016), both published by MIT Press. At UWM he runs a retrocomputing lab with working systems from the 1980s and 1990s. His current book project is Artificial Intelligence: The History of a Brand. |
2 June 2025; 10:30 in DC 1304 (Note the unusual room)
Title: | The Limitations of Data, Machine Learning & Us![]() |
Speaker: | Ricardo Baeza-Yates, Northeastern University, Universitat Pompeu Fabra and Universidad de Chile |
Abstract: | Machine learning (ML), particularly deep learning, is being used everywhere. However, not always is used well, ethically and scientifically. In this talk we first do a deep dive in the limitations of supervised ML and data, its key component. We cover small data, datification, bias, predictive optimization issues, evaluating success instead of harm, and pseudoscience, among other problems. The second part is about our own limitations using ML, including different types of human incompetence: cognitive biases, unethical applications, no administrative competence, misinformation, and the impact on mental health. In the final part we discuss regulation on the use of AI and responsible AI principles, that can mitigate the problems outlined above. |
Bio | Ricardo Baeza-Yates is a Visiting Professor in the Khoury College of Computer Sciences at the Silicon Valley campus of Northeastern University as well as part-time professor at the departments of Engineering of Universitat Pompeu Fabra and Computer Science of University of Chile. Before, he was VP of Research at Yahoo Labs, based in Barcelona, Spain, and later in Sunnyvale, California, from 2006 to 2016. He is co-author of the best-seller Modern Information Retrieval textbook published by Addison-Wesley in 1999 and 2011 (2nd ed), that won the ASIST 2012 Book of the Year award. In 2009 he was named ACM Fellow and in 2011 IEEE Fellow. He has won national scientific awards in Chile and Spain, among other accolades and distinctions. He obtained a Ph.D. in CS from the University of Waterloo, Canada, and his areas of expertise are responsible AI, web search and data mining plus data science and algorithms in general. |
9 June 2025; 10:30
Title: | ![]() ![]() |
Speaker: | Jeff Dalton, University of Edinburgh |
Abstract: | |
Bio |
14 July 2025; 10:30
Title: |
Invisible Yet Powerful: Watermarking to Protect Datasets and Models in Machine Learning ![]() |
Speaker: | Lingyang Chu |
Abstract: |
The rapid advancement of AI has transformed both datasets and models into valuable assets, yet they remain vulnerable to unauthorized use, theft, and replication. Watermarking provides a promising solution by embedding verifiable ownership signals to establish ownership protection. Traditional database watermarking techniques assume that attackers seek to preserve query utility, which inherently restricts the extent of modifications they can apply to the data. However, this assumption does not hold for machine learning, where models can maintain predictive performance even when trained on significantly altered datasets. As a result, adversaries can heavily modify a dataset or distill a model while preserving its learning utility, which enables much stronger watermark removal attacks than those in traditional database watermarking. How can we design watermarking methods that safeguard AI-related assets against these threats while maintaining their usability? This talk presents our recent research on addressing the novel challenges in watermarking tabular datasets and deep learning models in the context of machine learning. First, I will introduce TabularMark, a non-blind watermarking framework that embeds verifiable ownership signals into tabular datasets while ensuring that models trained on watermarked data retain high predictive performance. Second, I will discuss blind watermarking for numerical tabular datasets, which enables watermark verification without requiring access to the original data, making it more practical for real-world data-sharing scenarios. Third, I will introduce a robust model watermarking approach that embeds ownership signals into deep neural networks to withstand ensemble distillation attacks. Finally, I will conclude with open challenges and future directions. |
Bio: |
Lingyang Chu is an Assistant Professor in the Department of Computing and Software at McMaster University. He received his Ph.D. in Computer Science from the University of Chinese Academy of Sciences. Before joining McMaster University, he was a postdoctoral fellow at Simon Fraser University and a Principal Researcher at Huawei Technologies Canada. His research focuses on data mining, explainable machine learning, and trustworthy computing, with a growing focus on data security in database systems. Some of his recent works explore AI-related data watermarking techniques to ensure data integrity and provenance in large-scale systems and data markets. He is an Associate Editor of ACM Transactions on Knowledge Discovery from Data (TKDD) and he also served as a program committee member and reviewer for conferences and journals including SIGMOD, VLDB, KDD, ICDE, ICDM, CIKM, CVPR, NeurIPS, ICML, ICLR, ACM Multimedia, TKDE, TMM, etc. |