Better understanding the relationship between ice formation, climate, and lake health
Background
Climate change is a key factor driving changes in the formation and evolution of ice on Canadian lakes during the winter, including the Great Lakes. Changing lake ice has implications far beyond those that immediately come to mind such as being able to skate, ice fish, or navigate ships. The extent, thickness, and duration of ice coverage can also impact physical lake processes, ecological health, shoreline erosion, and water levels.
In the project, Evaluation of Ice Models, researchers investigate the complex process of ice formation on large fresh-water lakes. The relationship between water density, temperature, and pressure – also known as the Equation of State (EOS) – means that fresh water is at its greatest density at a temperature of about 4 degrees Celsius. For lakes situated in mid-to-high latitudes that undergo cooling during the fall, the water on the surface sinks when their density increases. This happens as they lose heat to the atmosphere. This process continues until the lake reaches an almost uniform temperature of 4 degrees. Any further loss of heat at the surface creates lighter (less dense) water which stays near the surface. Ice begins to form on the surface when it reaches zero degrees. In large lakes like Lake Erie, the surface temperature varies across the lake and freezing starts near the shore, where the water is most shallow. Winds complicate the process by pushing ice around the lake but also by mixing the cooler surface water with the warmer and denser water below. When this happens, the surface waters warm up, preventing ice formation. This is why large lakes often do not develop complete ice cover.
Models that simulate ice formation and movement can be helpful in predicting climate change impacts on lakes. For instance, the loss of ice can affect life cycles of aquatic organisms. Without the insulating effect of ice cover, lake temperatures warm which can impact cold water fish species, contribute to fish die-offs, and lead to more algal blooms.
While there are sophisticated ice models for oceans, similar predictive tools are not as available for large lake systems. The Evaluation of Ice Models project explores whetherocean models can be adapted to provide insights into ice formation, ice movement and lake processes for large freshwater lakes. The project focused on Lake Erie.
Researchers simulated the nature of ice cover using two ice models: the MITgcm-SEAICE and ROMS, which was coupled to the Los Alamos Sea Ice Model (CICE). Working at both lake wide and localized scales, they focused on specific processes, such as lake ice dynamics and convection near the ice edge and under ice. They also examined physical processes associated with the mixing of water near the temperature of maximum density.
Findings
- Using the MITgcm-SEAICE model, researchers were able to simulate how winds blowing along an ice edge affect ice growth and convection.
- The MITgcm-SEAICE model was unable to reproduce important behaviours such as the rapid freeze-up in mid-December that began in the central basin of Lake Erie in the 2013-2014 season. More work needs to be done to make this a useful model for studying ice formation on the Great Lakes.
- ROMS was coupled to CICE. The simulations that were done for the 2013-2014 winter season showed positive results, however the model predicted higher ice concentrations in much of the central and eastern basins.
- Predicting lake ice formation in Lake Erie requires a greater understanding of what happens when a river meets the lake during the winter months and how water mixes when it is near the temperature of maximum density (i.e., 4 degrees Celsius). Using high resolution numerical simulations and two-and three-dimensional model simulations, we found:
- Water temperature, gravity current, and surrounding ambient water conditions impact the mixing of river and lake water, as well as how far the river plume extends into the lake. Importantly, it also affects whether the river water stays near the surface or sinks.
- A lake’s surface currents – including river plumes and under ice current – during the winter are influenced by shear and how heat from the sun is absorbed into the water column.
- Cabbeling of gravity currents near the temperature of maximum density has significant implications for vertical mixing and where the water and nutrients that enter a cold lake end up.
This project provides the scientific grounding for future research into the relationship between winter phytoplankton blooms and harmful algal blooms that occur late summer.
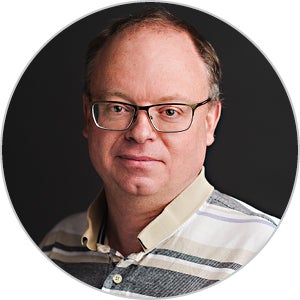
Principal Investigator:
Kevin Lamb, Professor, Applied Mathematics
Co-investigators from UW:
Andrea Scott, Marek Stastna
Project duration:
2017-2020
GWF funding support:
$170,000
Key messages for ice modellers
- Current hydrodynamic-ice models need considerable improvement before they can accurately model the formation and evolution of ice on large lakes that are only partially ice-covered. Improved remote sensing products are needed to assess model outputs.
- Mixing of waters near the temperature of maximum temperature has many subtle aspects. Better parametrizations of this are needed for use in lake scale models.
Key publications
Grace, A. P., Stastna, M., Lamb, K. G., & Scott, K. A. (2021). Asymmetries in gravity currents attributed to the nonlinear equation of state. J. Fluid Mech., 915, A18. https://doi.org/10.1017/jfm.2021.87
Grace, A. P., Stastna, M., Lamb, K. G., & Scott, K. A. (2022). Numerical simulations of the three-dimensionalization of a shear flow in radiatively forced cold water below the density maximum. Physical Review Fluids, 7(2). https://doi.org/10.1103/PhysRevFluids.7.023501
Grace, A. P., Stastna, M., Lamb, K. G., & Scott, K. A. (2023). Gravity currents in the cabbeling regime. Physical Review Fluids, 8(1). https://doi.org/10.1103/PhysRevFluids.8.014502
Scott, K. A., Xu, L., & Kheyrollah-Pour, H. (2020). Retrieval of ice/water observations from synthetic aperture radar imagery for use in lake ice data assimilation. Journal of Great Lakes Research, 46, 1521-1532. https://doi.org/10.1016/j.jglr.2020.08.018
Search the complete list of GWF publications.
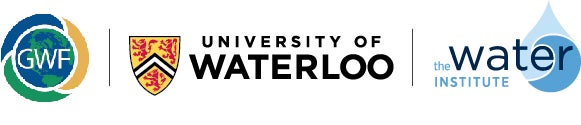