Ziang Zhu, Wayne Parker
Department of Civil and Environmental Engineering, University of Waterloo
Alexander Wong
Department of Systems Design Engineering & Waterloo Artificial Intelligence Institute, University of Waterloo
The widespread distribution and abundance of microplastics (MPs) has led to concerns about the impacts of this form of pollution on the environment. To better understand how MPs may be impacting the environment, we need to further develop the methods that we can use for the accurate identification of MPs. This is an important step supporting environmental assessment via tracing the source of plastic pollution and understanding the fate and transport of MPs in the environment.
Spectroscopic methods such as FTIR spectroscopy are commonly used for the identification of MPs in environmental samples. The spectrum of a particle is analogous to a fingerprint of a person, that can be used to determine its makeup. While the analysis of the spectra can be complex due to a variety of factors that cause the spectra of MPs to deviate substantially from the spectra of pure plastics, we have developed a deep learning technology that has shown promising results that could help standardize MP characterization.
There can be significant variations in spectra that are collected from MPs with the same base polymer. First, the composition of microplastics can vary depending on the presence of additives such as fillers, plasticizers, antioxidants, UV stabilizers, lubricants, dyes, and flame-retardants. Second, the surfaces of plastics can be modified when exposed to factors such as ozone, temperature, light radiation, mold and bacterial interaction. Third, biofilms can attach to MPs, and chemicals can adsorb to the surfaces of MPs, causing additional impurities. For these reasons, the spectra of MPs derived from environmental samples can differ substantially from their base polymers. This can make it difficult to accurately identify plastic types.
Conventional approaches for MP identification rely on matching the spectra of samples to the spectra of pure polymers obtained from databases. Due to the significant variation of spectra caused by the aforementioned conditions, the conventional method can result in substantial recognition errors.
Given the recent advances in the field of deep learning, there is a growing interest in leveraging Convolution Neural Networks (CNN) that contain a series of convolution layers and pooling layers for the recognition of MP spectra. CNNs have the ability to accurately recognize unknown entities after being trained with a known data set. The more diversity in the data used to train the model, the more intelligent the model becomes in terms of accounting for variations in the data to be recognized. Rather than comparing with a limited set of reference spectra, as with the conventional spectra matching methods, CNNs can be trained using numerous spectra of both pure polymers and polymers with impurities (10,000+ if data available). Hence, the CNN can learn discriminative spectral features thereby characterizing each plastic type in a way that accounts for inherent spectral variances.
Motivated by this, we constructed a tailored CNN (PlasticNet) for the purpose of identifying MP composition in environmental samples. The spectra of both virgin and non-virgin were employed in the training of PlasticNet to enhance recognition of a variety of spectra.
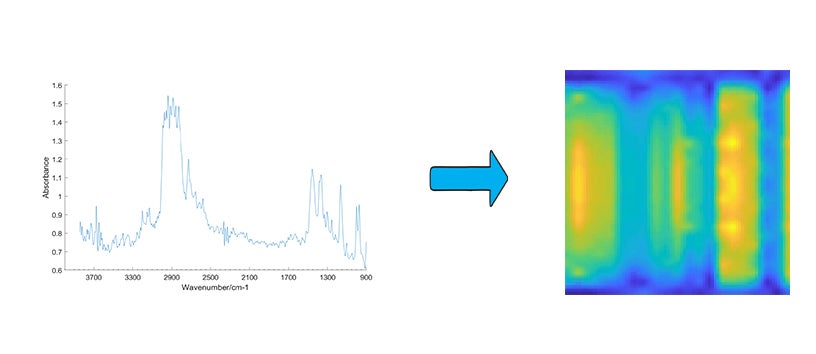
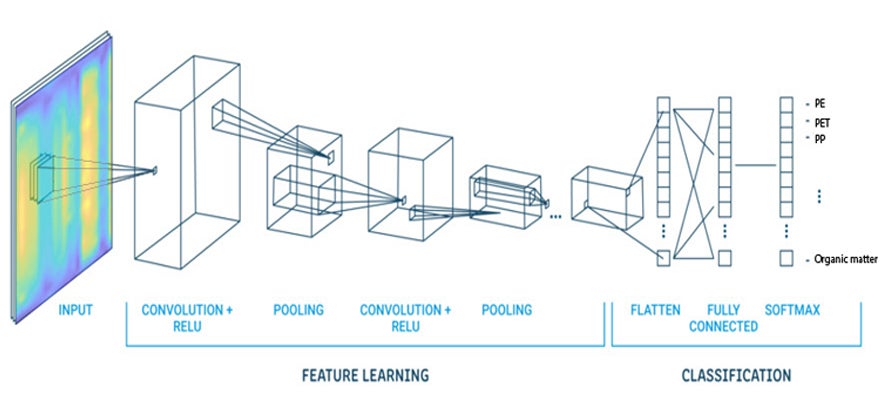
Converting a spectrum to an image and recognizing it using convolution neural network. The convolution layers and pooling layers in CNN are used for the learning of features, while the fully connected layers and the softmax layer are used for the classification.
The spectra of the MPs were collected using a focal plane array based (FPA-based) micro-Fourier transform infrared spectroscope. The instrument simultaneously collects multiple spectra of MPs on a surface; hence hundreds of spectra were obtained from each particle for training purposes.
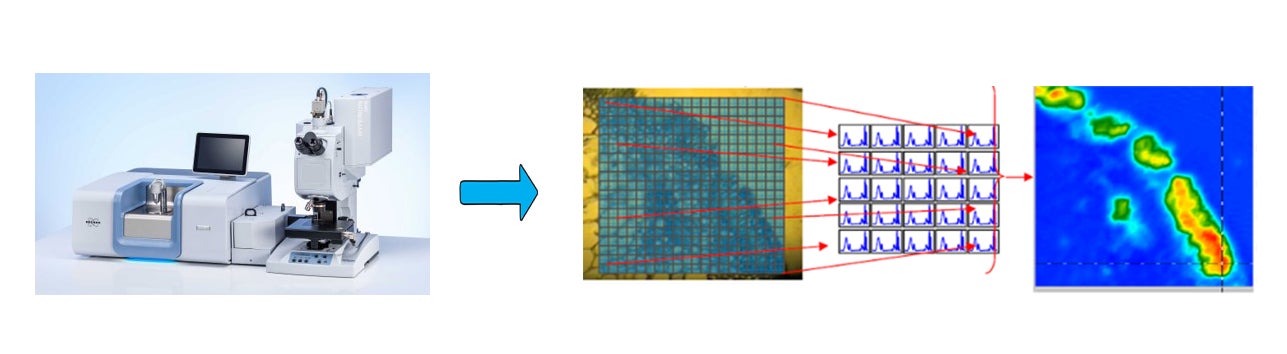
FPA-based micro-Fourier transform infrared spectroscope collecting multiple spectra on a surface
We tested the capability of PlasticNet to recognize spectra with variation by using a trained version to assess the range of spectra that were not in the training dataset. We tested MPs that were composed of 5 types of Polypropylene (PP) and 6 types of Polyethylene (PE) that had either experienced weathering or contained additives including UV stabilizer, anti-oxidants, process-scavengers, fire retardants and dyes. These spectra had additional peaks compared with the spectra of pure PP and PE MPs. Despite this, PlasticNet achieved 85%+ success in recognizing MPs in the test dataset thereby demonstrating that deep learning can resolve spectral variation in spite of the presence of additional peaks. Hence, PlasticNet has the potential to become a standard method for the recognition of MPs that are analyzed by spectroscopy.
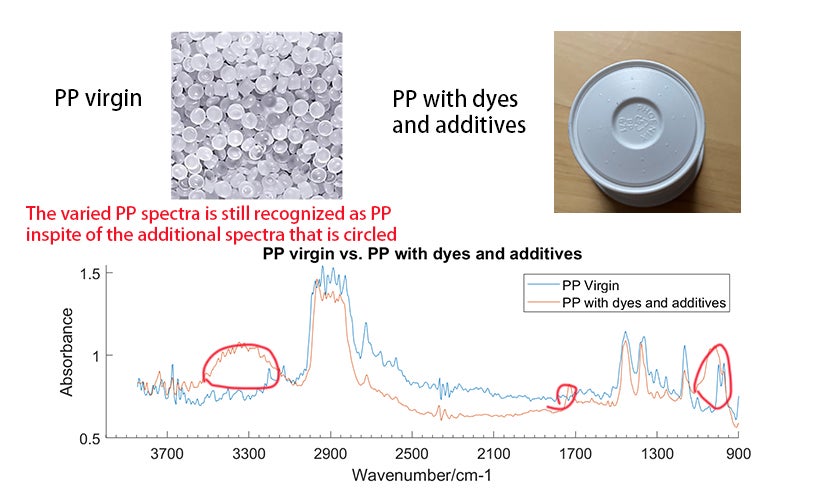
Images and spectra of virgin PP vs. PP with dyes and additives. The varied PP spectra is still recognized as PP