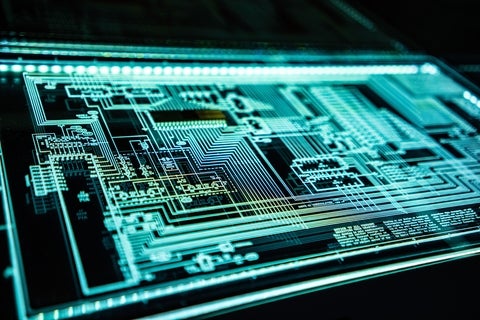
Team develops new probabilistic model to improve estimates of microplastic concentration
By: Frank Zhu, Wayne Parker, Philip Schmidt, Department of Civil and Environmental Engineering, University of Waterloo
The Microplastics (MPs) Fingerprinting project aims to understand the source, transport, and fate of microplastics at a watershed scale in order to develop risk mitigation strategies. But before we can understand the impact these contaminants have on our ecosystems, we need a methodological approach that can accurately determine the concentration of microplastics in the environment. With this in mind, our research team brought samples from wastewater influent, wastewater sludge, and wastewater effluent to the lab where we worked on perfecting the process for quantifying the number of microplastic particles present in each sample.
It may sound like a simple task, but it is quite challenging due to many uncertainties associated with the enumeration process. First, while attempting to quantify particles in a replicates sample, we kept coming up with different numbers even though we used the same sampling point. This could be because we had to extract the microplastics from the sample before we could begin counting the particles present. This meant that our recovery method was only able to measure the percentage of particles that “survived” the extraction process. Second, we lost a certain number of particles during the extraction process because microplastics tend to stick to the glass when the sample is transferred between vessels. Third, the recovery of particles is not constant when we perform replicates tests. Finally, we discovered that the recovery of microplastics was affected by their size and shape. For example, smaller particles were more likely to get lost in the process by sticking to the glassware, than larger particles.
To reduce uncertainty and improve accuracy of the counting process, we developed a model that helps us identify where things are most likely to go wrong so that we can avoid those pitfalls. The model is based on a number of statistical assumptions (e.g., we assumed that the random sampling error is Poisson distributed, the loss of MPs is binomially distributed, and that non-constant analytical recovery is beta distributed). These assumptions were informed by what we observed in the lab regarding microplastic behavior, as well as literature on other enumeration models (Schmidt et al., 2010), and relevant statistic theories. We also grouped the microplastic particles into four categories based on their shape and size because they have different properties that can impact recovery during extraction.
We tested out our model and found that it can be very helpful in reducing uncertainty and improving the accuracy of the enumeration process, when the following practices are observed:
- Each sample collection should include at least 3 replicates to reduce uncertainties
- The counting results should always be corrected to account for the imperfect recovery of microplastics
- The particles should be separated into different categories base on the size and shape before counting (here we suggest 4 categories), and different recovery parameters for each category should be considered
In the end, our team hopes that our model will help other microplastic researchers better interpret their data correctly and rigorously. This work can also contribute to the eventual establishment of a standardized approach to analyzing microplastics in wastewater and sludge samples. If you are interested in learning more about how we tested our statistical model or our analysis, please contact Frank Zhu.
References
Schmidt, P. J., Emelko, M. B., & Reilly, P. M. J. E. s. (2010). Quantification of analytical recovery in particle and microorganism enumeration methods. Environmental science technology, 44(5), 1705-1712.
Photo by Adi Goldstein on Unsplash