Have you seen the new paper published by Drs. Almosnino & Cappelletto, Human Factors Ergonomics (HFE) research scientists at Amazon? The article, published in IISE Transactions on Occupational Ergonomics and Human Factors, combines digital human modeling and optimization (using excel!) to inform job design. Our OBEL team recently reviewed this paper in our Journal Club, and we wanted to share a summary for you here.
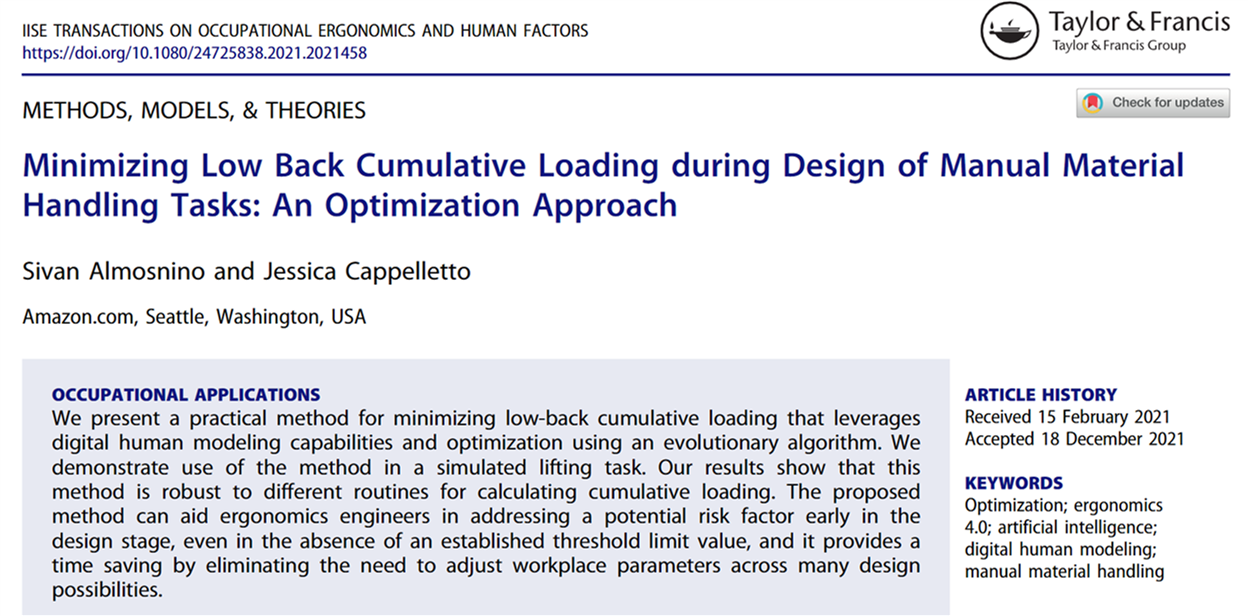
First, let’s make sure we’re all on the same page regarding some key terms. A Digital Human Model, or DHM for short, is simply a representation of a human on a computer screen. Several DHM software packages exist allowing users to simulate human behaviours, and in turn, estimate HFE hazard exposure metrics like peak low back compressive load, or in the case of the paper, cumulative low back compressive load.
Optimization, on the other hand, is a mathematical procedure for finding the design variable(s) that minimize or maximize an objective function, subject to constraints. What exactly does that mean? Well, basically we’re trying to figure out the best solution (“how should I place all of these boxes onto those shelves”), using some type of decision criteria (“I want to minimize low back cumulative load), subject to limitations (“each shelf can only hold a maximum of 16 boxes”).
So, why exactly might we care about this paper. We offer a few perspectives:
- Amazon is quickly growing a compliment of some of the best and brightest HFE experts from around the globe. This paper could be an early sign of things to watch out for. Is Amazon going to fill a HFE research void that was left when Liberty Mutual closed its laboratory?
- This paper is a really nice example of how two tools (digital human model) and Microsoft excel (solver add-in) can be used to advance MSD prevention efforts.
So let’s dive in. The main aim of the Almosnino & Cappelletto (2022) paper was to demonstrate how the use of a DHM and optimization could be combined to determine how to best allocate boxes into a shelving unit in a way the minimized the low back cumulative load on the worker. Using the DHM software, Jack™, Almosnino & Cappelletto (2022) simulated lifts from an origin location to each possible destination location within a mock shelving unit.
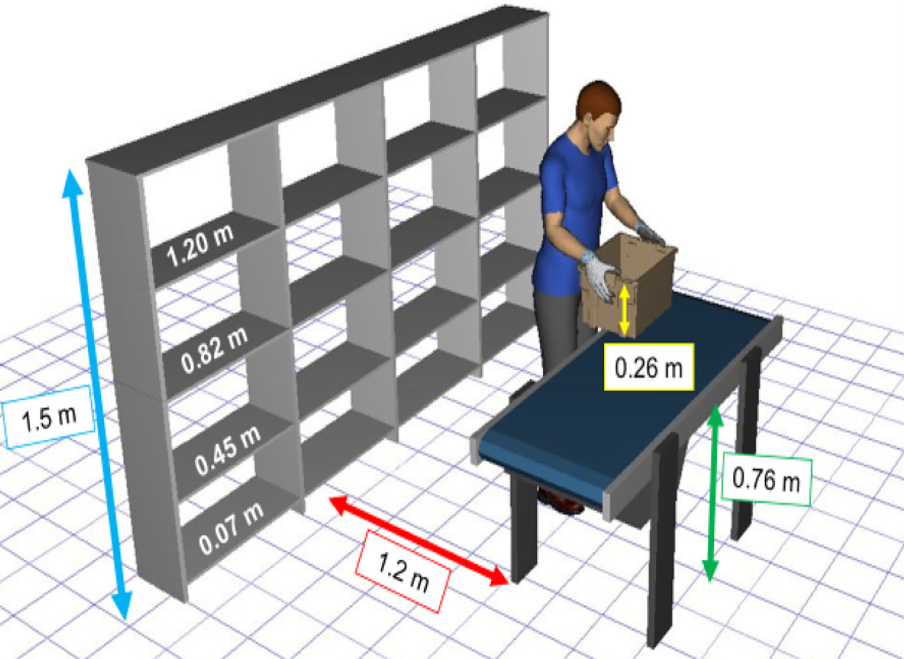
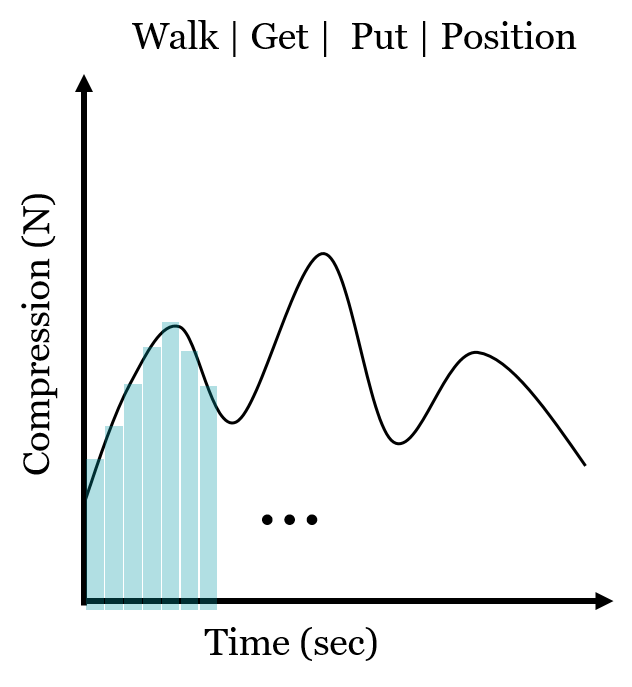
Simulations were completed using a 50th percentile male avatar and 50th percentile stature female avatar. The simulations were also repeated with four different box masses (2, 5, 10, and 15kg). These simulations were then used to determine the corresponding spine compression (for each lift, of each box, by each avatar), where the corresponding spine compression time series was integrated as an estimate of cumulative spine compression (see example diagram), which the authors termed low back cumulative load (LBCL). As a secondary aim, Almosnino & Cappelletto (2022) also explored different methods for calculating the LBCL metric, but we encourage interested readers to read the paper in detail for more insight on that analysis.
Once the simulations were complete, Almosnino & Cappelletto (2022) knew the LBCL “cost” to move each box to each shelf location. These data were then imported into Microsoft Excel and organized such that the Solver add-in could be used to find the optimal number of each box (by mass) to place on each shelf in order to minimize the LBCL “cost”. However, they also describe some specific constraints, or limitations to consider including:
- A total of 250 boxes must be placed in the shelf
- Only 16 boxes can be placed in any one shelf location
- They ran the optimization for specific proportions of boxes (e.g., Run 1 required that 80% were 2kg, 10% were 5kg, … etc.)
- A shelf couldn’t have negative boxes (“the non-negativity constraint”)
- A shelf couldn’t have a partial box (“the integer constraint”)
This short video demonstrates how Microsoft Excel can be applied to set up and run the optimization analysis, similar to Almosnino and Cappelletto (2022).
Now, like any study, the work of Almosnino & Cappelletto (2022) is not without limitations. For example, the results may be different if the goal was changed to, say, minimize peak shoulder torques. So it is important to keep in mind that the outcome of an optimization like this depends very much on the goal, or “cost”, or objective function to optimize. Second, like any model-based approach, several simplifications and assumptions are usually required. For example, cumulative load depends on both the magnitude of the compressive force, but also the duration of exposure. We know that some folks may move a little faster, and other a little slower. However, for this example, movement times based on Movement Time Methods data built into Jack™ were used as an approximation. As a result, it’s important to remember that an approach like this provides some guidance to inform decision making, but may not consider all of the natural variability that might occur within the workplace.
After an excellent Journal Club conversation, the OBEL team agreed that this paper represents a new and interesting way to combine DHM and optimization to inform MSD prevention. While more research and development is likely ahead to evolve the approach, if you’re interested in emerging trends in MSD prevention, this paper is a must read!