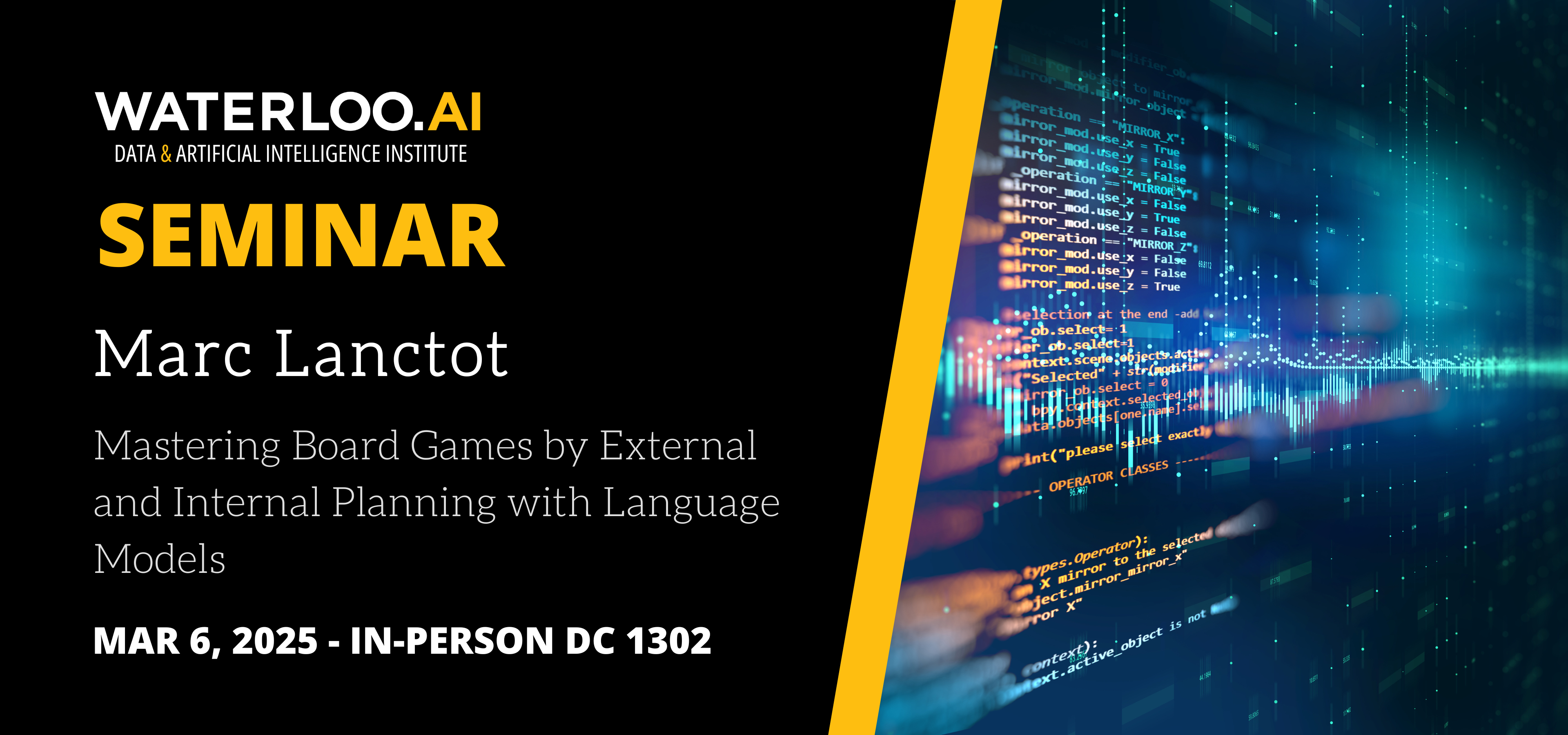
Date: Thursday, March 6, 2025
Time: 1:00 pm - 2:00 pm
Location: DC 1302
Title: Mastering Board Games by External and Internal Planning with Language Models
This seminar explores how AI utilizes language models for strategic planning in board games. It delves into the integration of external and internal planning mechanisms, where external planning involves leveraging pre-trained models to predict optimal moves, while internal planning refines decision-making through self-play and game-state evaluation. The talk will highlight how these approaches enable AI to understand, adapt, and optimize strategies in complex, decision-based environments. Attendees will gain insights into the latest advancements in AI-driven gameplay, reinforcement learning, and multi-agent planning, with real-world applications extending beyond board games.
Bio: Marc Lanctot is a research scientist at DeepMind. His research interests include multiagent reinforcement learning, computational game theory, multiagent systems, and game-tree search. In the past few years, Marc has investigated general agent evaluation based on computational social choice and game-theoretic approaches to multiagent reinforcement learning with applications to fully and partially observable games.
Marc received a Ph.D. degree in artificial intelligence from the Department of Computer Science, University of Alberta in 2013. Before joining DeepMind, Marc completed a Postdoctoral Research Fellowship at the Department of Knowledge Engineering, Maastricht University, in Maastricht, The Netherlands, on Monte Carlo tree search methods in games.