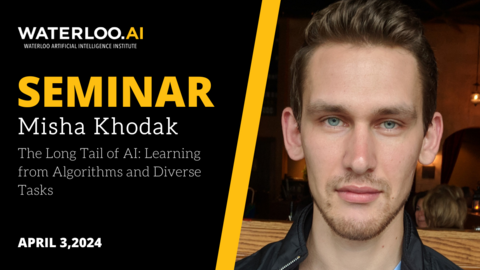
Speaker: Misha Khodak
Date: Wednesday, April 3rd, 2024
Time: 10:30 AM - 11:30 AM
Location: DC 1304
Title: The Long Tail of AI: Learning from Algorithms and Diverse Tasks
Abstract: Advances in machine learning (ML) have led to skyrocketing demand across diverse applications beyond vision and text, resulting in unique theoretical and practical challenges. The vastness of use cases calls for general-purpose yet customizable tools for tackling large subclasses of such problems.
In this talk, I will introduce an algorithm design framework for learning from algorithmic data, in which the goal is to develop and analyze “meta-algorithms” that improve the performance of other algorithms using datasets of related instances. Our approach yields the first guarantees for meta-learning to initialize gradient descent and a systematic way to answer crucial questions in the burgeoning field of algorithms with predictions, such as “where do the predictions come from?” In practice, this theory leads to an effective solution for the challenging problem of federated hyperparameter tuning and to the attainment of near-instance-optimal solver performance across sequences of linear systems. I will conclude with ongoing and future work on integrating learning to accelerate scientific computing and automating the application of the latest breakthroughs in AI to diverse data modalities.
Speaker Bio: Misha Khodak is a PhD student in computer science at Carnegie Mellon University. He studies foundations and applications of machine learning, especially learning across multiple tasks, incorporating data into algorithm design, and transferring modern AI tools across diverse data modalities.
Misha is a recipient of the Facebook PhD Fellowship and CMU’s TCS Presidential Fellowship, has been named a rising star in data science and in ML & systems, and has interned at Microsoft Research, Google Research, and the Lawrence Livermore National Lab.