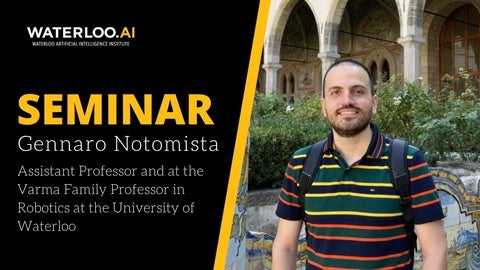
Title: Learning to Execute Prioritized Stacks of Robotic Tasks
Abstract: Learning complex robotic tasks can be challenging for several reasons. The nature of compound tasks, made up of several simpler subtasks, renders it difficult to simultaneously capture and combine all features of the subtasks to be learned. Therefore, whenever there is an effective way of combining the execution of multiple subtasks, it is useful to break down complex tasks into building blocks that can be independently learned in a more efficient fashion. Besides the reduced computational complexity stemming from the simpler nature of the subtasks to be learned, this approach has the benefit of increasing the modularity of the task execution framework, by allowing for a reuse of the subtasks as building blocks for the execution of different complex tasks. In this talk I will present a constrained-optimization formulation for the prioritized execution of multiple learned robot tasks, which lends itself to the execution of tasks encoded by value functions, such as tasks learned using the reinforcement learning paradigm. The key idea is to encode tasks as constraints of a convex optimization program, where an additional time-varying constraint can be included to specify dynamic stacks of prioritized tasks. The proposed control framework is showcased using a team of mobile robots performing long-term environmental monitoring.
Speaker Bio: Gennaro Notomista is an Assistant Professor and the Varma Family Professor in Robotics in the Department of Electrical and Computer Engineering at the University of Waterloo (Waterloo, ON, Canada). Prior to joining University of Waterloo, he was a post-doctoral researcher at the CNRS/Inria/IRISA, Rennes, France. He received the Ph.D. degree in robotics from the Georgia Institute of Technology (Atlanta, GA, USA) in 2020. Dr. Notomista is a Fulbright Scholar and was the recipient of the Alumni Small Grant (2020) and the IEEE ARSO Best Paper Award (2022). His main research interests lie at the intersection of design and control of robotic systems for long-duration autonomy with applications to environmental monitoring.
Date: Tuesday, June 6th, 2023
Time: 1:00 PM - 2:00 PM EST
Location: Davis Centre (DC) 1302
This seminar will be hybrid.