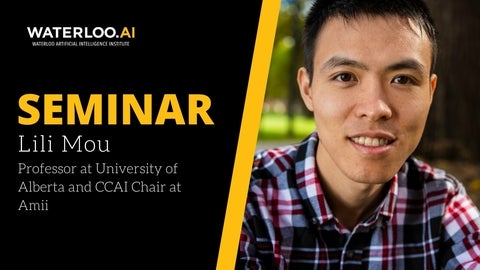
Title: Modeling Uncertainty in Text Generation
Abstract: Text generation is the task of writing meaningful and coherent text, covering various natural language generation applications such as summarization and machine translation. One of the major challenges in text generation is uncertainty, as natural language utterances are highly unpredictable. For example, a dialogue model may have multiple plausible responses when asked about its hobby, which makes training difficult as the model tries to learn all the different ways to respond. In this talk, Lili will go over two recent studies of his group that addresses this problem. His first work focuses on using a mixture model for diverse dialogue generation, where he proposes a novel EM algorithm, allowing different mixture components to capture distinct dialogue patterns. The second work addresses uncertainty in the sequence-level knowledge distillation setting, where he proposes FDISTILL, a novel framework that formulates sequential knowledge distillation as minimizing a generalized f-divergence function. Both studies highlight the importance of tackling uncertainty in text generation.
Speaker Bio: Dr. Lili Mou is an Assistant Professor at the Department of Computing Science, University of Alberta. He is also an Alberta Machine Intelligence Institute (Amii) Fellow and a Canada CIFAR AI (CCAI) Chair. Lili received his BS and PhD degrees in 2012 and 2017, respectively, from School of EECS, Peking University. After that, he worked as a postdoctoral fellow at the University of Waterloo. His research interests include deep learning applied to natural language processing as well as programming language processing. He has publications at top conferences and journals, including AAAI, EMNLP, TACL, ICML, ICLR, and NeurIPS. He also presented tutorials at EMNLP'19 and ACL'20. He received a AAAI New Faculty Highlight Award in 2021.
Date: Friday, July 7th, 2023
Time: 11:00 AM - 12:00 PM EST
Location: Faculty Hall (E7-7303 & 7363)
This seminar will be hybrid.