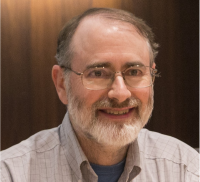
Speaker: Dr. Dietterich, Professor - Oregon State University
Abstract: Consider a user who must decide whether to execute a policy in a Markov Decision Process. Prior to pressing "Go", the user may wish to have a prospective guarantee on how the policy will behave starting in the current state and executing for H steps (the horizon). In this talk, I will show how to extend the methods of conformal prediction to produce a multi-dimensional confidence region that will contain the future trajectory of the policy with probability 1 - delta. I'll illustrate the method with examples from Starcraft games and from ecosystem management problems. This is part of a larger effort to increase the robustness and predictability of AI systems.
Bio: Dr. Dietterich (AB Oberlin College 1977; MS University of Illinois 1979; PhD Stanford University 1984) is Distinguished Professor Emeritus in the School of Electrical Engineering and Computer Science at Oregon State University. Dietterich is one of the pioneers of the field of Machine Learning and has authored more than 200 refereed publications and two books. His current research topics include robust artificial intelligence, robust human-AI systems, and applications in sustainability.
Dietterich has devoted many years of service to the research community. He is a former President of the Association for the Advancement of Artificial Intelligence, and the founding president of the International Machine Learning Society. Other major roles include Executive Editor of the journal Machine Learning, co-founder of the Journal for Machine Learning Research, and program chair of AAAI 1990 and NIPS 2000. He currently serves as one of the moderators for the cs.LG category on arXiv.
Seminar Recording: https://www.youtube.com/watch?v=0UKLcBSpTR4