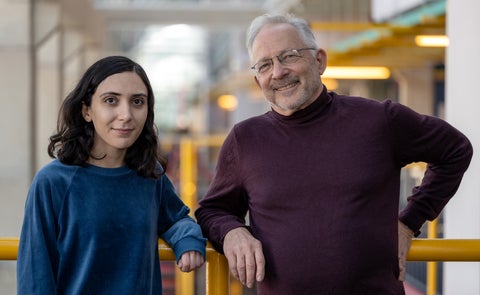
Niki Hasrati and Shai Ben-David receive best paper award at ALT 2023
By: Cheriton School of Computer Science
Master’s candidate Niki Hasrati and Professor Shai Ben-David have received the best paper award at ALT 2023, the 34th International Conference on Algorithmic Learning Theory. This year, the annual meeting that explores the theoretical and algorithmic aspects of machine learning will take place in Singapore from February 20 to 23.
Their paper, “On Computable Online Learning,” studies online learning with computable learners. It analyzes that question under various requirements for successful learning. The paper gives a necessary and sufficient condition for optimal computable online learning and shows that the Littlestone dimension — a combinatorial parameter that characterizes online learnability without computability requirements — no longer applies when the learners are required to be computable.
Niki Hasrati is a master’s student at the Cheriton School of Computer Science, advised by Professor Shai Ben-David. Her research interests intersect theoretical computer science and theoretical machine learning. She studies online learning, private learning, combinatorial characterizations of different learning models, and non-worst-case analysis of computational and statistical resources required for learning.
Shai Ben-David is a University Research Chair and Professor at the Cheriton School of Computer Science. He is also a faculty member at the Vector Institute and a Canada CIFAR Artificial Intelligence Chair. His research spans a wide spectrum of topics in the foundations of computer science and its applications, with a particular emphasis on statistical and computational machine learning. The common thread throughout his research is to provide mathematical formulation and understanding of real-world problems.
About this award-winning research
Two central setups for machine learning are batch learning, where the learner is given a set or batch of randomly generated training examples, and online learning, where the learner encounters examples one at a time and gets feedback on its performance on each example before being challenged with the next one.
A fundamental question in each of these setups is distinguishing between learnable and unlearnable tasks. Learnability in the batch setup is known to be characterized by the Vapnik–Chervonenkis dimension (see Vapnik and Chervonenkis, 1971) and learnability in the online setup is characterized by the Littlestone dimension (see Littlestone, 1988).
In both cases, however, learnability is defined in terms of the existence of a successful learning function — for example, a mapping of training examples to learned models. In their ALT 2020 paper, Agarwal, Ananthakrishnan, Lechner, Ben-David and Urner pointed out that in practical machine learning, one cares only about learners that can be realized by computer programs, what are known as computable learners. They showed that once one considers only such learners, the Vapnik–Chervonenkis dimension no longer characterizes learnability in the batch setup.
Niki and Shai’s 2023 award-winning paper addresses the question of computable learnability in the online learning setup. The paper analyzes various notions of success, or optimality, of an online learner when learners are required to be computable — that is, when they are executable by an algorithm. Their paper shows that, in fact, the well-known characterization of online learnability by the Littlestone dimension breaks down in the context of computable learning. The answer required the use of sophisticated recursion theoretic analysis, as well as careful examination of different tasks that an online learner may be faced with.
To learn more about the research that resulted in Niki and Shai receiving the best paper award at ALT 2023, please see Niki Hasrati, Shai Ben-David. On Computable Online Learning. 34th International Conference on Algorithmic Learning Theory (ALT), 2023.