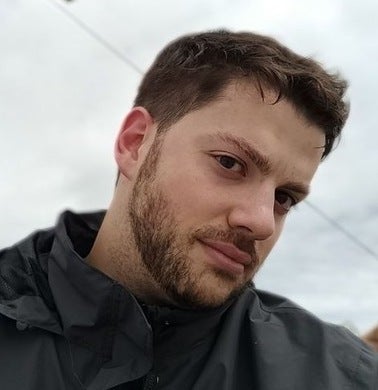
Title: Fast and differentiable emulation of cosmological observable
Abstract: Forthcoming LSS and CMB surveys will provide us with an unprecedented amount of data, that will shape our knowledge of the Universe. However, the analysis of these datasets poses a serious computational challenge, pushing standard methods to their limits. In order to face this kind of problem, several codes known as emulator have been developed by the cosmological community. An emulator is an approximate method that mimics the behavior of a computationally expensive function. Although creating an emulator is conceptually straightforward, there are several design choices (type and architecture of the surrogate model, preprocessing procedure, dimensional reduction technique, ...) which can have a dramatic impact on the final performance. In this talk I will present one of these applications, Capse.jl, a novel emulator that utilizes neural networks to predict Cosmic Microwave Background (CMB) temperature, polarization and lensing angular power spectra, which uses different preprocessing and dimensional-reduction techniques to similar works in the literature. The emulator computes predictions in just few microseconds with emulation errors below 0.1 sigma for all the scales relevant for the planned CMB-S4 survey. Capse.jl can also be trained in an hour's time on a CPU. As a test case, we use Capse.jl to analyze Planck 2018 data and ACT DR4 data. We obtain the same result as standard analysis methods with a computational efficiency 3 to 6 order of magnitude higher. We take advantage of the differentiability of our emulators to use gradients-based methods, such as Pathfinder, Hamiltonian Monte Carlo (HMC), and MicroCanonical Hamiltonian MonteCarlo (MCHMC), which speed up the convergence and increase sampling efficiency. Finally, I'll give an overview of similar ongoing applications in the cosmological context and how I am working to extend the developed framework.