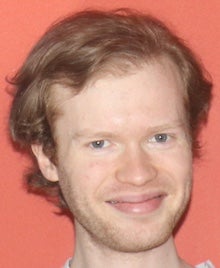
Harry Desmond is a Senior Research Fellow at the Institute of Cosmology and Gravitation, University of Portsmouth. He completed his PhD at Stanford in 2017 and then held postdoctoral fellowships at Oxford and CMU. His primary interest is testing fundamental physics (mainly modified gravity and dark matter) using astrophysical objects (mainly galaxies and stars); he also works on galaxy formation and dynamics, cosmological simulations, astrostatistics and machine learning.
Title: Exhaustive Symbolic Regression: Learning Astrophysics directly from Data
Abstract: Machine learning is the new frontier in data-driven science. A key challenge is to make machine-assisted discovery interpretable, enabling it not only to uncover correlations but enhance our physical understanding of the world. A nascent branch of machine learning -- Symbolic Regression (SR) -- aims to discover the optimal functional representations of datasets, producing perfectly interpretable outputs (equations) by construction. After summarising the traditional SR method of stochastically generating trial functions through an analogue of natural selection ("genetic programming"), I will describe my more ambitious approach of searching and evaluating function space exhaustively. Coupled to an information-theoretic model selection principle based on minimum description length, Exhaustive Symbolic Regression is guaranteed to find the simple functions that optimally balance accuracy with simplicity on a dataset. This gives it broad application across science. I will describe the method and use it to quantify the extent to which state-of-the-art astrophysical theories -- FLRW cosmology, General Relativity and Inflation -- are implied by the current data.