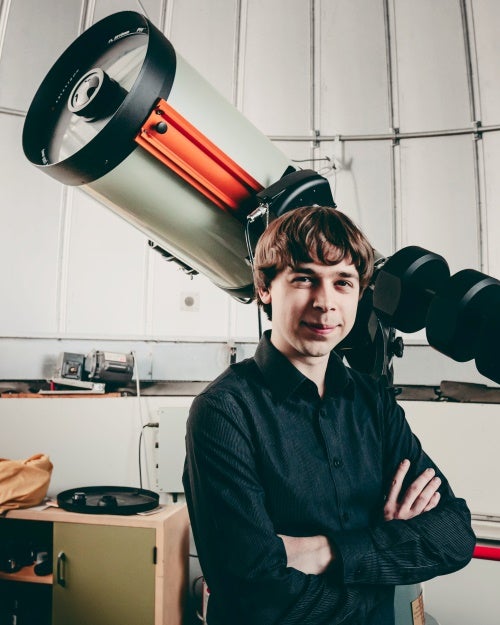
Title: AstroPhot: Fitting Everything Everywhere All at Once in Astronomical Images
Abstract: The latest generation of telescopes is producing multi-band and multi-epoch data at an unprecedented scale of quantity and quality. However, the pixels in these images are not of-themselves very informative; only when models are applied to these images do insights emerge. For this purpose we have developed AstroPhot, a python-based, GPU-accelerated, differentiable, object-oriented tool for fitting astronomical images. Everything: AstroPhot can fit models for sky, stars, galaxies, PSFs, and more in a principled chi^2 forward optimization, recovering Bayesian posterior information and covariance of all parameters. Everywhere: AstroPhot can optimize forward models on CPU or GPU; across images that are large, multi-band, multi-epoch, rotated, dithered, and more. All at once: The models are optimized together, thus handling overlapping objects and including the covariance between parameters. I will discuss how these capabilities compare with other PSF and galaxy fitting techniques. Finally, I will discuss how differentiable codes (like AstroPhot, though extending to machine learning) can enable new avenues for principled Bayesian analysis of complex data.