Conventional and Emerging CO2 Capture and Conversion Technologies
An integrated real-time optimization, control, and estimation scheme for post-combustion CO2 capture (Patrón and Ricardez-Sandoval, Applied Energy, 2022).
This study presents a novel operational scheme for post-combustion CO2 capture (PCC) plants downstream from fuel-fired power plants. The approach is comprised of real-time optimization (RTO), nonlinear model predictive control (NMPC), and moving horizon estimation (MHE) layers. These layers are integrated to operate the system economically via a new economic function that accounts for the most significant economic aspects of PCC, including the carbon economy, energy, chemical, and utility costs. The proposed approach was employed on the case study of an MEA-based PCC absorber section, which uses a mechanistic process model to provide an accurate representation of the system. The NMPC layer is novel in its ability to enable flexible control of the plant by manipulating fresh material streams to impact CO2 capture and the MHE layer is the first to provide accurate system estimates to the controller with realiztically accessible measurements. The proposed scheme was subjected to cofiring and dirunal load variation scenarios. Both scenarios displayed steady-state cost savings that exceeded the energy penalty imposed on the power plant by the PCC plant. This suggests the proposed scheme is an effective framework for the economic operation of a general class of PCC plants (i.e., with different solvents, process designs and control schemes, etc.) and can help enable the viability of PCC for the continued use of fuel-firing.
The full paper can be found here.
Dynamic Modeling of Recirculating Aquaculture Systems: Effect of Management Strategies and Water Quality Parameters on Fish Performance (Kamali, Ward, & Ricardez-Sandoval, Aquacultural Engineering, 2022).
The full paper can be found here.
Computer-Aided Design of Materials
A Semi-Empirical Force Balance-Based Model to Capture Sessile Droplet Spread on Smooth Surfaces: A Moving Front Kinetic Monte Carlo Study
The full paper can be found here.
Catalyst Screening for Reaction Networks using Machine Learning
Often, catalyst screening in reaction networks requires hundreds or thousands of density functional theory (DFT) simulations, or demanding laboratory experimental period, thus making the exclusive use of these tools expensive and time consuming. Machine learning (ML) tools can dramatically expedite the predictive and filtering process, thereby significantly speeding up the discovery of attractive materials. The aim of this study is to develop metal doped CeO₂ catalysts for CO₂ hydrogenation via reverse water gas shift reaction using DFT, machine learning and laboratory experiments, as shown in Figure 1 below. A 3D-Graphomer model was adopted and modified to screen for potential doping materials. To overcome the challenge of limited data and the absence of cerium in the accessible data, modifications of the vector representations of the elements were performed and enabled predictions with higher accuracy. By refining the model, effective screening for potential catalysts and other materials is possible and promising.
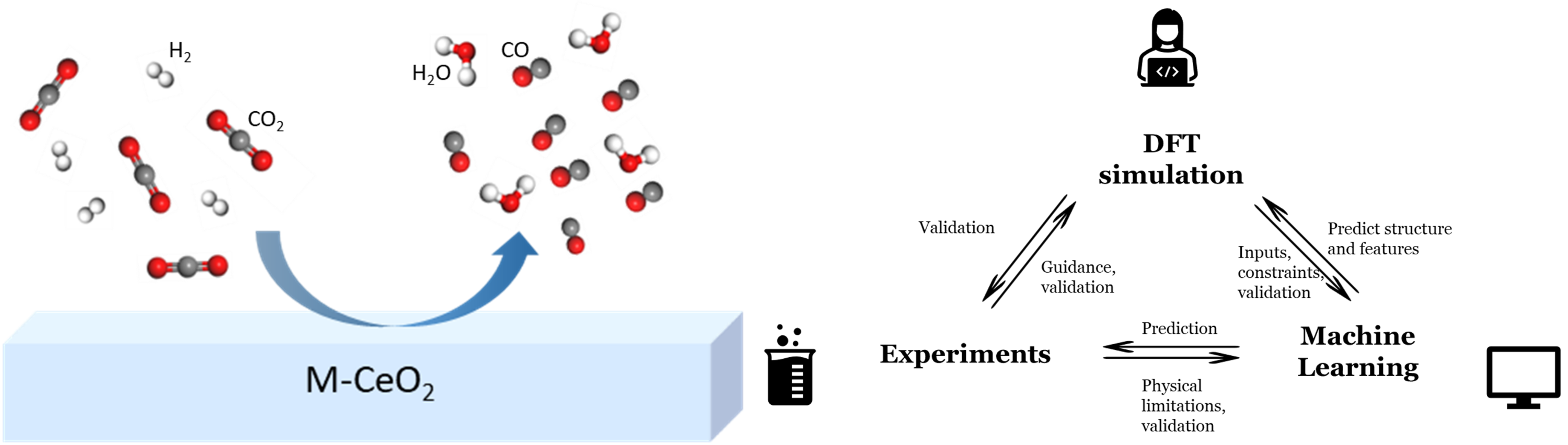
Figure 1. CO₂ hydrogenation via reverse water gas shift reaction using DFT, Machine Learning, and Laboratory Experiments to screen for a metal doped CeO₂ catalyst.
Optimal Operation of Industrial-Scale Facilities
A Nested Online Scheduling and Nonlinear Model Predictive Control Framework for Multi-Product Continuous Systems
The full paper can be found here.
Novel Process Intensification Systems
Optimal Design and Dynamic Transitions of Multitask Catalytic Distillation Columns: A Discrete-Steepest Descend Framework
Catalytic Distillation (CD) is a process that combines separation and heterogeneous reaction into a single unit, and it has recently gained attention for its potential to produce multiple products. Recent studies have shown successful optimization of transition policies and steady-state process designs for multigrade reactive distillation units, but the simultaneous integration of both tasks has not been considered. To avoid suboptimal or dynamically infeasible designs, it is critical to simultaneously optimize the design and dynamic transitions of a multigrade CD unit during the conceptual design stage.
The Discrete-Steepest Descent Algorithm (D-SDA) is an optimization framework that has been applied to the design and synthesis of CD columns using rigorous equilibrium and non-equilibrium models. The D-SDA handles these problems by fixing discrete variables, solving the resulting Nonlinear Programming (NLP) subproblems, and comparing the solution obtained within a neighborhood of the discrete variables at each iteration. If there is no better solution to the NLP subproblems within the neighborhood, then the algorithm stops; otherwise, the algorithm takes the steepest-descent direction to continue the search by performing another iteration. The D-SDA incorporates a reformulation step that allows to decrease the combinatorial difficulties of the problem associated with the presence of discrete design variables, e.g., number of stages and the location of feeds and catalytic stages. In addition, this algorithm applies a rigorous re-initialization strategy at each iteration to handle model nonlinearities, e.g., non-ideal vapor and liquid mixtures, reaction kinetic models, pressure drop calculations and hydrodynamic constraints. The D-SDA has shown better performance than other determinist MINLP solvers for solving CD steady-state design problems, thus making it a promising framework to handle the design and transient operation in CD processes.
This work presents a new version of the D-SDA that integrates process design and dynamic transitions of a multigrade CD process. The problem incorporates dynamic and steady-state operation requirements into a single large-scale Mixed-Integer Dynamic Optimization (MIDO) problem. The objective is to minimize investment and production costs, intensify production throughput and optimize the dynamic operation of a multigrade CD system. An improved version of the D-SDA that returns attractive solutions in reasonable computational times is used to tackle this challenging problem. The new D-SDA algorithm solves the integrated MIDO problem as a multi-scenario problem, where each scenario corresponds to one of the dynamic transitions involved in the process. Moreover, the new D-SDA incorporates a refined initialization strategy and an iterative procedure. At the initialization step, a feasible initialization is found using the conventional D-SDA applied to a steady-state version of the multi-scenario model. This results in an initial CD design that can accommodate multiple grades of a product. Iterations over discrete design variables is performed next. An iteration comprises the solution of the dynamic NLP subproblem for the discrete point being evaluated, and a collection of candidate neighbors that may improve the objective function. The key feature that differentiates the proposed iterative procedure from the conventional D-SDA’s methodology is the implementation of a new systematic procedure that eliminates feasible neighbors for which the overall objective function is not expected to improve, i.e., neighbors are discarded without the need to solve their corresponding dynamic NLP subproblems. For the remaining neighbors, the dynamic NLP subproblems are solved and used to search for an optimal solution using the steepest descent direction method. Overall, these improvements make the original MIDO problem computationally tractable.
The enhanced D-SDA algorithm was used to optimize the design and dynamic transitions of a multigrade CD unit to produce Ethyl Tert-Butyl Ether (ETBE) with different molar compositions. The results showed that the new D-SDA generated a different and more affordable design compared to the sequential design and dynamic transition strategy. Moreover, the new algorithm reduced computational time by decreasing the number of neighbors to be verified at each iteration, resulting in fewer dynamic optimization subproblems to be solved. Future work includes testing the new strategy with more challenging case studies, accounting for scheduling constraints, and investigating the performance of the proposed algorithm under closed-loop operation.
The full paper can be found here.
Nonlinear Model Predictive Control of a Large-Scale Packed Bed Chemical-Looping Combustion Process
In this work, a nonlinear model predictice control (NMPC) scheme is presented for chemical-looping combustion (CLC) in a large-scale packed bed reactor. CLC is a carbon capture process where an oxygen carrier is added to the process and alternatingly exposed to air (oxidation stage) and fuel (reduction stage). By avoiding contact between air and the fuel, it is possible to isolate the CO2 without an additional separation process, thereby employing process intensification.
When NMPC is performed for the oxidation stage of packed bed CLC, manipulating both the inlet air and inlet inert gas fluxes allowed the outlet gas temperature to track a given setpoint for longer than a constant inlet flux, generating energy for a longer period of time (Figure 1). When NMPC was implemented for the reduction stage, manipulating the inlet flux to control the outlet CO2 selectivity and flux, the product stream had improved CO2 selectivity (Figure 2). This significantly improved the carbon dioxide purity of the outlet stream and thus improved the carbon capture effectiveness of the process.
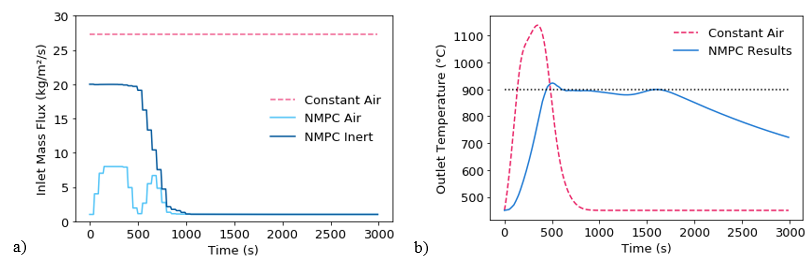
Figure 1. a) Inlet mass flux (air and inert) and b) outlet temperature profiles for the oxidation stage from using a constant inlet air flux (dashed pink lines) and from implementing the NMPC scheme (solid blue lines).
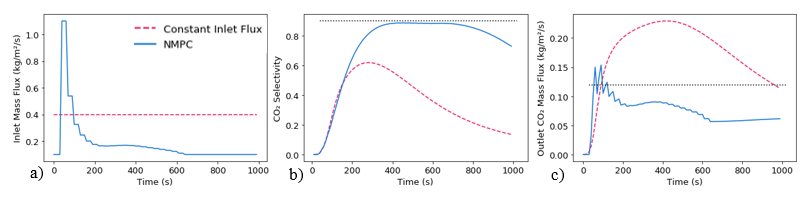
Figure 2. a) Inlet mass flux, b) CO2 selectivity, and c) outlet CO2 flux profiles for the reduction stage from using a constant inlet CH4 flux (dashed pink lines) and from implementing an NMPC scheme (solid blue lines).
Optimal Process Integration for Chemical and Manufacturing Systems
Integration of Design and NMPC-Based Control for Chemical Processes under Uncertainty: an MPCC-Based Framework
The full paper can be found here.