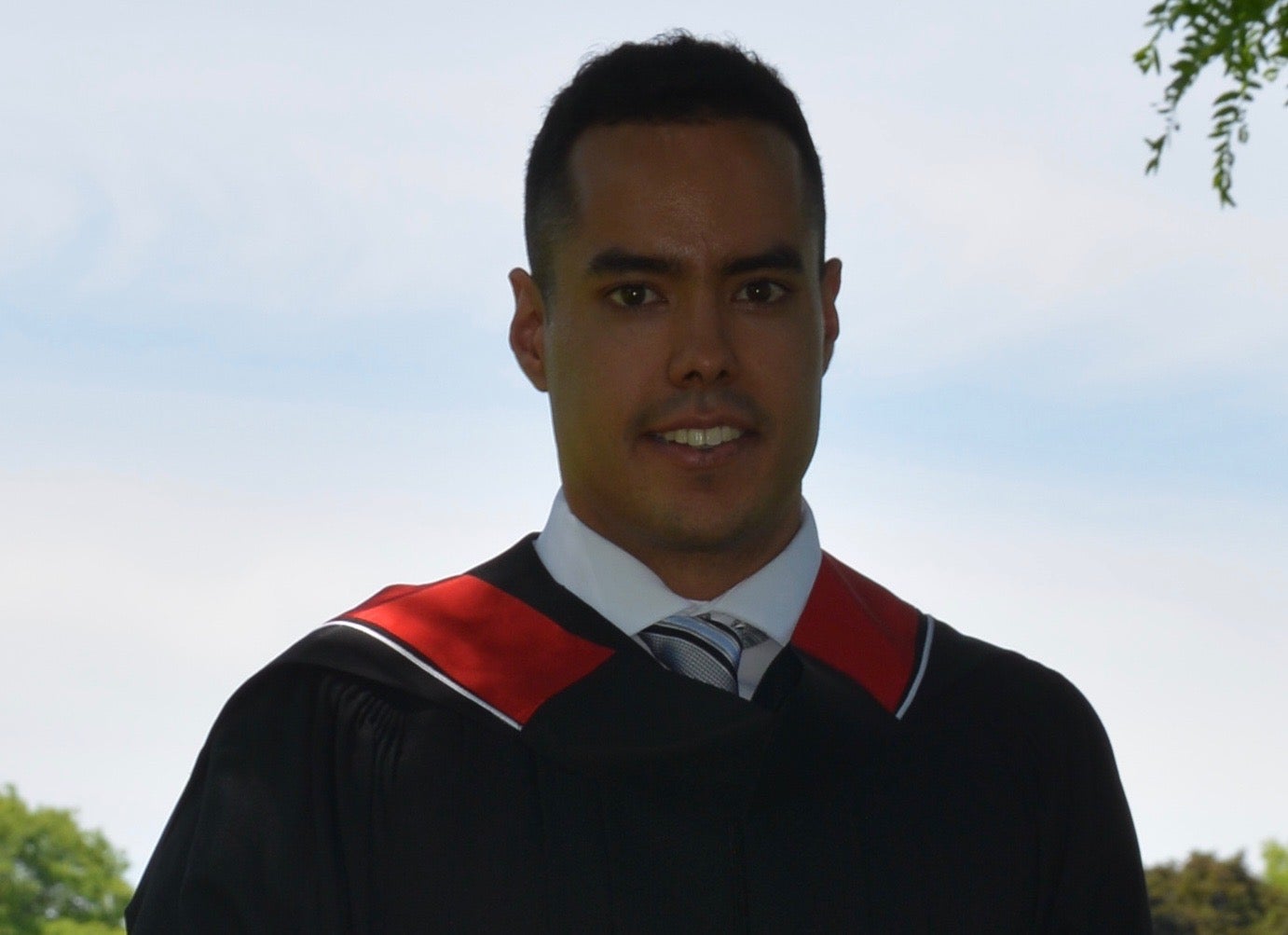
The main project of my thesis is the application of Adjustable Robust Optimization (ARO) to design robust Model Predictive Control (MPC) systems. MPC is a powerful control tool as it has the ability to drive a process to a desired set point in an optimal fashion while remaining feasible with respect to any constraints that have been imposed. The main disadvantage is that the optimization problem which makes up the MPC controller must be solved at every time step in the process. ARO has the potential to make MPC amenable to larger processes by significantly reducing the computational time required to calculate the following control action. Like other explicit MPC schemes, rather than solving the optimization problem at every time step, the problem is solved offline. The result is a set of linear control laws which are a function of the state of the system. To determine the following control action, the controller simply evaluates the control laws based on the measured state rather than solving an optimization problem.