Artificial Intelligence and Pharmaceutical Dosing
Large datasets exist that itemize the physical and biochemical status of healthcare study participants. Current interpretation of this data typically considers the median, standard deviation, and mean statistics for each dataset. These studies rely heavily upon the normal distribution when interpreting these results to determine attributes such as probability of occurrence as well as significant differences between populations. Moreover, these studies use these statistics to infer causal relationships to changes in the location, scale and shape of the distributions with external influences such as; age, weight, gender, pre-existing conditions, exercise, diet, geographic location, and pharmaceutical dosing, among others. Baseline studies provide a foundation for determining the influence of external influences in changing physical and biochemical measures. Example studies include those conducted by the National Center for Health Statistics (Centers for Disease Control and Protection) as shown on the histograms below may include physical measures such as blood pressure and heart rate or biochemical measures such as creatinine production or cholesterol levels, among many others.
Fitting the histograms with parametric PDFs provides data compression as well as a time-continuous function to determine the influence of external influences (causality) on changes in the location, scale and shape of the distribution. Pharmaceutical dosing of blood pressure medication on a test group extracted from the population shown on the histogram may reduce the instances of high-blood pressure measurements, thus changing the location, scale, and shape of the distribution defining the test group relative to its initial overlap with the baseline distribution. The parametric probability density function accurately measures the probability of occurrence of patients within these regions. Furthermore, a single time-continuous set of parameters defining the location, scale and shape of the distribution can be correlated to attributes of the dose thereby inferring a causal relationship of how a test group responds to a prescription.
The advantage of our methodology to physicians and health care professionals is that the compressed data set of location, scale and shape information of the distribution of patient responses can be monitored and attributed to a prescription-based dosage of pharmaceutical drugs. Moreover, the methodology is amenable to machine learning algorithms that can be trained to replicate physicians response to the output analysis and further infer casual relationships between patients and response to their assigned drug dosage.
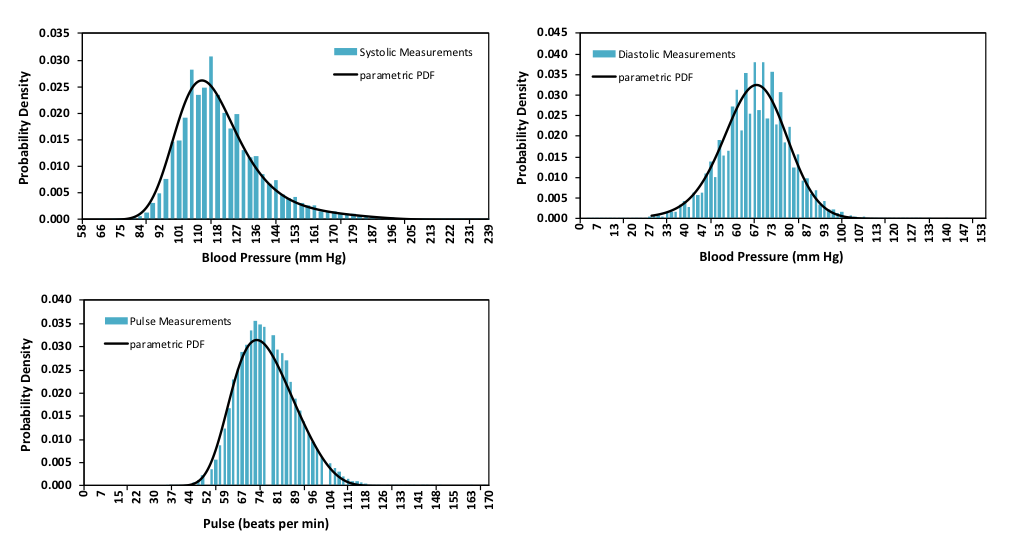
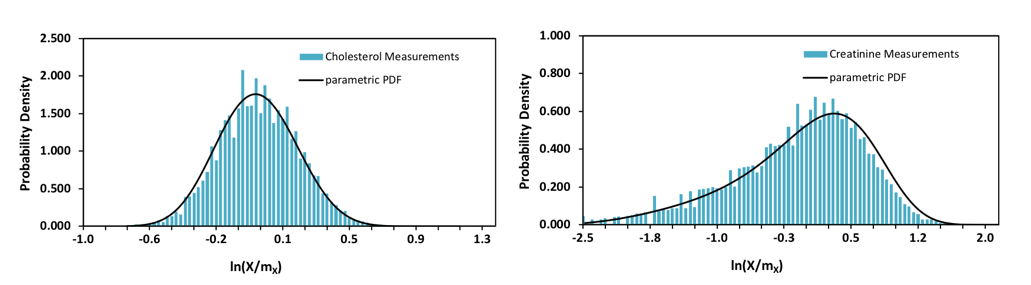