We have developed and used a variety of simulators for experiments studying human-machine interaction.
Simulators provide a safe and well-controlled environment for studying human factors. The human data collected from such experiments are used to build and validate human performance models. The modelling approach can quantitatively predict human factors constructs including performance, mental workload, and situation awareness. The models can be used to test human performance theories and also serve as engineering tools for the analysis and prediction of human performance.
- Driving simulators: The Open Racing Car Simulator (TORCS) human experiment version, OpenDS, CARLA.
- Flight simulators: X-Plane.
- Cognitive architectures for human performance modelling: adaptive control of thought - rational (ACT-R), queueing network-adaptive control of thought rational (QN-ACTR).
- Human-machine interface simulation in domains such as power plant control room and process industry procedural tasks.
Related publications
- Xu, R., Cao, S., Kearns, S., Niechwiej-Szwedo, E., & Irving, E. (2024). Computational cognitive modeling of pilot performance in pre-flight and take-off procedures. Journal of Aviation/Aerospace Education and Research.
- Sun, X., Cao, S., & Tang, P. (2020). Shaping driver-vehicle interaction in autonomous vehicles: How the new in-vehicle systems match the human needs. Applied Ergonomics [IF 2019: 3.145].
- Zhu, A., Cao, S., Jadliwala, M., & He, J. (2020). Can wearable devices facilitate a driver’s brake response time in a classic car-following task? IEEE Access [IF 2019: 3.745], 8, 40081-40087.
- Cao, S., Nandakumar, K., Babu, R., & Thompson, B. (2020). Game play in virtual reality driving simulation involving head-mounted display and comparison to desktop display. Virtual Reality [IF 2019: 2.941], 24(3), 503-513.
- Rehman, U., & Cao, S. (2020). Comparative evaluation of augmented reality-based assistance for procedural tasks: A simulated control room study. Behaviour and Information Technology [IF 2019: 1.781], 39(11), 1225-1245.
- Deng, C., Cao, S., Wu, C., & Lyu, N. (2019). Modeling driver take-over reaction time and emergency response time using an integrated cognitive architecture. Transportation Research Record [IF 2019: 1.029], 2673(12), 380-390.
- Cao, S., Chan, K., & Elkamel, A. (2019). A controlled experiment investigating the effects of explanatory manual on adherence to operating procedures. Safety, 5(2), 1-12.
- Cao, S., Ho, A., & He, J. (2018). Modeling and predicting mobile phone touchscreen transcription typing using an integrated cognitive architecture. International Journal of Human-Computer Interaction [IF 2019: 2.902], 34(6), 544-556.
- Guo, Z., Pan, Y., Zhao, G., Cao, S., Zhang, J. (2018). Detection of driver vigilance level using EEG signals and driving contexts. IEEE Transactions on Reliability [IF 2019: 3.177], 67(1), 370-380.
- Cao, S., & Liu, Y. (2015). Modelling workload in cognitive and concurrent tasks with time stress using an integrated cognitive architecture. International Journal of Human Factors Modelling and Simulation, 5(2), 113-135.
- Cao, S., Qin, Y., Zhao, L., & Shen, M. (2015). Modeling the development of vehicle lateral control skills in a cognitive architecture. Transportation Research Part F: Traffic Psychology and Behaviour [IF 2019: 2.518], 32, 1-10.
- Cao, S., Qin, Y., Jin, X., Zhao, L., & Shen, M. (2014). Effect of driving experience on collision avoidance braking: An experimental investigation and computational modelling. Behaviour and Information Technology [IF 2019: 1.781], 33(9), 929–940.
- He, J., Chaparro, A., Nguyen, B., Burge, R., Crandall, J., Chaparro, B., Ni, R., & Cao, S. (2014). Texting while driving: Is speech-based text entry less risky than handheld text entry? Accident Analysis and Prevention [IF 2019: 3.655], 72, 287–295.
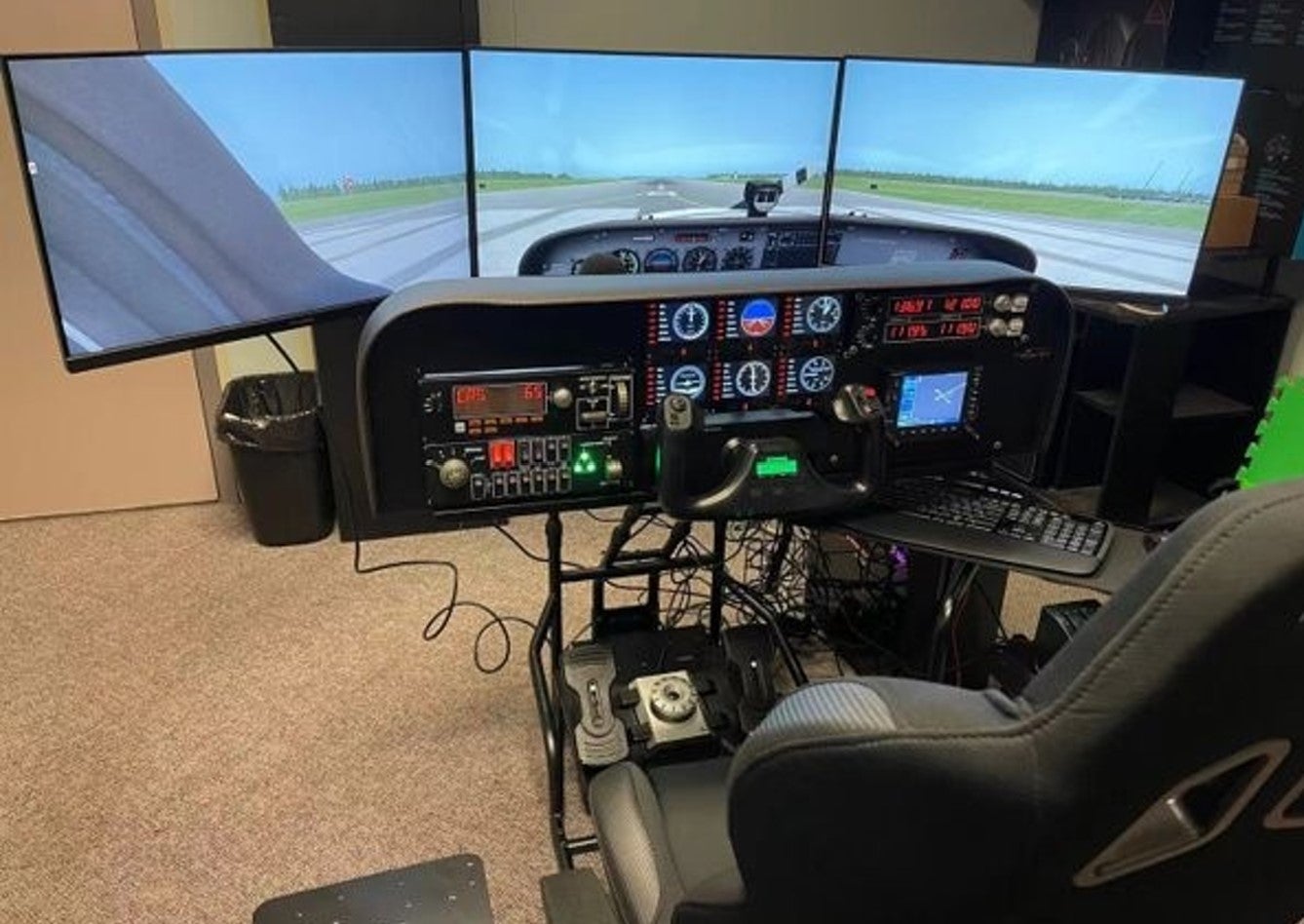
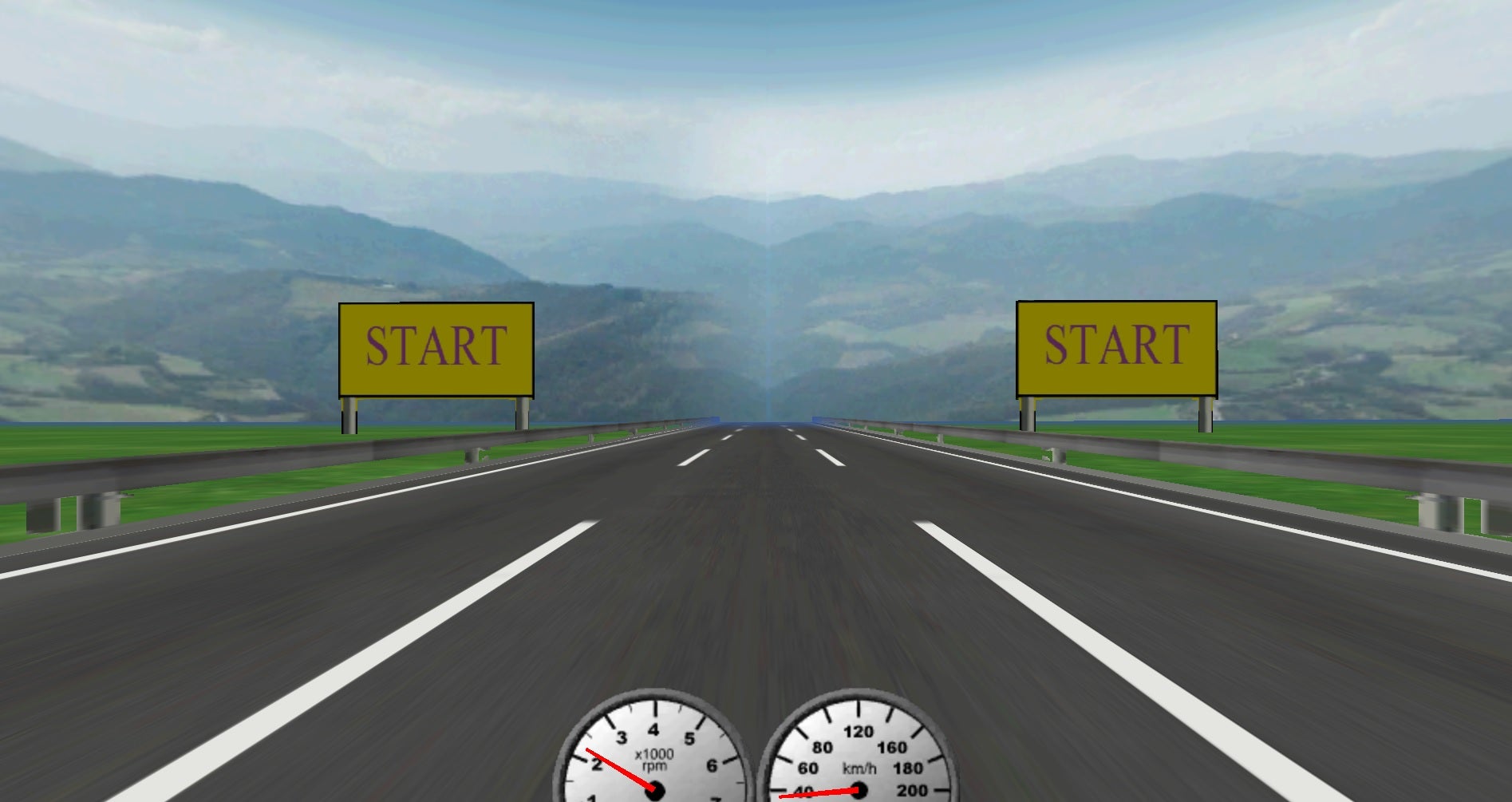
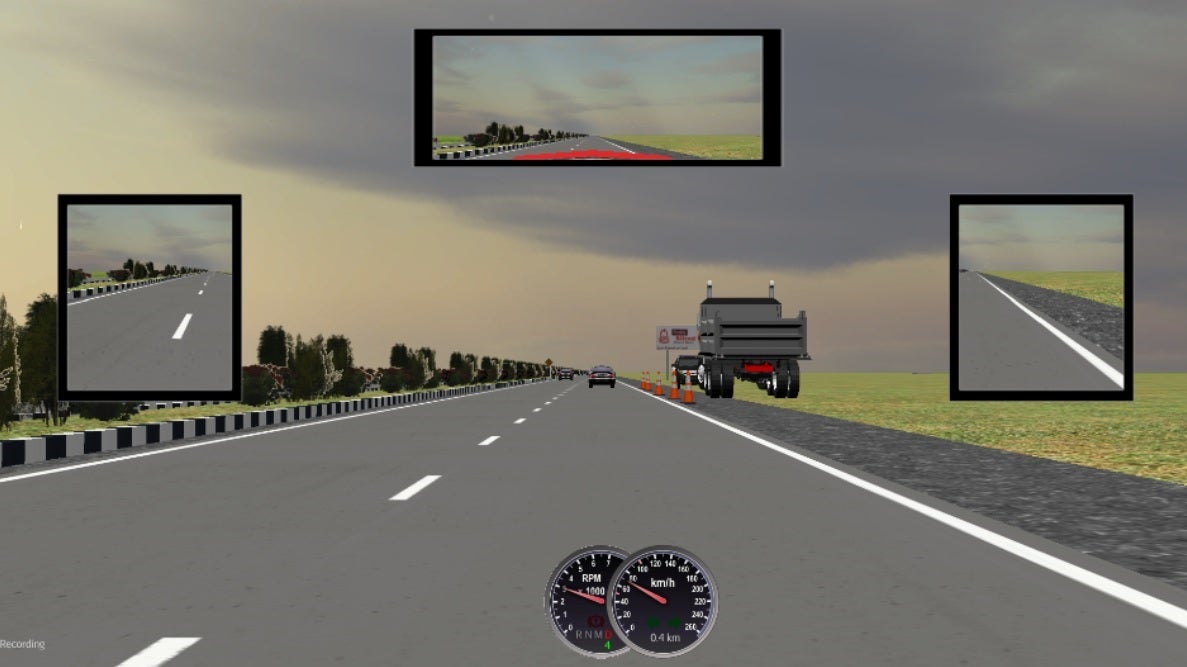
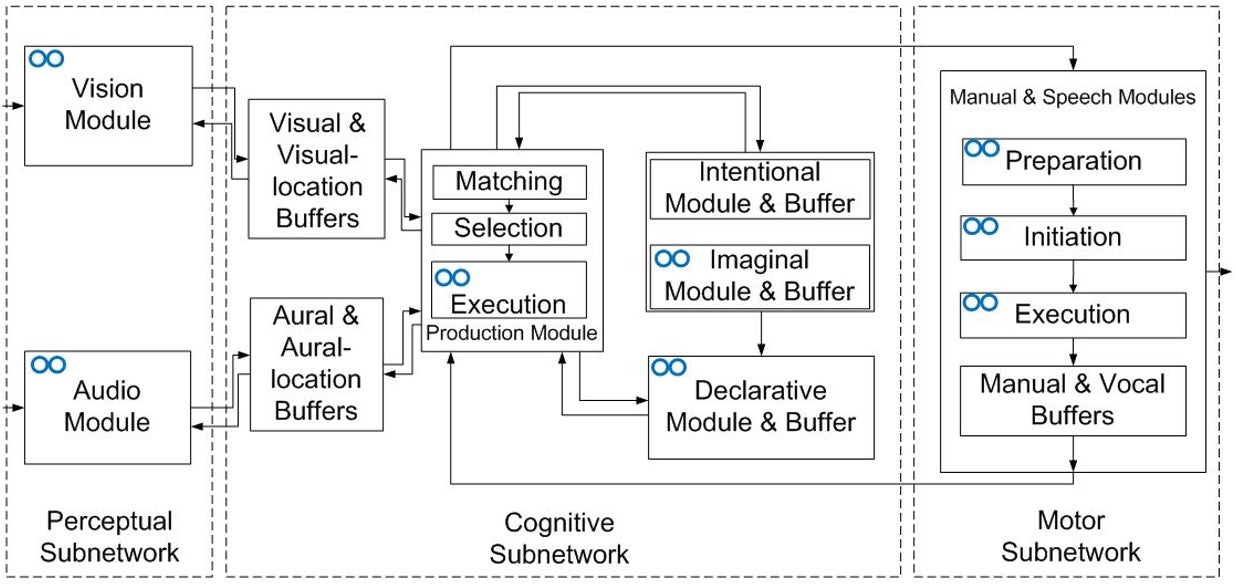
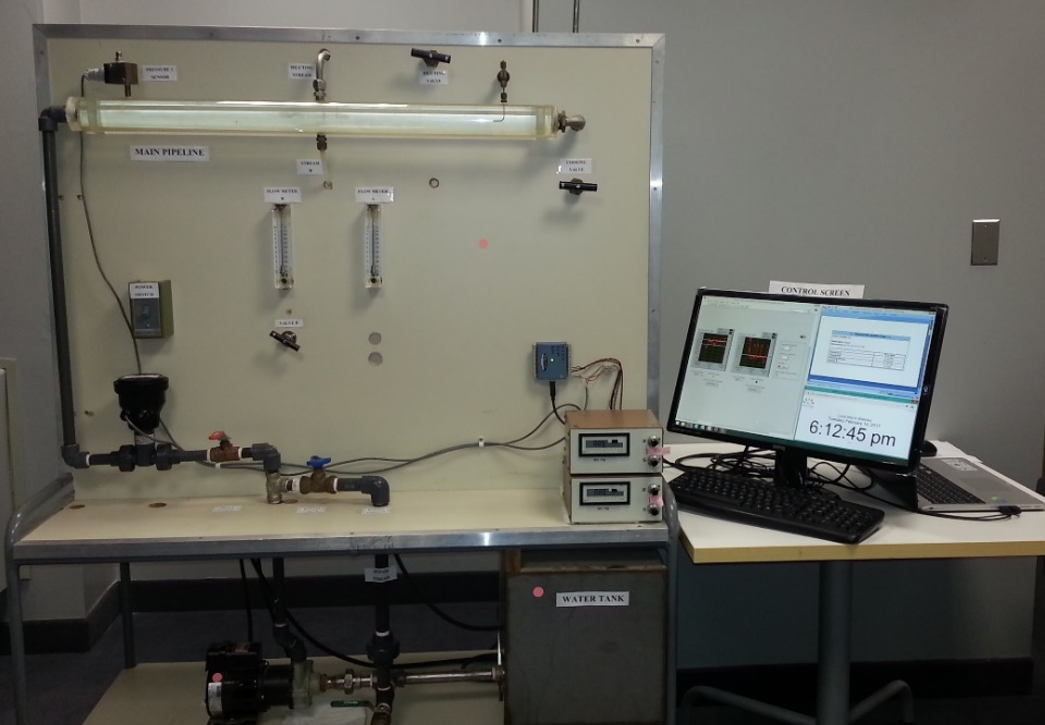