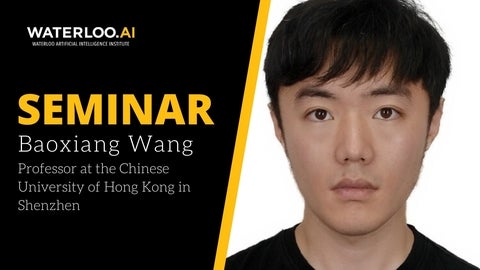
Title: Information Design in Multi-Agent Reinforcement Learning
Abstract: Reinforcement learning (RL) mimics how humans and animals interact with the environment. The setting is somewhat idealized because, in actual tasks, other agents in the environment have their own goals and behave adaptively to the ego agent. To thrive in those environments, the agent needs to influence other agents so their actions become more helpful and less harmful. Research in computational economics distills two ways to influence others directly: by providing tangible goods (mechanism design) and by providing information (information design). We investigate information design problems for a group of RL agents. Main challenges on non-stationarity and persuasiveness are addressed. The algorithm is tested on various mixed-motive tasks. The work gives further insights into game theory and computational economics.
Speaker Bio: Baoxiang Wang is an assistant professor at The Chinese University of Hong Kong, Shenzhen. He's currently visiting University of Waterloo for the summer of 2023. Baoxiang works on reinforcement learning, multi-agent learning, and learning theory. He obtained his Ph.D. in Computer Science and Engineering from The Chinese University of Hong Kong in 2020, fortunate to be advised by Siu On Chan and Andrej Bogdanov. Before that, He obtained B.E. in Information Security from Shanghai Jiao Tong University in 2014.
Date: Friday, July 21st, 2023
Time: 11:00 AM - 12:00 PM EST
Location: E7 2324/2328–MBET Classroom
This seminar will be hybrid.