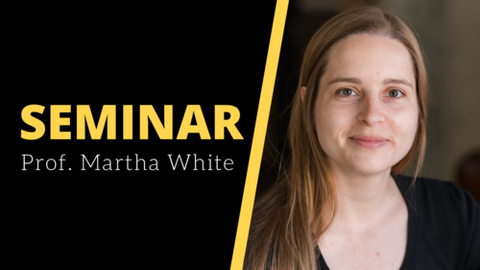
Title: Developing Reinforcement Learning Agents that Learn Many Subtasks
Abstract: Learning agents operating in complex environments must accumulate knowledge about the environment to continually improve. This knowledge can take the form of a dynamics model, option policies that achieve certain subgoals and long-term predictions in the form of general value functions. All of these are subtasks that the agent can learn about in parallel, to improve performance on the primary task: accumulating reward. When we commit to the perspective that our reinforcement learning agents need to discover, learn and use many subtasks, new algorithmic considerations arise. The agent needs to answer: how can I direct data gathering (exploration) to learn these subtasks efficiently? How can I learn these subtasks in parallel, from a single stream of experience, and maintain stability under these off-policy (counterfactual) updates? In this talk, I will motivate the need to develop such agents, as well as insights into how to efficiently learn these subtasks using directed exploration and off-policy algorithms.
Speaker Bio: Martha White is an Associate Professor of Computing Science at the University of Alberta and a PI of Amii--the Alberta Machine Intelligence Institute--which is one of the top machine learning centres in the world. She holds a Canada CIFAR AI Chair and received IEEE's "AIs 10 to Watch: The Future of AI" award in 2020. She has authored more than 50 papers in top journals and conferences. Martha is an associate editor for TPAMI, and has served as co-program chair for ICLR and area chair for many conferences in AI and ML, including ICML, NeurIPS, AAAI and IJCAI. Her research focus is on developing algorithms for agents continually learning on streams of data, with an emphasis on representation learning and reinforcement learning.
Recording (YouTube): https://youtu.be/GmGL9cVfJG4