Congratulations to He Zhao
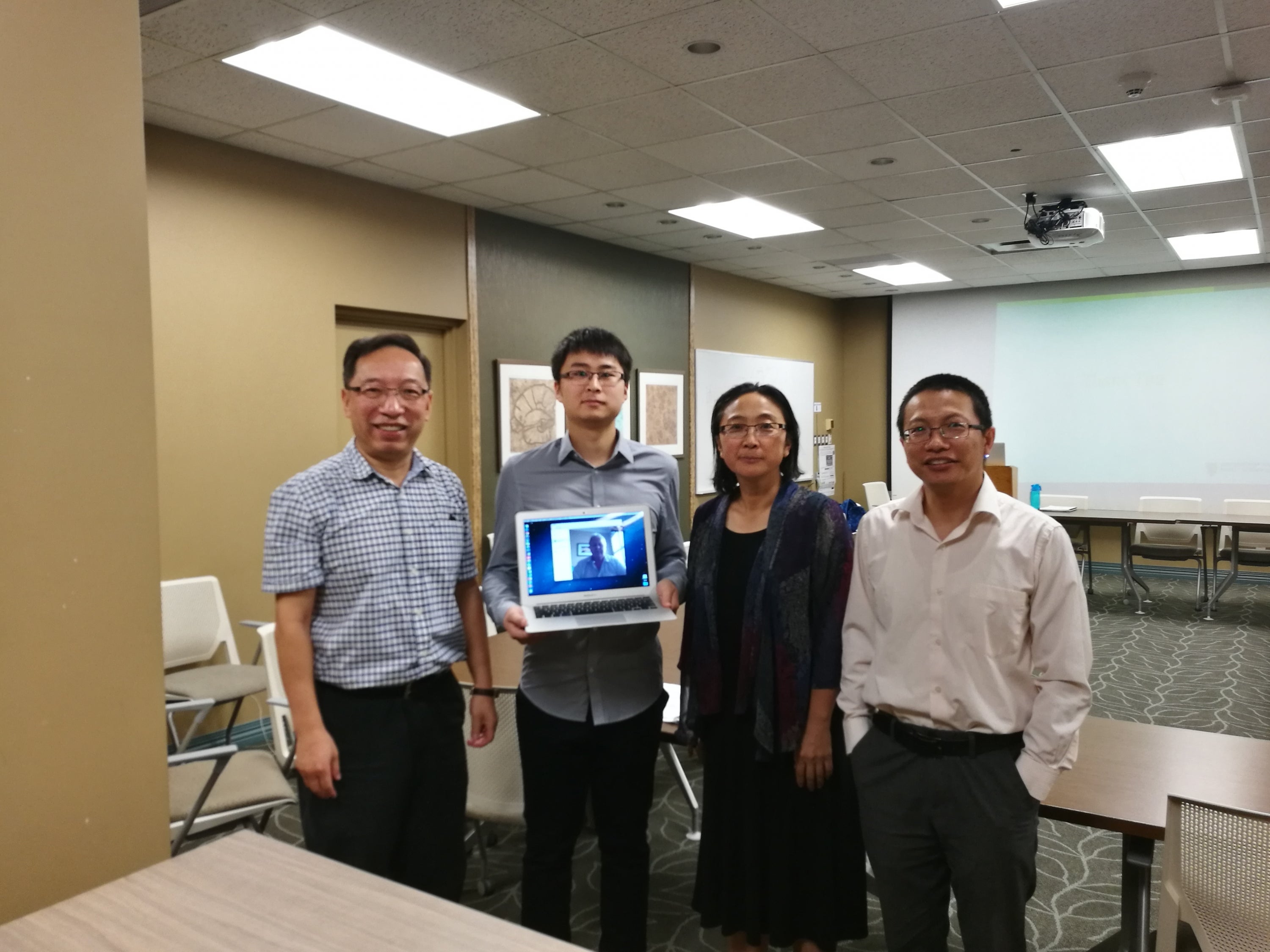
Thesis abstract:
In the field of precise localization and navigation, the sensors mounted on a driverless vehicle are not always reliable. In some cases, a subtle perceptual error may cause devastating consequences. Therefore, map-aided localization has become an urgent demand. By comparing the real-time sensory detection data with a priori map, the autonomous navigation system can transform the complicated sensor perception mission into a simple map-based localization task. However, the lack of robust solutions and standards for creating such 3D high-definition road maps is a major challenge in this emerging field.
This study develops a semi-automated method for extracting meaningful road features from mobile laser scanning (MLS) point clouds and creating precise road maps for autonomous vehicles. After pre-processing steps including coordinate system transformation and non-ground points removal, a road edge detection algorithm is performed to distinguish road curbs and extract road surfaces. In this method, road markings are divided into two categories and separately extracted. On the one hand, textual and directional road markings including arrows, symbols, and words are detected using intensity thresholding and the conditional Euclidean clustering algorithm. On the other hand, lane markings are extracted by local intensity analysis and distance thresholding according to road design standards. Afterwards, centerline points in every single lane are estimated based on the position of the extracted lane markings. Ultimately, 3D road maps with precise road boundaries, road markings, and the estimated lane centerlines are created.
The experimental results demonstrate the feasibility of the proposed method, which can accurately extract most targeted road features from the point clouds. The average completeness, correctness, and F-score for road marking extraction are 93.87%, 93.76%, and 93.73%, respectively. All of the estimated lane centerlines are manually validated through geo-referenced UAV orthoimages. The proposed method is also compared with some other existing methods. The comparison results further prove the robustness of our method.