Dr. Juliane Mai wins Jim Dooge Award for outstanding paper from European Geosciences Union
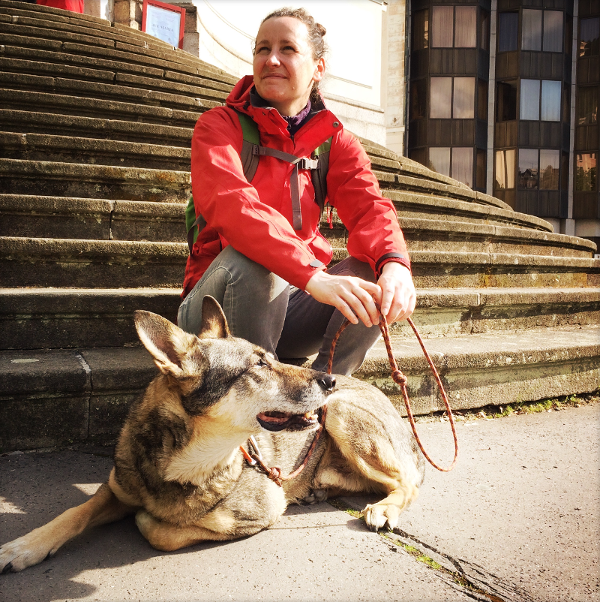
The European Geosciences Union (EGU), the leading organization for Earth, planetary and space science research in Europe, has awarded the Jim Dooge Award to Water Institute member Juliane (Julie) Mai, Research Associate Professor in the Department of Earth and Environmental Sciences. The award was announced April 16th at the 2024 EGU general assembly in Vienna, Austria.
Photo: Dr. Juliane (Julie) Mai, Research Associate Professor in the Department of Earth and Environmental Sciences.
In memoriam of the ground-breaking contributions of Jim Dooge, Hydrology and Earth System Sciences honours the most outstanding paper of the year with this prestigious award.
Mai’s paper ‘The Great Lakes Runoff Intercomparison Project Phase 4: the Great Lakes (GRIP-GL)’ published in Hydrology and Earth System Sciences conducts an immense multi-model comparison across the Great Lakes Basin.
In the study, which also included Water Institute members Bryan Tolson and James Craig from the Department of Civil and Environmental Engineering, 13 different models were put to the test, ranging from machine-learning-based, basin-wise, subbasin-based, and gridded models that are either locally or globally calibrated or calibrated for one of each of the six predefined regions of the watershed. They all used the same data and were evaluated across the vast study area of over 1 million square kilometers.
"I am honoured to receive the Jim Dooge Award. Model inter-comparison exercises are increasingly recognized as the critical step for informed environmental decision making; however, what is often not appreciated is the significant effort it takes to bring diverse models together," said Dr. Mai.
"In GRIP-E and GRIP-GL, we brought 30 models, 38 researchers and 12 institutions together through monthly meetings over 5 years - through many many hours of intense interactions, we managed to develop a vibrant community platform, with connections forged that will last beyond the duration of the project."
Receiving this award is a testament to the power of collaboration in shaping hydrological research and practice.
By comparing model outputs to reference data after all models where setup in a highly standardized fashion that is unheard of in the hydrologic community, researchers gained insight into each model's strengths and weaknesses.
Key findings included:
- The comparison of models for streamflow revealed the superior quality of the machine-learning-based model in all experiments; even for the most challenging spatiotemporal validation, the machine learning model outperformed any other physically based model.
- While the locally calibrated models led to good performance in calibration and temporal validation (even outperforming several regionally calibrated models), they lost performance when transferred to locations that the model has not been calibrated on.
- The regionally calibrated models – while losing less performance in spatial and spatiotemporal validation than locally calibrated models – exhibited low performance in highly regulated and urban areas and agricultural regions in the USA.
- Comparisons of additional model outputs against gridded reference datasets showed that aggregating model outputs and the reference dataset to the basin scale can lead to different conclusions than a comparison at the native grid scale.
- A multi-objective-based analysis of the model performances across all variables revealed overall well-performing locally calibrated models and regionally calibrated models due to varying reasons.
- All basin-aggregated model outputs and observations for the model variables evaluated are available on an interactive website that enables users to visualize results and download the data and model outputs.
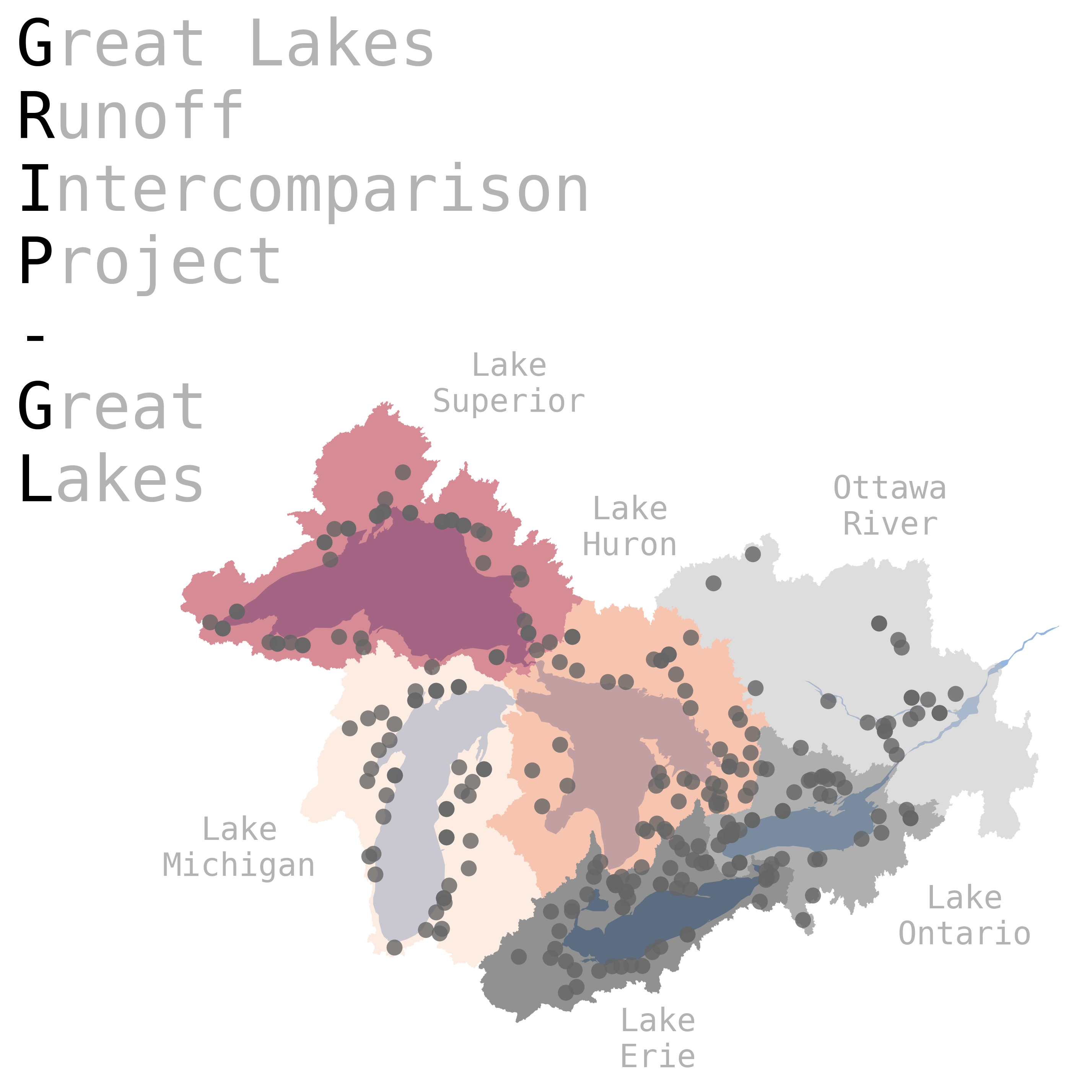
The multi-model comparison, supported by the Global Water Futures project at the University of Waterloo, improved understanding of water systems in this crucial region, helping us better manage water resources for the future.
Learn more about the project on Dr. Mai’ GRIP-GL website.
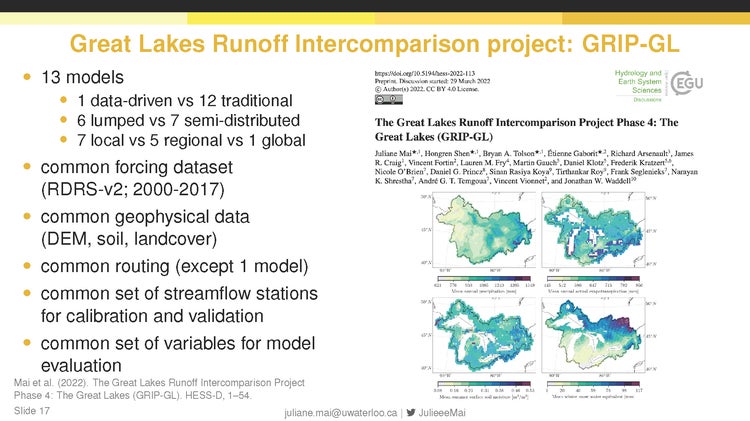