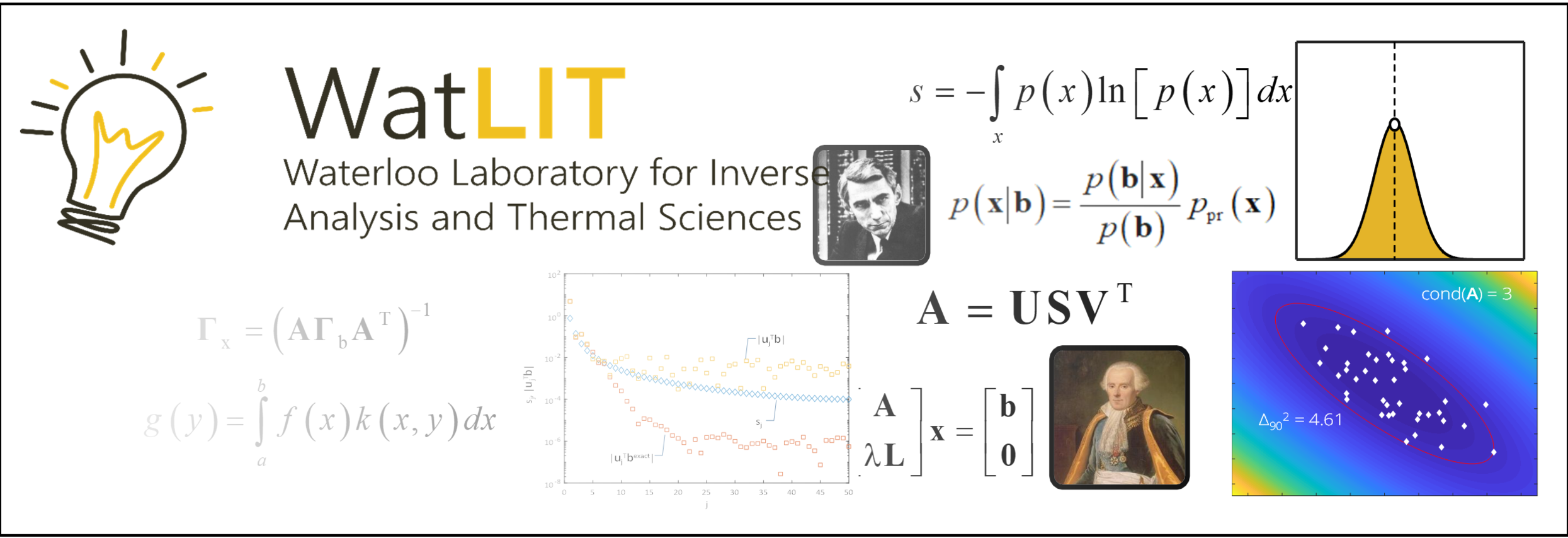
What is an inverse problem?
Much science and engineering research is devoted to modeling physical systems. Given a complete description of the physical system, these models can be used to predict how the system affects its surroundings. This is called the direct or forward problem. When some aspects of physical system are unknown, however, it is sometimes possible to infer these characteristics from a known system response; this is the inverse problem.
Mathematically ill-posed problems
Inverse problems are challenging to solve because they are ill-posed. Ill-posed problems violate one or more of the criteria for well-posed problems:
The problem must have a solution;
The solution must be unique, and;
The solution must be stable under small changes to the data.
At first, mathematicians thought problems that violated these criteria made no practical or physical sense, and that there was no need to attempt solutions to these problems. It has subsequently been shown, however, that mathematically ill-posed inverse problems are ubiquitous throughout science and engineering.
Inverse problems can be categorized as either parameter estimation or inverse design problems. Both problems may be ill-posed, and require prior information to solve them.
Parameter estimation
The goal of many experiments is to infer a property or attribute from data that is indirectly related to the unknown quantity. Parameter estimation problems usually satisfy the first criterion of well-posed problems, since something is responsible for the observed system response. Instead, they violate the third criterion and "almost" violate the second criterion because many different candidate solutions exist that, when substituted into the measurement model, produce very similar data. If different system configurations produce similar observed data, the corollary is that the recovered solution will be highly sensitive to small perturbations in the data, which are inevitable in an experiment.
A classic example is the problem of a knight trying to guess what kind of dragon he is hunting from footprints he finds in the forest. This is a difficult task if many types of dragon produce similar footprints, and becomes impossible if the footprints are even slightly smeared in mud.
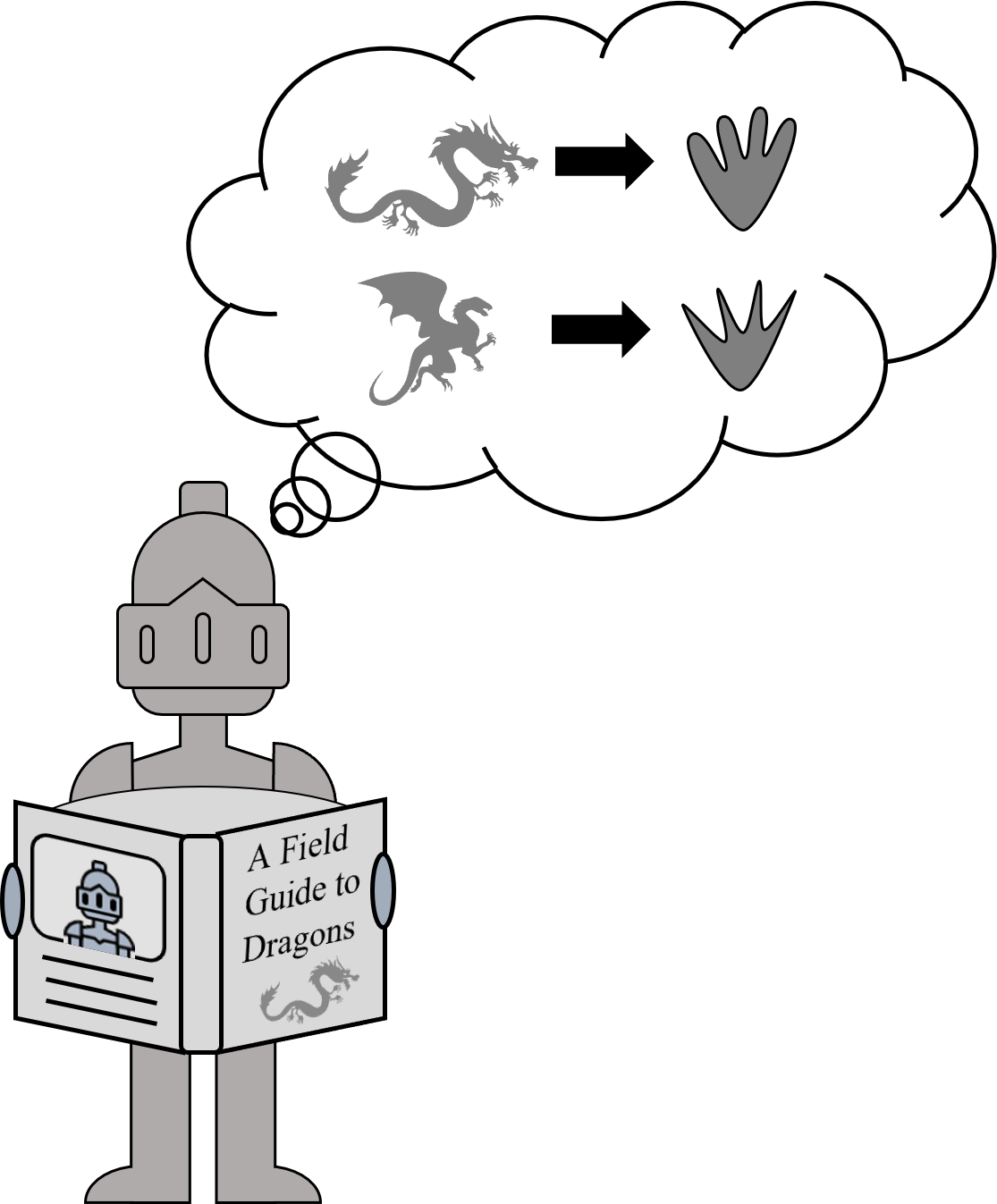
Forward problem
What kind of footprints does each dragon produce?
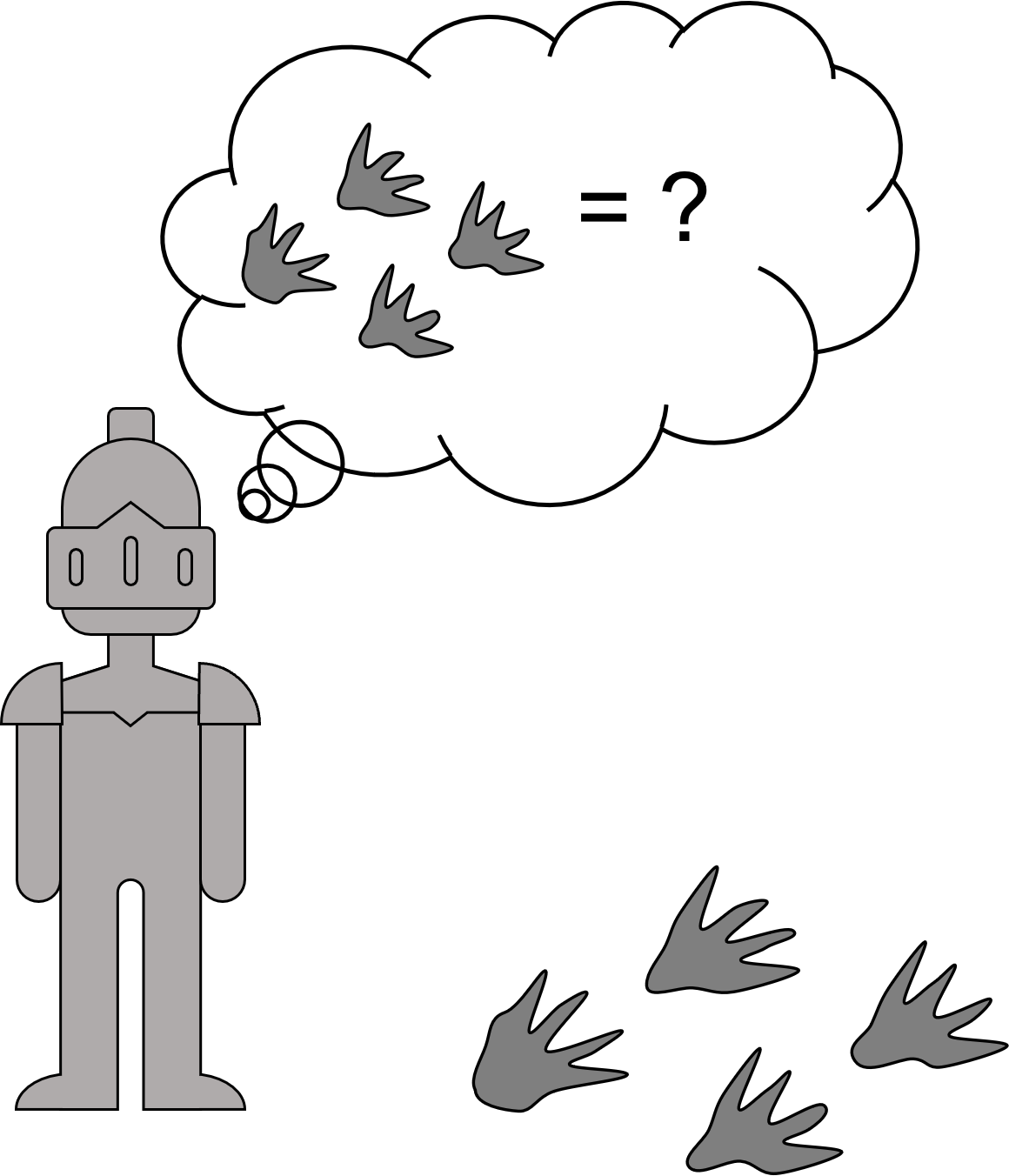
Inverse problem
What dragon left the footprints? (violation of stability criterion)
Inverse design problems
Unlike parameter estimation, inverse design problems often violate Hadamard’s first criterion since an optimal design outcome may be specified that cannot possibly be produced by the system. On the other hand, the existence of multiple designs that produce an acceptable outcome violates the second criterion. In an everyday example, say you go to the store to buy concentrated orange juice. Hadamard's second criterion would be violated if the store has two brands of orange juice, both of which taste good and cost the same. If the store were sold out of orange juice, Hadamard's first criterion would be violated.
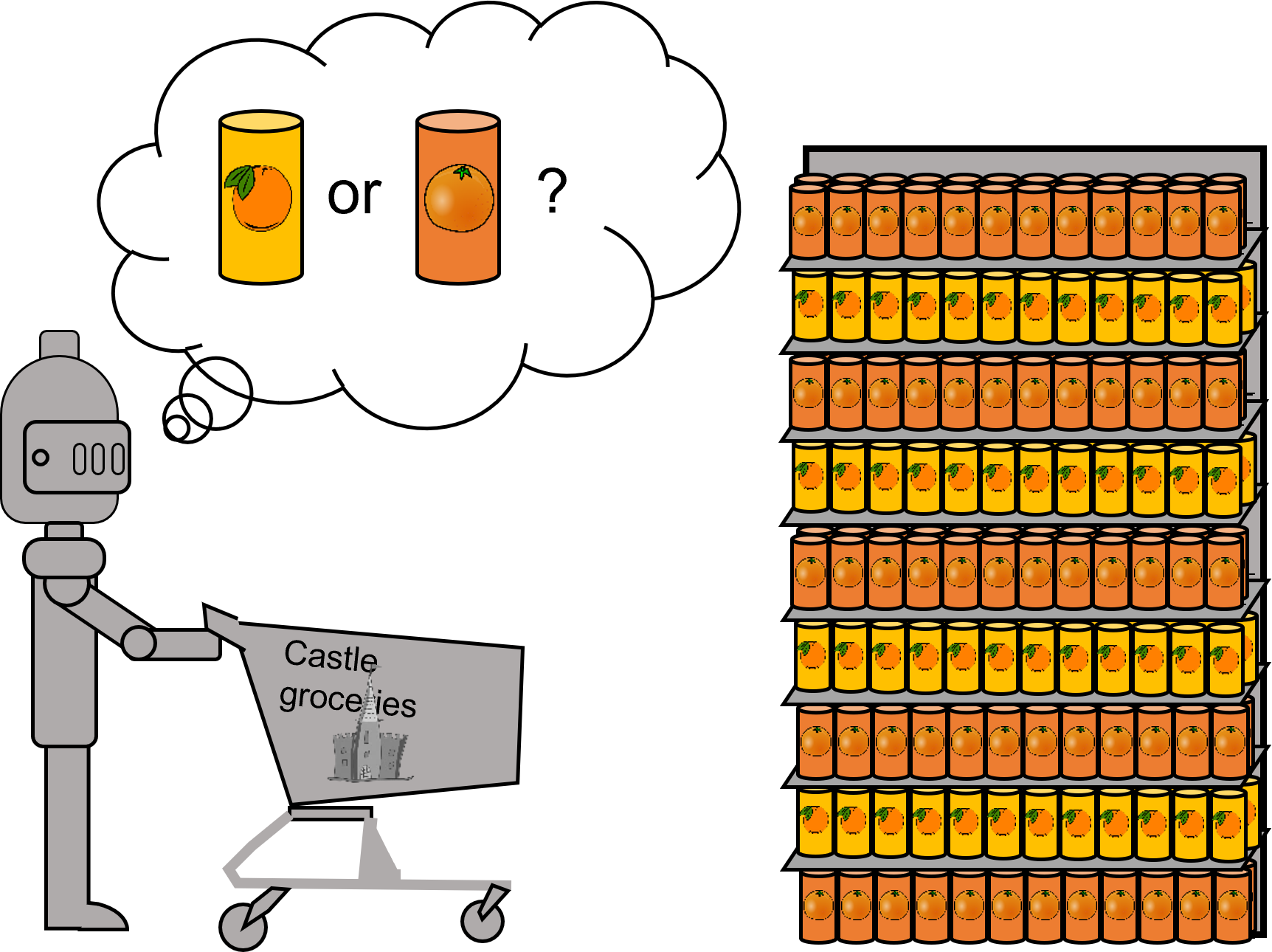
Design optimization can be ill-posed
Either brand of OJ is fine! (Violation of uniqueness criterion)
Role of prior information
Inverse problems are mathematically ill-posed due to an information deficit. In the parameter estimation case, the measurements barely provide sufficient information to specify a unique solution, and in some cases the data could be explained by an infinite set of candidate solutions. Accordingly, inverse analysis resolves this ambiguity by injecting additional information into the problem. Often, this information is based on expectations of the solution; for example, an expected range of nanoparticle sizes, a degree of spatial smoothness in a species concentration distribution, or additional desired attributes of an optimal design. A classic example is the "backwards reasoning" used by Sherlock Holmes.
In this example, the observations by themselves (“sunbaked skin”, “ammunition boots”, "large stature," "wearing black clothes", etc.) tell Sherlock and Mycroft very little about the situation, but a complete picture is obtained when these observations are combined with prior knowledge (British soldiers of that era served in Afghanistan, black clothes denoted mourning in Victorian society, etc.)
When combining prior knowledge with measurements, however, care must be taken to ensure that the information obtained from these two sources is weighted appropriately. If not enough emphasis is placed on the prior knowledge, the solution will be ambiguous. On the other hand, if too much emphasis is placed on the prior knowledge, the inferred solution will correspond exactly with expectations; one could think of this as "the Law of Self-Fulfilling Prophecies.“
Bayesian inference
Information from measurement data and prior information can be combined through Bayes’ equation to produce estimates for the Quantities-of-Interest (QoI). In this approach the measurements, b, and the QoI, x, are interpreted as random variables that obey probability density functions (PDFs). The width of the PDFs describes how much is known about each parameter. The PDFs are related by Bayes’ equation
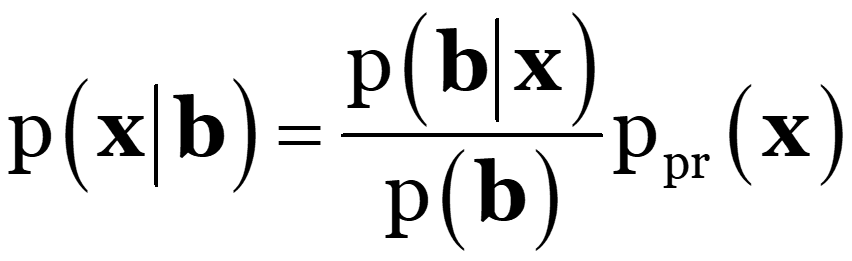
where p(b|x) is the likelihood of the observed data occurring for a hypothetical x, accounting for measurement noise and model error (“likelihood PDF”), ppr(x) defines what is known before the measurement takes place, (“prior PDF”), p(x|b) is the posterior PDF, which defines what is known about x from both the measurements and prior information, and p(b) is the evidence, which scales the posterior so that it satisfies the Law of Total Probabilities.
The posterior probability provides an unambiguous measure of uncertainty, e.g. “the correct answer is contained within an interval [xa, xb] with 95% probability. We have used this technique for laser-based nanoparticle diagnostics, chemical species tomography, optical gas imaging, and steel pyrometry.
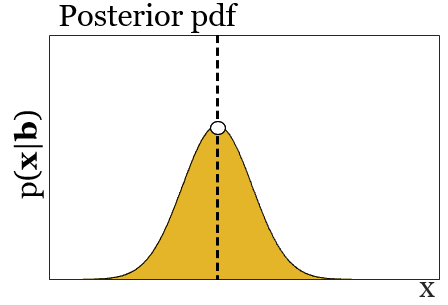
What is known about x after the measurement
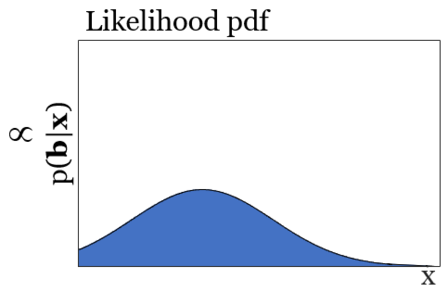
Information from the measurement data
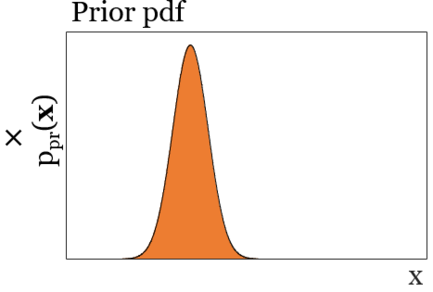
What is known about x before the measurement