Portable ultrasound is recently emerging as a new medical imaging modality featuring high portability, easy connectivity, and real-time on-site diagnostic ability. However, it does not yet provide ultrasound elastography function that enables the diagnosis of malignant lesions using elastic properties. This is mainly due to the limitations of hardware performance and wireless data transfer speed for processing the large amount of data for elastography. Therefore, data transfer reduction is one of the feasible solutions to overcome these limitations. Recently, compressive sensing (CS) theory has been rigorously studied as a means to break the conventional Nyquist sampling rate and thus can significantly decrease the amount of measurement signals without sacrificing signal quality. In this research, we implemented various CS reconstruction frameworks and comparatively evaluated their reconstruction performance for realizing ultrasound elastography function on portable ultrasound. Combinations of three most common model bases (Fourier transform [FT], discrete cosine transform [DCT], and wave atom [WA]) and two reconstruction algorithms (L1 minimization and block sparse Bayesian learning [BSBL]) were considered for CS frameworks. Echoic and elastography phantoms, were developed to evaluate the
performance of CS on B-mode images and elastograms. To assess the reconstruction quality, mean absolute error (MAE), signal-to-noise ratio (SNRe), and contrast-to-noise ratio (CNRe) were measured on the B-mode images and elastograms from CS reconstructions. Results suggest that CS reconstruction adopting BSBL algorithm with DCT model basis can yield the best results for all the measures tested, and the maximum data reduction rate for producing readily discernable elastograms is around 60%.
This research is conducted in collaboration with Prof. Hyock-Ju Kwon.
- Schematic of the procedure to construct a virtual elastography phantom and to produce elastogram from the undersampled RF data of the phantom using CS reconstruction.
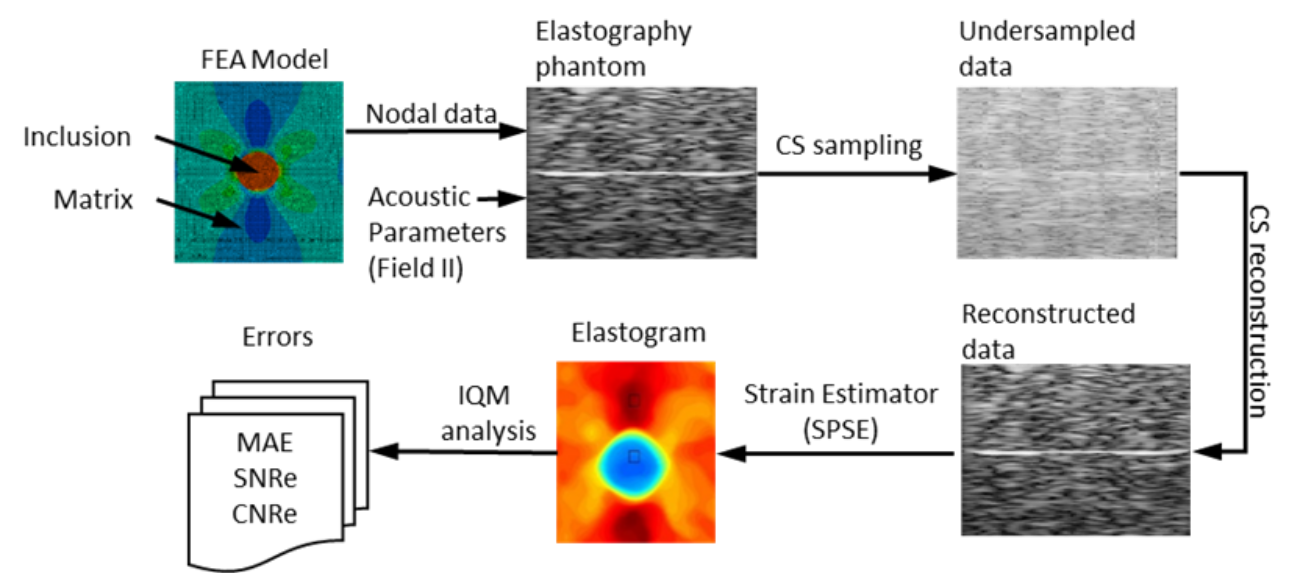
- Elastograms of elastography phantom computed from the original data and from various CS reconstruction frameworks for the subsampling rate of: (a) 30%, (b) 40%, and (c) 50%.
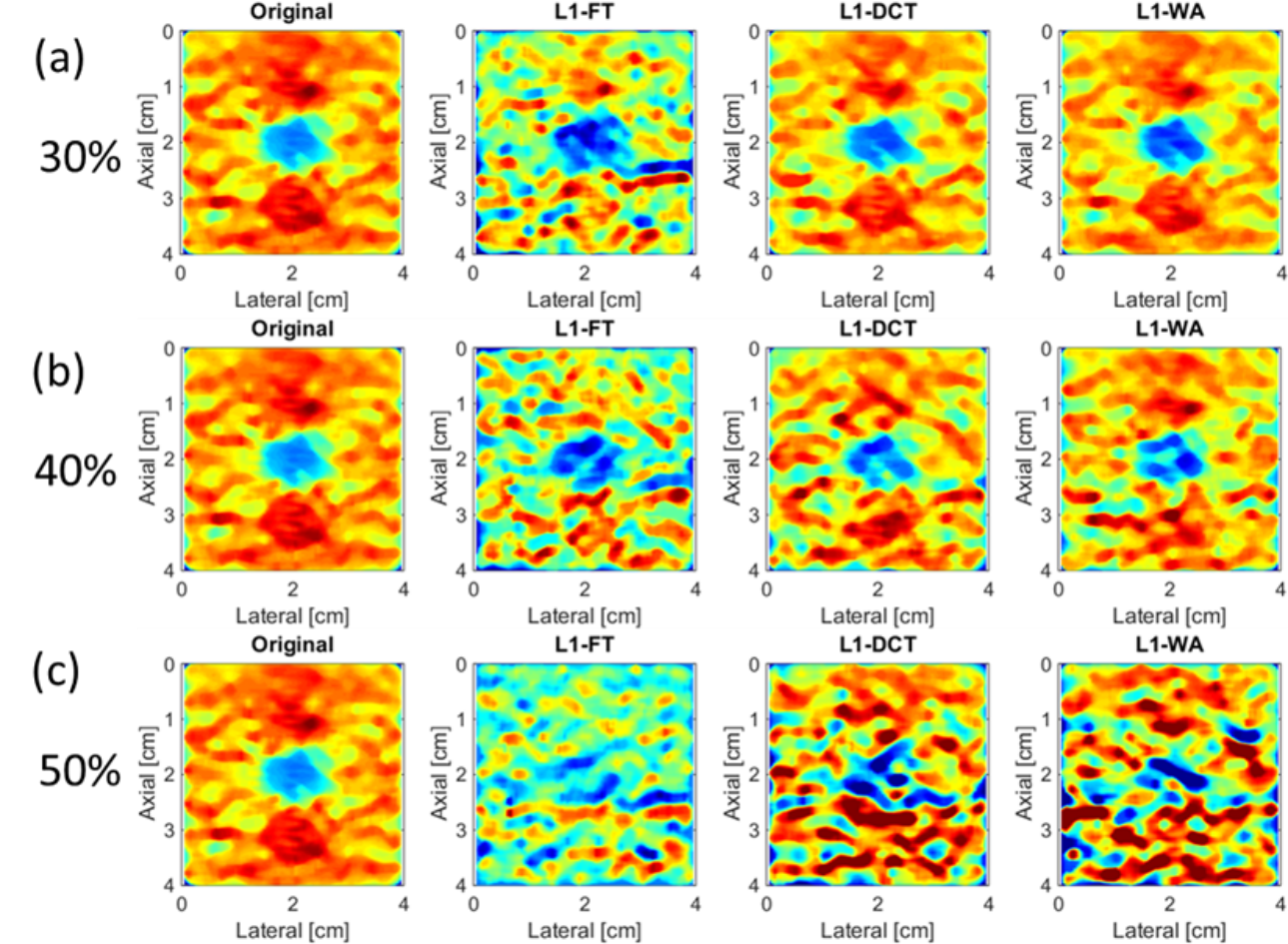
Related publication
-
Shin, B. ., Jeon, S. ., Ryu, J. ., & Kwon, H.-J. . (2017). Compressed Sensing for Elastography in Portable Ultrasound. Ultrasonic Imaging, 39, 393\textendash413.
-
Open configuration options
Shin, B. ., Jeon, S. ., Ryu, J. ., & Kwon, H.-J. . (2018). Elastography for portable ultrasound. Biomedical Engineering Letters, 8, 101\textendash116. Retrieved from https://doi.org/10.1007/s13534-017-0052-1