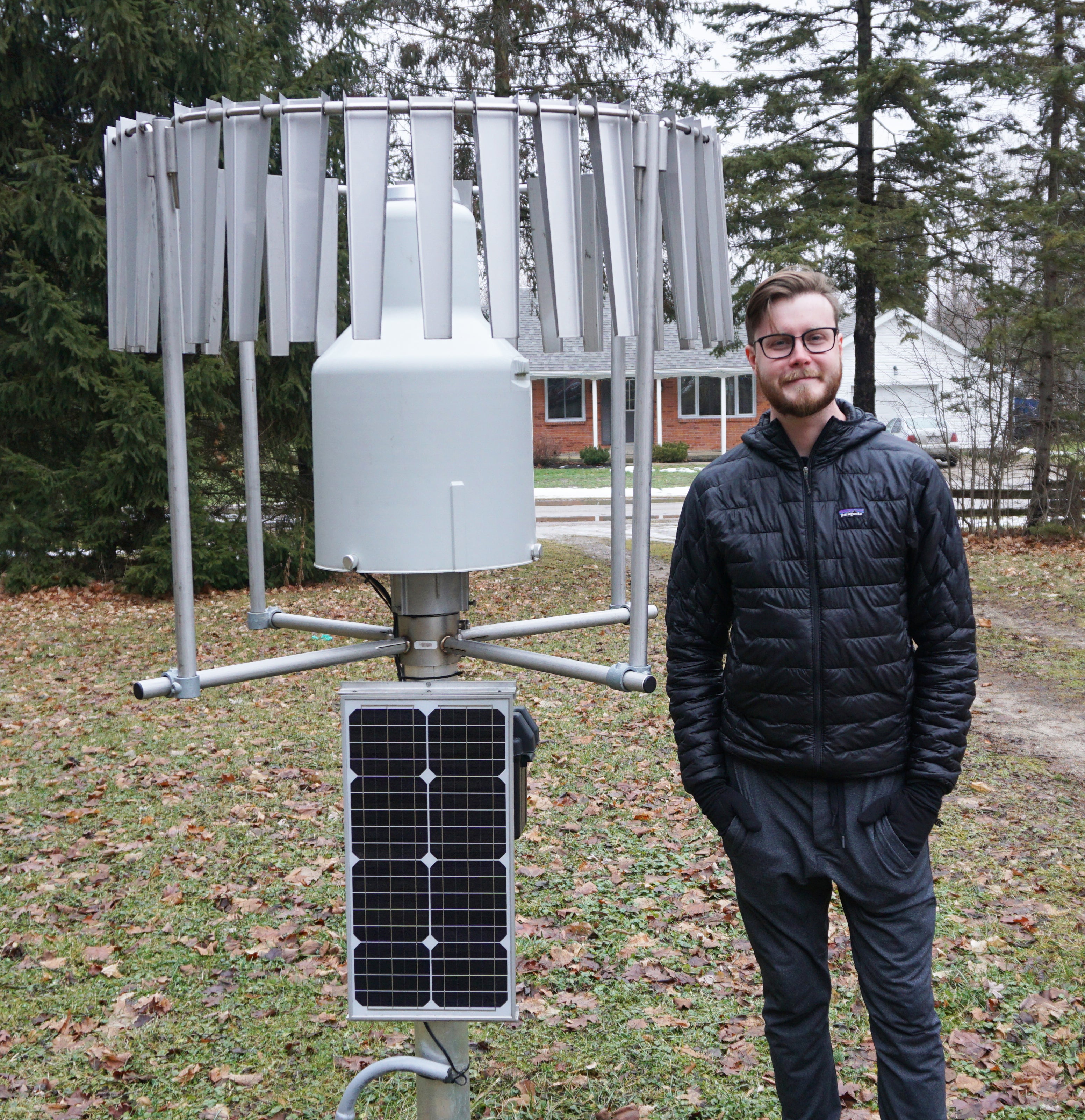
Hey!
I'm a 2017 Honours Computer Science, 2019 Master's of Science and 2022 PhD graduate from the University of Waterloo. My PhD program focus at the University of Waterloo was on machine learning, remote sensing and statistical modelling of precipitation. I also have experience as both full stack developer and iOS mobile developer from work done on personal projects as well as full time employment. My interests have always been in science, and it is this drive for understanding and innovation that sparked my career as a developer and my passion for design. Check out my blog for a detailed breakdown of my publications, presentation, side-projects, iOS apps and other hobbies. I also do statistics tutoring on the side.
A brief overview of my current research is illustrated below in my award-winning (1st place) 60 second animated gradFlicks video, along with my 3-Minute thesis presentation and 1st place video for the ESRI App Challenge 2019 and Jack Rosen memorial award for 2019.
For more information about me and the projects I'm invested in, please see my personal website: frasertheking.com
DeepPrecip
This image shows 1.7 million vertices and 2.8 million edges, which illustrates a snapshot in time of our deep learning neural network’s complex brain for calculating precipitation. As temperatures continue to rise, snowfall patterns are expected to change in complex ways with global impacts on springtime flooding, ecosystem development and water resource availability. High-accuracy precipitation models will allow us to better prepare for and mitigate against the consequences of a changing global climate. Each colour and cluster of vertices in this image represents a unique component of the neural network responsible for a specific task, and you can visualize how each of these pieces interact as neurons communicate across the network to predict rain and snow. Read more about this work in the follow blog posts: TowardsDataScience Graphcore uWaterloo
2020 Research poster (presented at the 2020 Canadian Space Agency Atmospheric Science from Space Workshop):
2021 International Summer Snow Workshop Poster:
EV1 Stairwell Environment Map (Canadian True Colour Composite):
www.mapsbyfraser.com for more information and other historic maps!
iLiDAR
Snow has significant impacts to springtime flooding, water resource management practices and the regional water-energy budget. In situ observations are considered some of the highest quality measurements of snow depth available, and are useful constraints for numerical weather prediction models and reanalysis system estimates of snow water equivalent. The application of laser altimetry (LiDAR-Light Detection and Ranging) for measuring snow depth has proven an effective method for quickly and accurately observing large areas, however this technique is expensive to perform in practice due to the high cost of the necessary equipment coupled with required operator training. In this work, we examine the capabilities of the iPhone 12 Pro LiDAR (iLiDAR) when attached to a consumer-grade DJI Phantom 4 quadcopter in estimating snow depth at three study sites in southern Ontario, Canada. Initial comparisons between drone iLiDAR depth estimates and collocated snow ruler measurements demonstrate good agreement, with root mean squared error < 2 mm, and absolute mean error of 2.5 cm. The intersection of these two technologies defines a novel, low-cost alternative to traditional LiDAR-based snow depth measurement systems, while maintaining a high level of accuracy and precision.
Research Videos:
2019 University of Waterloo GRADflix 1st Place Winner: Fraser King
Using CloudSat to Constrain Gridded SWE Products
Personal Weather Station (wunderground supporter):
Live updates every 5 seconds:
Previously advised by Dr. Christopher G. Fletcher (https://uwaterloo.ca/scholar/c5fletch/group_members)