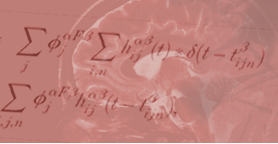
In short , theoretical neuroscientists are centrally interested in understanding the computational and mathematical principles behind brain function. Practically speaking, this research is important for both our understanding health-related issues and the development of future technologies. Already, a better understanding of neural systems has lead to important breakthroughs in treating brain-related illnesses. For instance, theoretical models allow us to understand and tailor medical interventions, such as the treatment of Parkinsonian tremor with deep-brain stimulation. Similarly, theoretical neuroscientists model the effects of various pharmacological interventions, improving our understanding of their precise effects (e.g., serotonin reuptake inhibitors used to treat depression and other anxiety disorders). Other health-related applications include brain-machine interface development, resulting in the construction of more effective prosthetics. New theoretical insights into neural coding have recently allowed monkeys to control robotic arms through direct neural implants.
In the context of information technologies, the insights gained from studying a successful, complex and behaviourly sophisticated system should prove ground-breaking for developing the next generation of intelligent machines. It has been clearly established that we do not have appropriate tools for engineering large, complex systems (for instance, only 30% of large software projects are successful). However, nature has solved such problems in ways we do not currently understand. The study of theoretical neuroscience provides new insights into nature’s solutions. In some areas (e.g., face recognition), neurally inspired algorithms are the state-of-the-art. However, there is much more we can learn regarding complex control, pattern recognition, learning, and dynamical systems from reverse-engineering the brain.
In
recognition
of
the
importance
of
theoretical
neuroscience,
significant
international
commitments
of
research
funding
have
recently
been
announced,
including
programs
from
the
European
Union
(Cognitive
Systems
Program,
$72
million/5
years),
the
United
States
(Defense
Advanced
Research
Projects
Agency
(DARPA)
(Biologically
Inspired
Cognitive
Architecture
Program),
National
Science
Foundation,
National
Institutes
for
Health
(NSF/NIH)
(Collaborative
Research
in
Computational
Neuroscience,
$6
million/year),
and
Japan
(RIKEN
Institute,
Creating
the
Brain
Division
$9
million/year).
Unfortunately,
Canada
does
not
have
similarly
targeted
funds,
although
Natural
Sciences
and
Engineering
Research
Council
of
Canada
(NSERC),
Canadian
Institutes
of
Health
Research
(CIHR),
and
Canadian
Foundation
of
Innovation
(CFI)
fund
research
in
the
area
through
their
general
programs.
As
a
result,
there
are
few
institutionalized
organizations
for
theoretical
neuroscience
in
Canada.