Citation:
Subramanian, S.G. et al., 2018. Decision Assist For Self-Driving Cars. In 31st Canadian Conference on Artificial Intelligence, Candian AI 2018. Toronto, Ontario, Canada: Springer, pp. 381-387. Available at: https://link.springer.com/chapter/10.1007%2F978-3-319-89656-4_44.
SelfDrivingAssist_3.pdf | 4.44 MB |
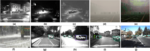
Abstract:
Research into self-driving cars has grown enormously in the last decade primarily due to the advances in the fields of machine intelligence and image processing. An under-appreciated aspect of self-driving cars is actively avoiding high traffic zones, low visibility zones, and routes with rough weather conditions by learning different conditions and making decisions based on trained experiences.
This paper addresses this challenge by introducing a novel hierarchical structure for dynamic path planning and experiential learning for vehicles.
A multistage system is proposed for detecting and compensating for weather, lighting, and traffic conditions as well as a novel adaptive path planning algorithm named \textbf{Checked State A3C}. This algorithm improves upon the existing A3C Reinforcement Learning (RL) algorithm by adding state memory which provides the ability to learn an adaptive model of the best decisions to take from experience.
This paper addresses this challenge by introducing a novel hierarchical structure for dynamic path planning and experiential learning for vehicles.
A multistage system is proposed for detecting and compensating for weather, lighting, and traffic conditions as well as a novel adaptive path planning algorithm named \textbf{Checked State A3C}. This algorithm improves upon the existing A3C Reinforcement Learning (RL) algorithm by adding state memory which provides the ability to learn an adaptive model of the best decisions to take from experience.