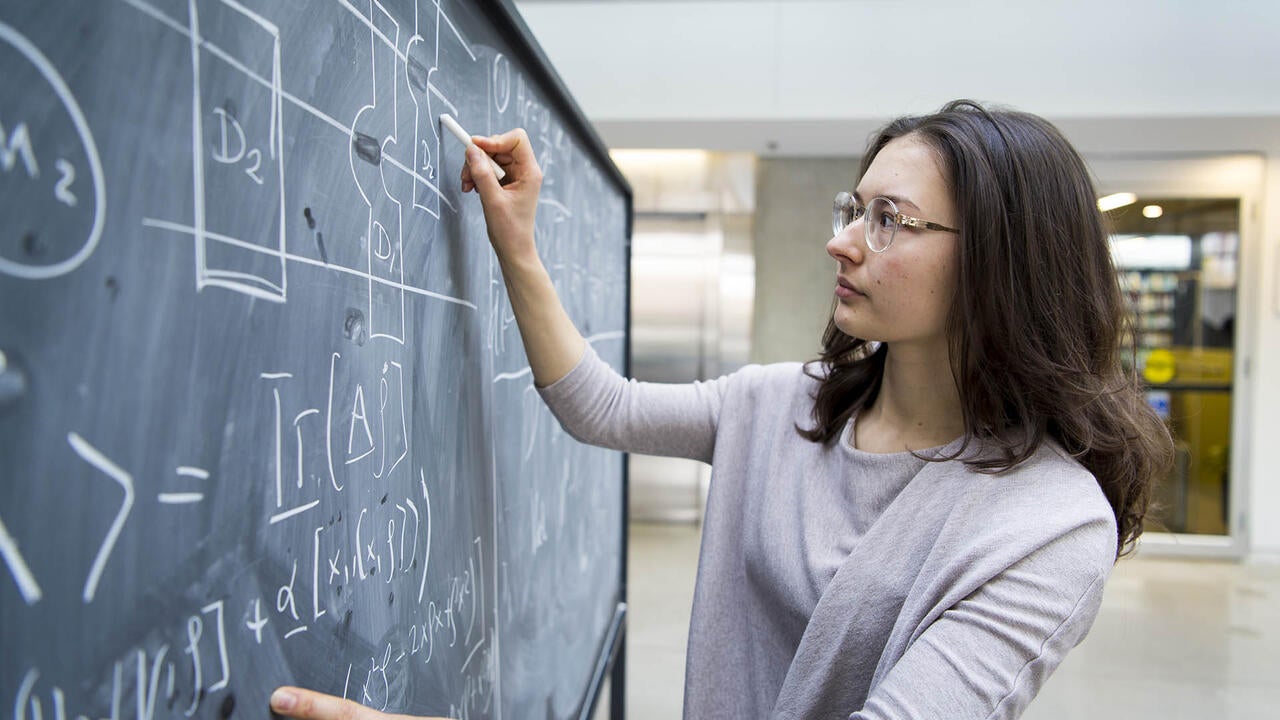
It’s time to wake up and do some physics
Rising star Anna Golubeva is using quantum theory to advance AI (and vice versa)
Rising star Anna Golubeva is using quantum theory to advance AI (and vice versa)
By Sam Toman University RelationsWhen University of Waterloo physicist Anna Golubeva got an email saying she’d won the NSERC Gilles Brassard Doctoral Prize for Interdisciplinary Research she thought it was an elaborate phishing scam.
“I just got this email saying I had won the award and a $10,000 prize. It looked real, but who knows right?” says Golubeva sitting in an ultra-modern breakout room in the Perimeter Institute for Theoretical Physics. “I didn’t believe it until I googled the woman’s name on the email and it all checked out.”
Golubeva shouldn’t have been that surprised. A year prior she was one of six Waterloo students who’d won a prestigious $50,000 Vanier Canada Graduate Scholarship for academic excellence, research potential, and leadership. While Gilles Brassard winners are selected from Vanier Scholars, the students have no idea if they’re even being considered until they win — hence Golubeva’s suspicion.
She should probably get used to it. The Waterloo PhD candidate is steadily establishing herself as one of the top young physicists working in the world and the problems she is helping solve are at the vanguard of both physics and machine learning.
Her work explores quantum many-body systems and precisely how interactions between microscopic particles lead to complex properties of the macroscopic system. To do this, Golubeva must also pioneer machine learning to handle the heavy computing necessary for untangling the web of information produced by these quantum many-body systems.
If you don’t totally understand what that means, it’s okay, the 29-year-old Golubeva is warm, personable, quick to laugh and clearly empathetic enough to intuit when a non-physicist can’t comprehend her work. Quickly she transitions to lay-person-speak.
“Imagine you have a picture of an apple and an orange,” she says. “You can teach AI to tell the difference between the two based on a bunch of examples.”
The conventional way to solve this task of distinguishing between apples and oranges would be to write a set of rules by hand. “We call it ‘hard-coding,’” she says.
“But even for a simple task like this there are too many exceptions to account for: differences in shape, color, other elements in the picture that one hasn’t accounted for, etc. The key difference between conventional programming and AI is precisely that AI does not require the human programmer to provide a set of rules — instead, it derives the rules itself, based on the examples that we present it. We call that ‘training data.’”
For a non-native English speaker, the Moldovan-born, Russian-speaking, German-raised 29-year-old scientist has a clear knack for making some of the most theoretical ideas sound approachable.
“Oranges are round, apples are differently shaped. Maybe an apple has a little leaf, or an orange has a rough skin… are you still with me?” Golubeva jokes.
Apples and orange are different. Got it.
An AI program begins learning how to differentiate between the two. With every pair of images it builds more and more training data it can use to determine more complicated or subtle differences. Gradually it gets better and better with each correct guess. But, as Golubeva says, “it does not disclose the rules it has learned — that’s the problem.”
The solution Golubeva is working towards is applying those machine learning methods to solve problems in complex quantum many-body systems. At the moment, her focus is on the development of a solid theoretical understanding of this machine learning, which would expand its applicability in physics. This in turn will also improve the power of the very machine learning techniques she is employing.
“It’s like a closed loop of learning,” Golubeva says.
There is an apocryphal quotation often attributed to 19th century British physicist Michael Faraday. After Faraday was made a fellow of the Royal Society the prime minister of the day asked what good his science theory could yield, and Faraday answered: “Why, Prime Minister, someday you can tax it.”
Golubeva isn’t nearly that glib, but as someone working on foundational science it’s clear she gets asked this question often. She knowingly and effortlessly un-cans a practiced and satisfactory answer.
“I suppose the problems I am working on could perhaps one day be used in finance,” she says. “Already they can use artificial intelligence to make successful stock market transactions, but these trades aren’t trusted because nobody knows how the AI makes those decisions — even if they’re correct. Without this validation it cannot be used commercially.”
As patient as Golubeva is with questions “impact,” and “real world uses,” they clearly don’t interest her much.
As a science-oriented high school student in the northern German city of Hanover she once thought she’d “go into business or something.” But after a rare (by German standards) clerical error landed her in an advanced physics class by accident, she’s gradually ditched the most applied elements of her science scholarship in favour of the ever-more theoretical.
“Anna is one of very few bold young researchers charting the boundaries between artificial intelligence and fundamental theoretical physics,” says Roger Melko a researcher with department of Physics and Astronomy at Waterloo and Perimeter Institute associate faculty member. “She represents the new generation of scientist exploring some of the most important foundational questions in machine learning. I am personally excited to have a front-row seat to the exciting developments coming out of Anna's new interdisciplinary field of research.”
Despite the excitement and beyond the awards Golubeva seems more than anything to be a young person enjoying being in an environment where she’s making a legitimate contribution to solving the grand puzzle of the universe.
What’s next for the young multiple-award-winning physicist? “In the morning I’ll wake up and then decide when it’s time to do some physics.”
Read more
Waterloo researcher David Cory shares three rules for building a quantum computer
Read more
University of Waterloo will play important role with applied AI solutions for everything from healthcare to finance and transportation
Read more
Track record of funding success speaks to University’s research strength
The University of Waterloo acknowledges that much of our work takes place on the traditional territory of the Neutral, Anishinaabeg, and Haudenosaunee peoples. Our main campus is situated on the Haldimand Tract, the land granted to the Six Nations that includes six miles on each side of the Grand River. Our active work toward reconciliation takes place across our campuses through research, learning, teaching, and community building, and is co-ordinated within the Office of Indigenous Relations.