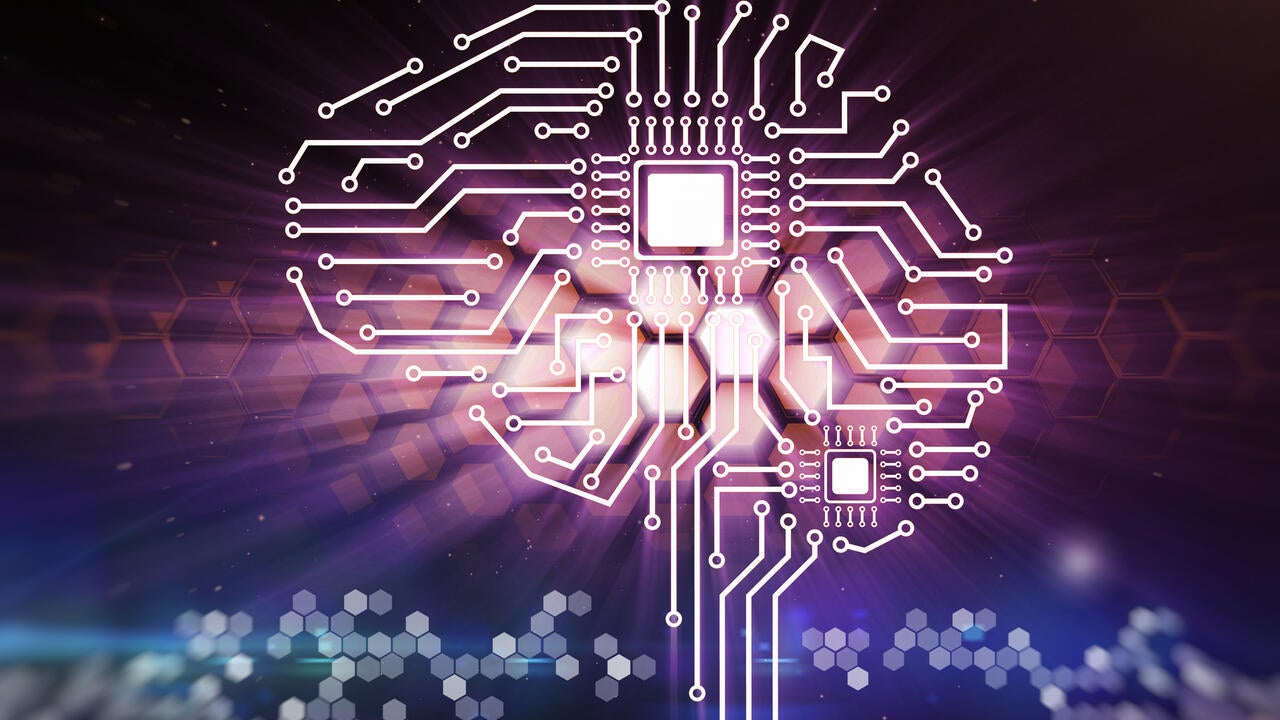
Reading the minds of deep learning AI systems
Waterloo researchers are analyzing how the complex computer programs that will drive our cars and help doctors diagnose illness — actually learn
Waterloo researchers are analyzing how the complex computer programs that will drive our cars and help doctors diagnose illness — actually learn
By Brian Caldwell Faculty of EngineeringAs expectations soar in the exploding field of artificial intelligence (AI), a small but growing group of researchers is buckling down on a fundamental problem: understanding how increasingly complex computer programs actually work.
One of those researchers is Devinder Kumar, a doctoral candidate in systems design engineering at the University of Waterloo who gave a keynote address on his work recently at the prestigious AI Toronto conference.
Together with colleagues at the Vision and Image Processing (VIP) Lab, Kumar is developing software technology called CLEAR (for ‘class-enhanced attentive response’) that would track backwards from the decisions made by deep-learning AI systems to analyze and ultimately explain them.
That is crucial information, largely ignored until the last few years, if powerful but so far inscrutable machines are to receive regulatory approvals and gain acceptance from users, particularly in sensitive areas such as medicine, finance and self-driving cars.
“If you don’t know how it works, you can’t have confidence in it,” says Kumar, who is supervised by Waterloo systems design engineering Professor Alexander Wong and Graham Taylor, an engineering professor at the University of Guelph.
The problem stems from the fact that deep-learning software, enabled by relatively recent growth in computational resources, essentially teaches itself by processing and identifying patterns in vast amounts of data.
Instead of being specifically told all the physical characteristics of cats, for instance, AI recognition systems are shown millions of images until they learn to identify them on their own.
That self-teaching ability makes such systems extremely powerful. The catch is that even their creators don’t know exactly how or what they have learned, such as which features they use to identify the cat in a given image.
Kumar’s work on this ‘explainability problem,’ as it is called, comes after previous research on deep-learning discovery radiomics software to detect lung cancer using CT scans, which involved collaborators at the Sunnybrook Health Sciences Centre in Toronto.
The potential benefits of that system include earlier detection and diagnosis without invasive biopsies, but to win approval for use in hospitals, it would need to be able to tell professionals why it decided a particular scan did or didn’t show cancer.
“If you’re a doctor using it, you can’t put your job on the line just because AI tells you something without knowing how it works,” Kumar says. “If something goes wrong, you are responsible.”
Understanding how deep-learning systems make their decisions, especially flawed decisions, would also allow programmers to improve their accuracy by correcting mistakes and, possibly, reveal new knowledge, such as previously unknown biomarkers of a disease.
Explainability techniques being developed by Kumar and his colleagues at the VIP Lab utilize advanced mathematical functions to look through the layers and layers of artificial neurons in AI software to identify the key areas and key data relied on to make decisions.
Their work on reading the minds of machines dovetails with expertise and ongoing research at Waterloo in operational AI, a branch of AI focused on compacting deep neural networks until they’re small enough to be embedded in stand-alone devices.
That practical approach, which Kumar also covered in his Toronto speech, promises cheaper, highly efficient AI without the need for links to cloud-based computers to get specific jobs done.
Operational AI has tremendous potential, particularly in fields such as healthcare and autonomous vehicles where security is a concern, but the mysterious inner workings of deep-learning systems are a hurdle in the way of widespread deployment.
“Very, very few researchers are focusing on this problem right now, but there is increasing activity,” Kumar says. “It is the next stage in the progression of AI.”
Read more
3D printing technology is perfect fit with new Waterloo Institute for Sustainable Aeronautics
Read more
Waterloo team creates vision of a transformed world for architecture biennale
Read more
Waterloo launches virtual simulation to simplify and explore climate solutions
The University of Waterloo acknowledges that much of our work takes place on the traditional territory of the Neutral, Anishinaabeg, and Haudenosaunee peoples. Our main campus is situated on the Haldimand Tract, the land granted to the Six Nations that includes six miles on each side of the Grand River. Our active work toward reconciliation takes place across our campuses through research, learning, teaching, and community building, and is co-ordinated within the Office of Indigenous Relations.