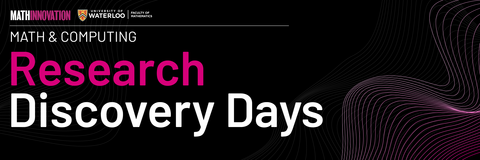
The Math & Computing Research Discovery Days (RDD) features thematic presentations from the Faculty of Mathematics. Math Innovation welcomes you to join us to learn about where researchers, students, and industry partners can engage in discussion. This event hosts keynote speakers who will discuss their research and its impacts. Faculty, student, and industry engagement through mathematical research innovation and entrepreneurship is at the forefront of this initiative.
RDD features world-leading keynote speakers, research spotlights, panel discussion, and poster sessions. The event is open to graduate students, faculty, entrepreneurs, start-ups, government, sponsors, and industry partners.
Join us to:
- Learn about leading-edge math research and applications from world-class researchers;
- Meet talented graduate students and be inspired by their passion for mathematics and its ability to change the world;
- Connect and share insights with thought leaders including industry experts, government policymakers, technical experts, and an ever-growing family of successful entrepreneurs with roots in the Faculty of Mathematics;
- Have fun with the math community during socials and more!
We look forward to seeing you in the Fall 2024!
Previous Events
April 2023 Research Themes and Speakers
Health and Medicine
The Faculty of Mathematics is poised to support the University of Waterloo’s long-term planning for Health Futures, as presented in the ‘Waterloo at 100’ vision. The institution aims to be a national and global leader at the interface of health, society, and technology. The Faculty of Mathematics is home to several prominent researchers studying topics at this interface:
- Mathematical modeling to better understand aspects of health and disease, including cancer treatment, kidney physiology, diabetes, antibiotic resistance, hypertension, epilepsy, hydrocephalus and syringomyelia, as well as modelling of public health and One Health processes
- Development and application of statistical methods for public health: analysis of life history data, longitudinal data, incomplete data, sequential methods, multivariate analysis, clinical trial design, and the assessment of diagnostic tests
- Computational health informatics: computational mechanisms for enabling more efficient and easy-to-use healthcare delivery, including large-scale data analytics problems in healthcare
- Mathematical biology: understanding cellular and biomolecular networks driving gene expression, signal transduction, and metabolism
Anita Layton
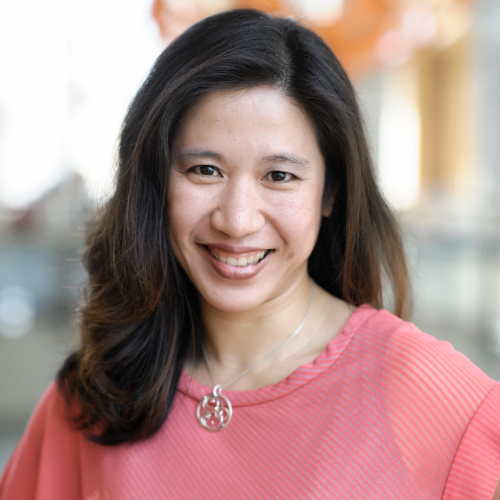
Health and Medicine Keynote: Anita Layton
Anita Layton is the Canada 150 Research Chair in Mathematical Biology and Medicine, and Professor of Applied Mathematics, Computer Science, Pharmacy and Biology at the University of Waterloo. She leads a diverse and interdisciplinary team of researchers who use computational modeling tools to better understand aspects of health and disease. Mathematics is their microscope! The Layton group collaborate with physiologists, biomedical engineers, and clinicians to formulate detailed models of cellular and organ function. Model simulations and predictions are used to answer questions such as: How should anti-hypertensive drugs be prescribed differently for men and women? Is it better to take one's medication in the morning or at night (or does it matter), given the interactions between the drug and our body's circadian rhythms?
Professor Layton is a Fellow of the Association for Women in Mathematics (2022), winner of the Krieger-Nelson Prize (2021), and winner of Canada’s Most Powerful Women: Top 100 Award (2021). She is an Associate Editor of SIAM Review Book Section, an Associate Editor of SIAM Journal on Applied Dynamical Systems, a Section Editor (AI/Machine Learning) of Hypertension, and an Associate Editor of Maple Transactions. Additionally, she serves as the Associate Dean, Research and International, for the Faculty of Mathematics, and chairs the Research Equity, Diversity and Inclusion Council at the University of Waterloo.
Yue Lyu
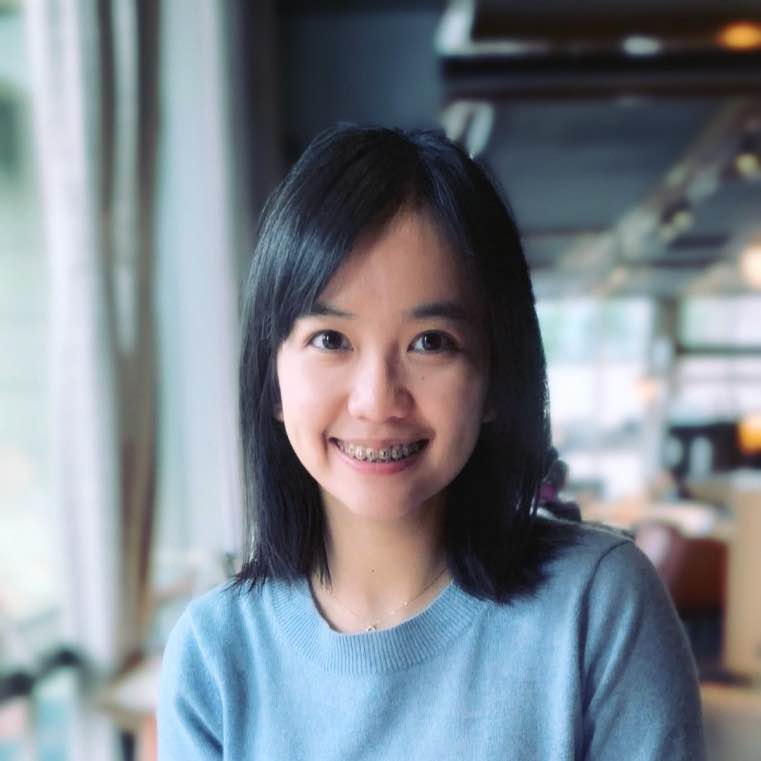
Student Research Spotlight: Yue Lyu
Yue Lyu is a Master’s student in Human-Computer Interaction at the WatVis Lab in the School of Computer Science, University of Waterloo, under the supervision of Drs. Jian Zhao and Keiko Katsuragawa. Her research focuses on building new user experiences and systems for autistic children by using advanced computer technologies to offer various knowledge and skills presentations as well as create opportunities for children to apply the skills in both the digital and physical worlds. Leveraging her background in computer science and business administration, her work aims to create solutions that are not only innovative but also practical and beneficial to the community. Yue has extensive experience in system design, database design, machine learning engineering, and mobile application development with multiple programming languages and platforms.
Security and Privacy
The Faculty of Mathematics at the University of Waterloo is world-renowned for its research excellence in data science, with faculty members and students exploring foundational and emerging questions in cryptography, privacy, security, machine learning, and the quantum environment. Examples of recent work in this area include:
- Security and privacy in machine learning: Analyzing and preventing the leakage of private information from machine learning models, enabling provenance verification of machine learning models, analyzing model theft and security-relevant failure modes of machine learning models
- Traffic classification in an increasingly encrypted web: Service detection, classification, and analysis from encrypted communication using Machine Learning
- Methods and tools for mapping and optimizing the implementation of quantum circuits to realistic architectures
Michele Mosca
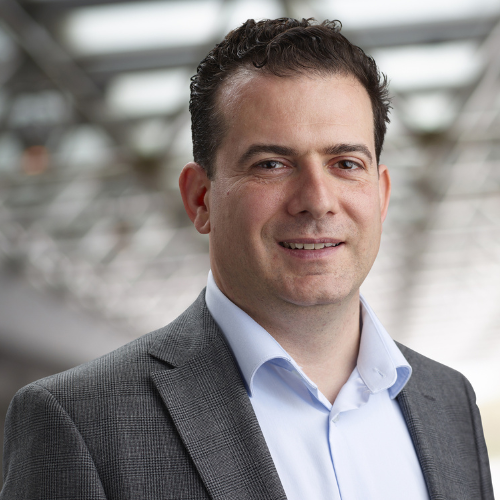
Security and Privacy Keynote: Michele Mosca
Michele Mosca is co-founder of the Institute for Quantum Computing at the University of Waterloo, a Professor in the Department of Combinatorics & Optimization of the Faculty of Mathematics, and a founding member of Waterloo's Perimeter Institute for Theoretical Physics. He is co-founder and CEO of the quantum-safe cybersecurity company, evolutionQ, and co-founder of the quantum software and applications company, softwareQ. He serves as Chair of the board of Quantum Industry Canada.
He started working in cryptography during his undergraduate studies and obtained his doctorate in Mathematics in 1999 from the University of Oxford on the topic of Quantum Computer Algorithms. His research interests include algorithms and software for quantum computers, and cryptographic tools designed to be safe against quantum technologies.
He co-founded the not-for-profit Quantum-Safe Canada, and the ETSI-IQC workshop series in quantum-safe cryptography and is globally recognized for his drive to help academia, industry and government prepare our cyber systems to be safe in an era with quantum computers.
Dr. Mosca’s awards and honours include 2010 Canada's Top 40 Under 40, Queen Elizabeth II Diamond Jubilee Medal (2013), SJU Fr. Norm Choate Lifetime Achievement Award (2017), and a Knighthood (Cavaliere) in the Order of Merit of the Italian Republic (2018).
Elham Akbari
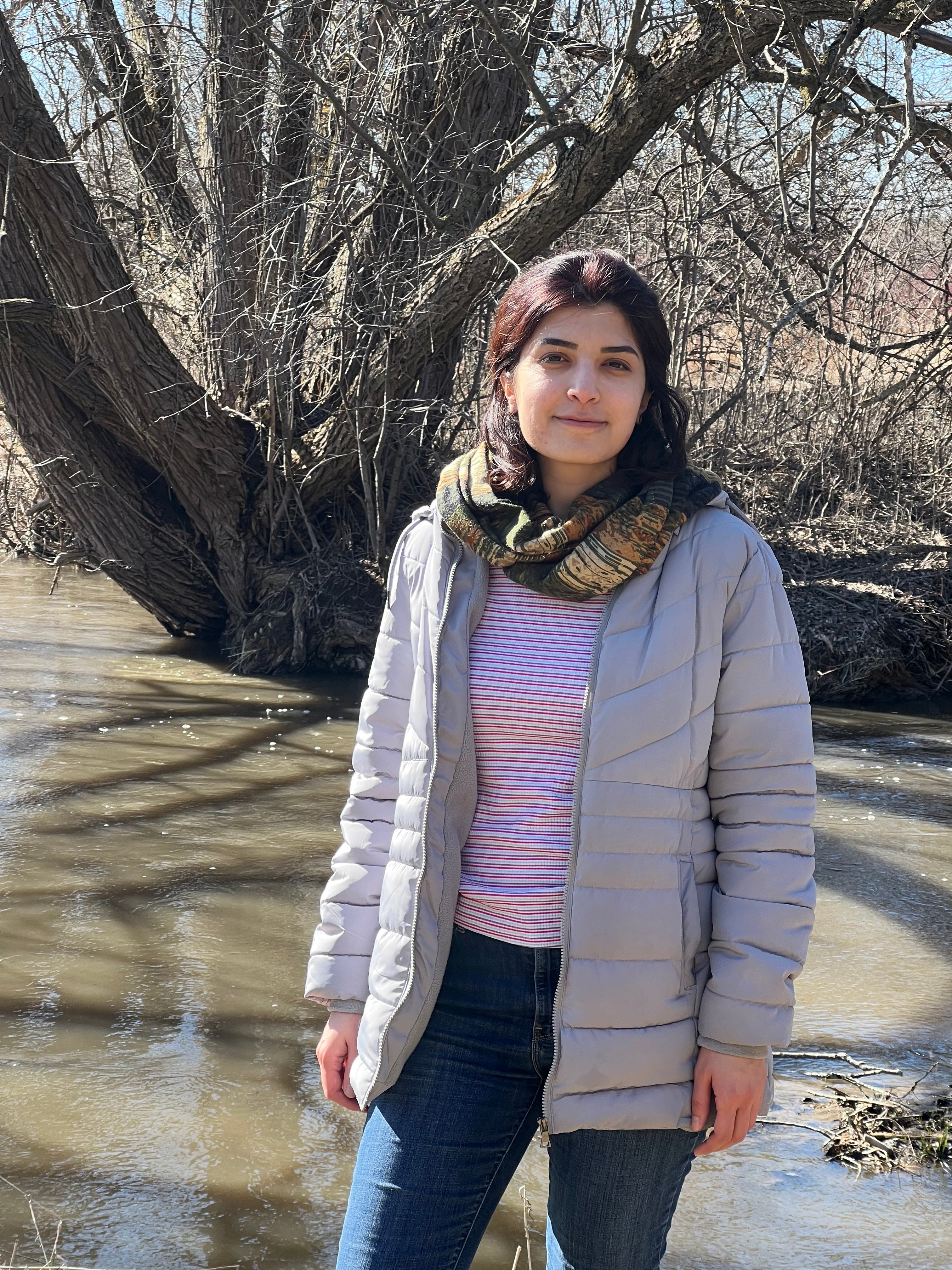
Student Research Spotlight: Elham Akbari
While consensus exists on the importance of encryption to ensure users’ privacy, encrypted traffic poses challenges for traffic classification; an indispensable first step for many network operations carried out by network service providers. A plethora of machine learning models, including deep learners, have been suggested in the literature for encrypted traffic classification, most of which assume access to a labeled training dataset and close training and test data distributions. In this talk, I will present the challenges that an operator will face while working with a model built under those assumptions. I will then present an alternative solution based on few-shot learning. We will see how few-shot learning reduces the number of labeled data samples required for classifying a new dataset but falls short of training a generalizable learner.
Data Integrity and Trust
In a world of ‘Big Data’, integrity and trust are critical aspects of data analytics and management for decision-making. With the data life cycle, data needs to be correct, truthful, complete, valid, and reliable.
- Leveraging the AI-people partnership: how to enhance and make sense of intelligent systems, addressing transparency, engagement, trust and collaboration
- Constrained optimization using interior point and trust region approaches analysis of theoretical algorithmic properties and development of efficient and robust software for application in financial services
- AI-powered meta-analyses: Building systems using AI and neural networks to help users gain insights from large amounts of varied data types
Ihab Ilyas
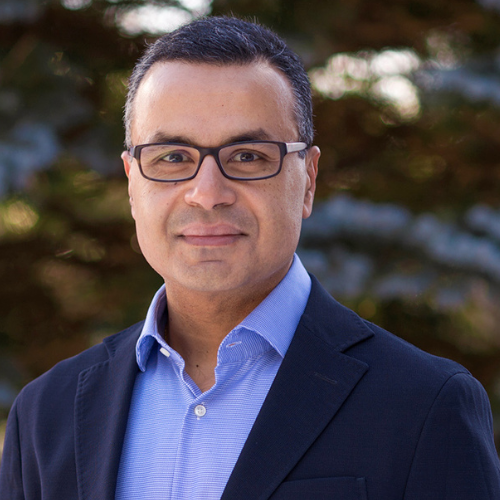
Data Integrity and Trust Keynote: Ihab Ilyas
Ihab F. Ilyas is a professor at Cheriton School of Computer Science at the University of Waterloo, and he is NSERC-Thomson Reuters Research Chair on data quality. Professor Ilyas’ main research interest lies in database systems, with focus on probabilistic and uncertain data management, machine learning for data quality and enrichment, and information extraction. He co-founded Tamr Inc. in 2013, a startup focusing on large-scale data integration and data cleaning. He also co-founded Inductiv in 2019 (acquired by Apple), a Waterloo-based startup using AI for structured data cleaning. Professor Ilyas’s research is premised on the belief that poor data quality is a main hurdle to adoption of AI technologies, and machine learning can be leveraged to clean datasets and train effective models accurately and timely at scale, significantly reducing time and labour costs.
Professor Ilyas is an ACM Fellow and IEEE Fellow, a recipient of the Ontario Early Researcher Award, a Cheriton Faculty Fellowship, an NSERC Discovery Accelerator Award, and a Google Faculty Award. In 2014, Professor Ilyas was a winner of ACM Distinguished Scientist. Additionally, he was an elected member of the VLDB Endowment board of trustees (2016-2021), elected SIGMOD vice chair (2016-2021), an associate editor of the ACM Transactions of Database Systems (2014-2020), and an associate editor of Foundations of Database Systems. Professor Ilyas is currently on leave from the university, leading the Apple knowledge platform at Apple.
Aida Sheshbolouki
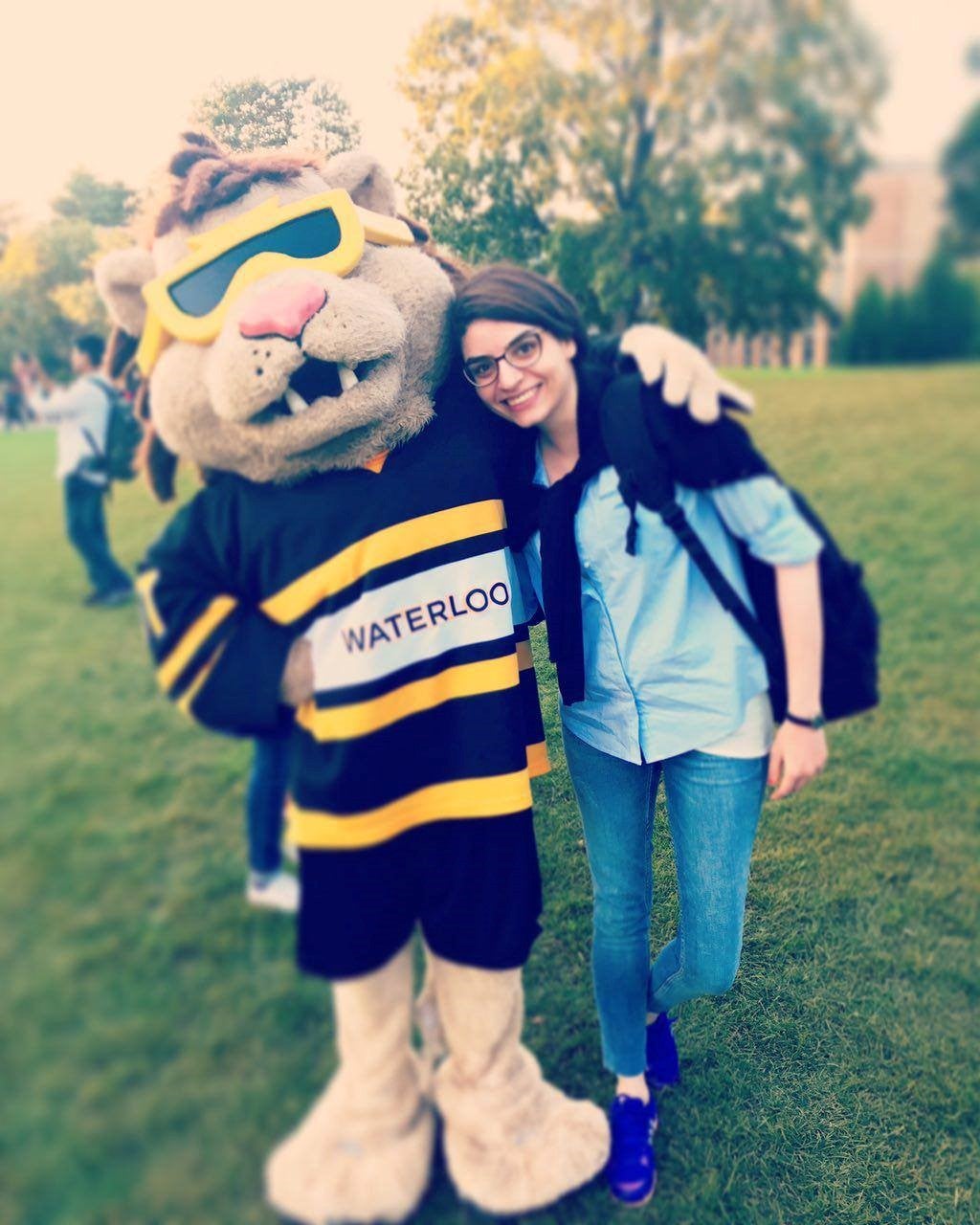
Student Research Spotlight: Aida Sheshbolouki
Aida Sheshbolouki is a Ph.D. candidate and a member of the Data Systems Group at the University of Waterloo under the supervision of Prof. M. Tamer Ozsu. Her research involves steps towards data-driven algorithm design for explainable and interpretable analytics over streaming graphs with a particular focus on (2,2)-bicliques (known as butterflies) in bipartite structures.
Founding Innovation
As the only dedicated Faculty of Mathematics in North America, our faculty make world-leading contributions to technology and innovation, while our students are trained and supported to become industry leaders and disrupters. The entrepreneurial spirit within the Faculty of Mathematics is strong, and many of our alumni have founded and exited successful businesses that translate foundational math into innovations for real-world application.
Steven Woods
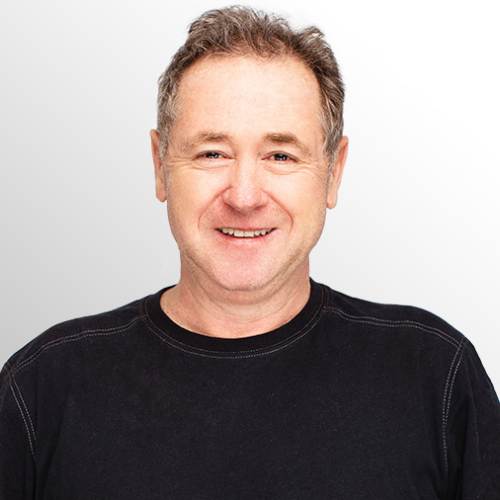
Founding Innovation Keynote: Steven Woods
Steven has worked in software development, research and technical leadership for more than 30 years. As co-founder of three software and services companies based in the USA (Quack.com, Kinitos, NeoEdge Networks), he built strong, high-performing teams of product and technical experts based in both Silicon Valley and the Waterloo-Toronto tech corridor. One of these companies - Quack.com - pioneered the world's first interactive voice assistant and was acquired by Netscape/America Online in 2000.
Steven holds a BSc in Computer Science from the University of Saskatchewan and a Masters and PhD in Computer Science (Mathematics) from the University of Waterloo. Among numerous awards, Steven received the prestigious JW Graham Medal in Computing and Innovation from the University of Waterloo in 2010 and was recognized with a Distinguished Lifetime Achievement Award from the University of Saskatchewan in 2021.
Enamul Haque
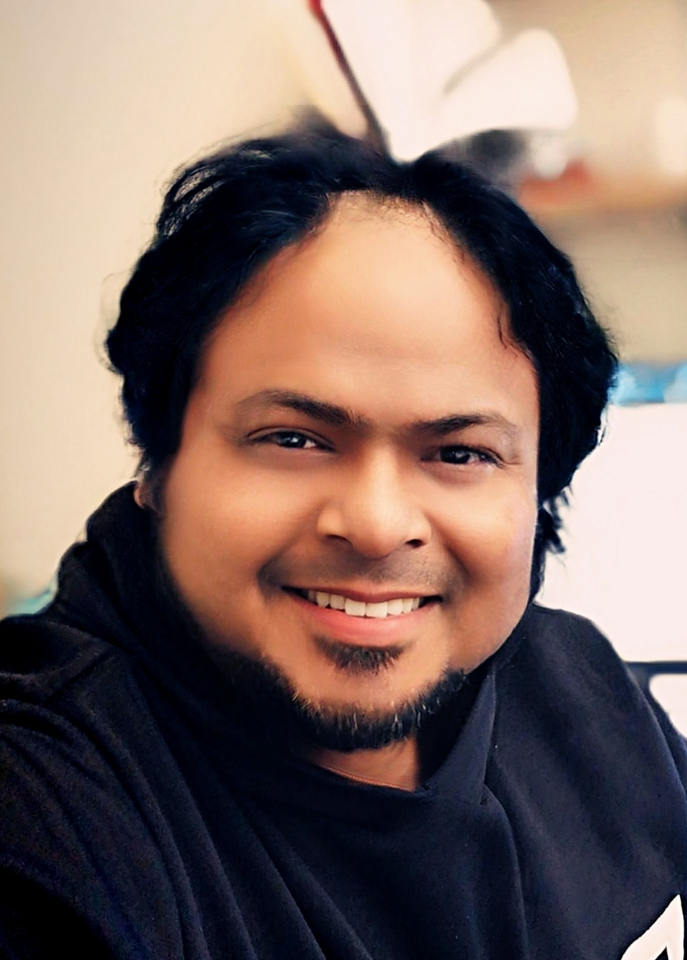
Research Abstract: Lightning Round
Reference in Structured Data Integration for Data Science
For any enterprise whether it is a business or research organization, making better decisions is extremely important for success but that requires handling huge amounts of data analytics from diverse sources which is again a big technical challenge. Data integration helps in this regard by bringing data from disparate sources together to provide users with a unified view where they can run queries easily to obtain the required information. In this research, we discuss semantic data modelling and reference issues in structured data integration. Our work will explore and establish the theoretical foundations for related data models, reference issues, query language grammar, semantics, and constructs for the integration mechanisms as well.
Enamul Haque's Biography
At the University of Waterloo (Data Systems Group), Enamul's research area falls broadly under AI, specifically at the cross-section of Knowledge Representation and Databases. He is supervised by Dr. Grant Weddell and Dr. David Toman. Before that, he completed his MSc. In Computer Science (Data Science Lab) under the supervision of Dr. Fei Chiang from McMaster University. At McMaster, his research topic was data cleaning from an ontological perspective. Also, he has an MBA in Management and Marketing from North South University and an undergrad degree in Computer Science and Engineering from Chittagong University of Engineering and Technology, and his undergrad research project was on Machine Learning.
Zitao He
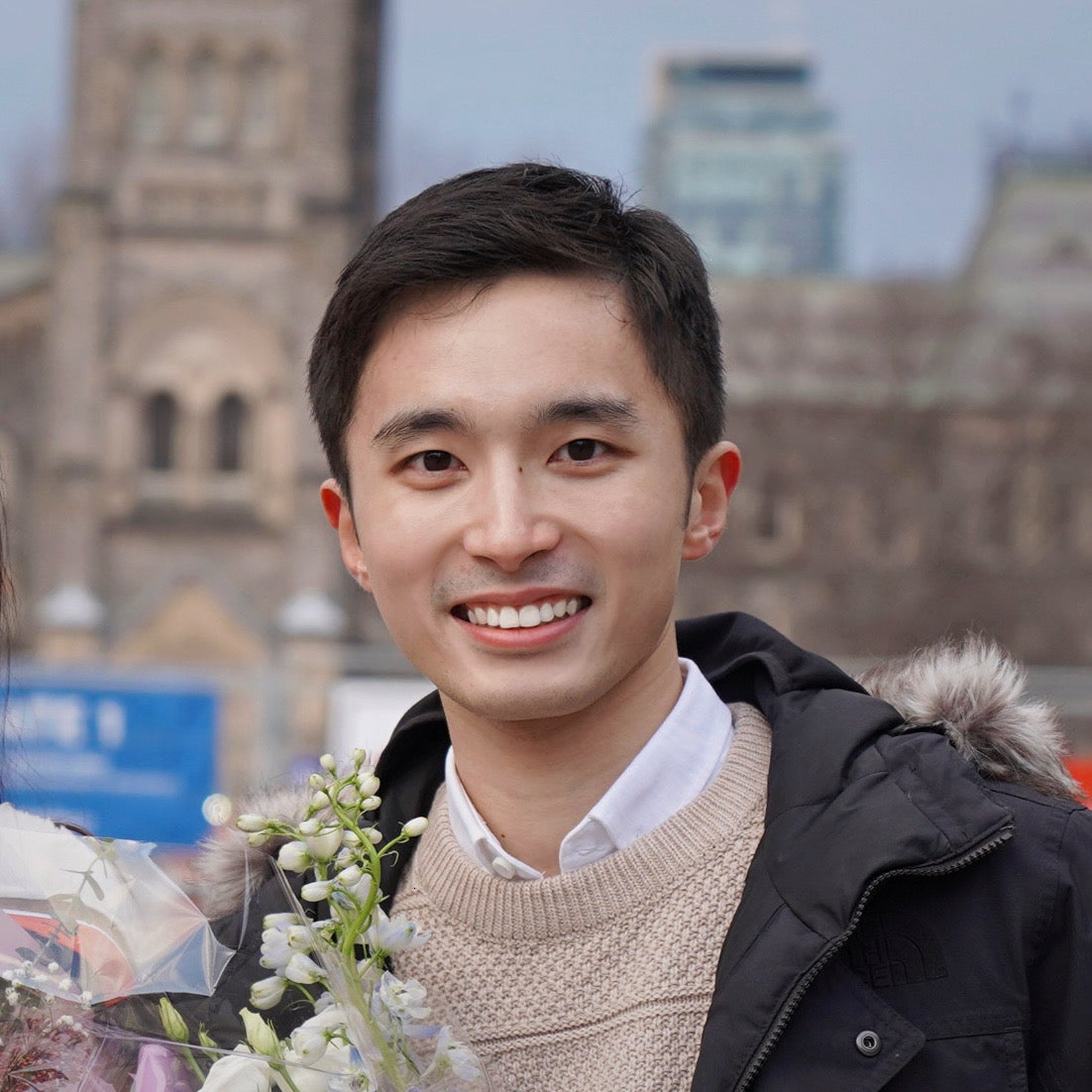
Research Abstract: Lightning Round
Predicting Measles Outbreaks from Vaccine Sentiments on Social Media
Vaccinating decisions for childhood diseases such as measles have been studied using coupled
models of disease dynamics and individual behaviors. Such models applied evolutionary game
theory to model people’s vaccination strategies as they are affected by social norms.
The popularity of social media has provided convenience for the spread of pro- and anti-vaccine
sentiments. High-frequency social media data also brings new ideas to study population dynamics
of infectious diseases.
This project aims to build a bridge directly from social media data to potential disease outbreaks
through deep learning models and provide novel methods for disease outbreak prediction
near thresholds. We improved existing coupled behavior-disease models by considering different
social groups and investigated the effect of homophily on disease dynamics near tipping points. We
generated realizations from our developed stochastic model. After being trained with enough theoretical
data, we showed that a deep learning model (e.g. a CNN-LSTM model) could capture EWS
directly from real-world social media data and predict a potential disease outbreak in the future.
Zitao He's Biography
Zitao He is a PhD candidate in Applied Mathematics at the University of Waterloo under the supervision of Dr. Chris Bauch. Zitao's research interest lies at the intersection of population dynamics in epidemiology, data-driven dynamical systems, and applications of evolutionary game theory. His current research focuses on critical slowing down in online social media and social networks in coupled behavior-disease systems. Specifically, he is investigating how vaccine strategy change in social networks affects the spread of infectious diseases, such as measles, and the possibility of using machine learning models to predict future disease prevalence from vaccine sentiments on social media.
Amandeep Kaur
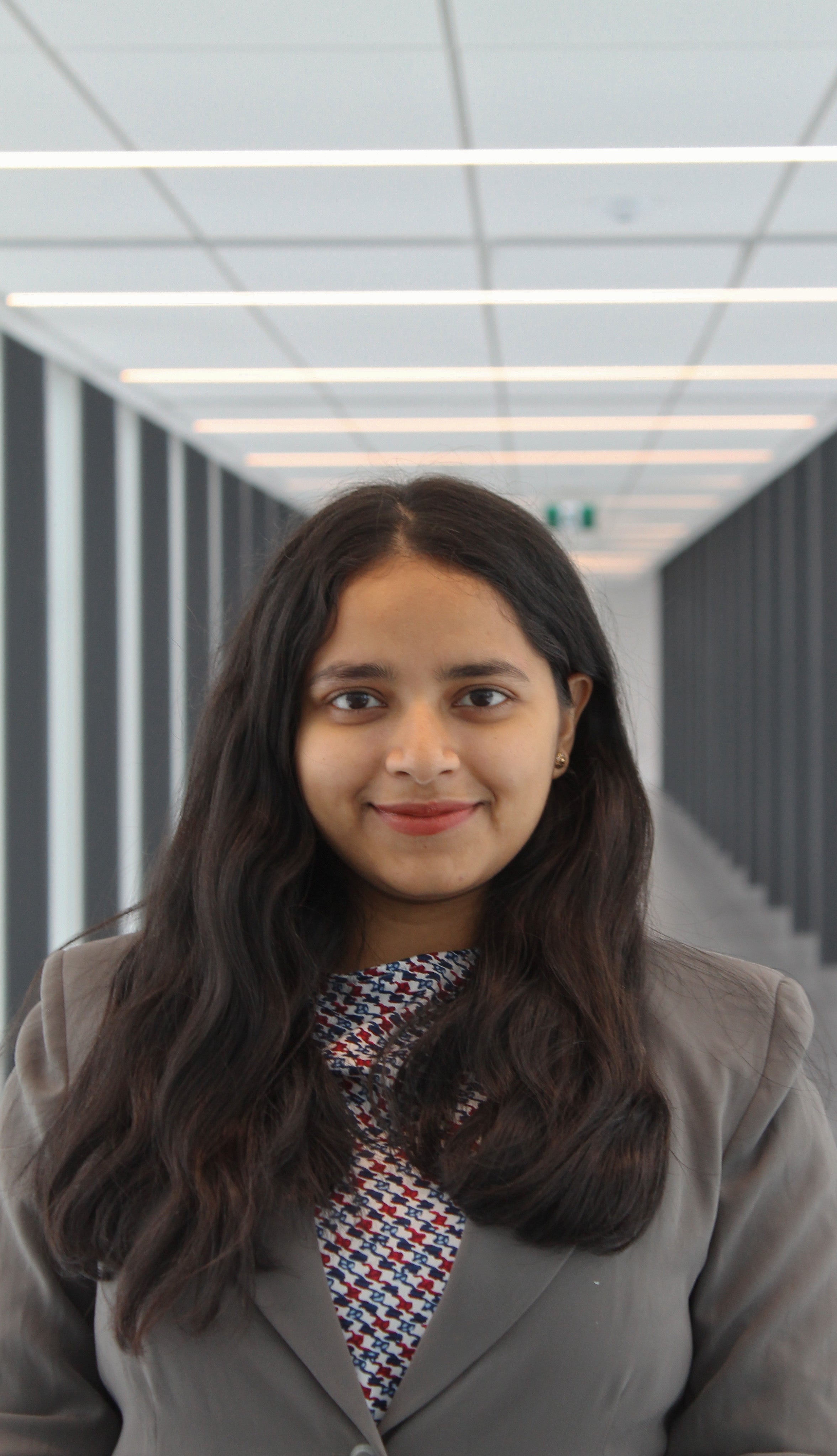
Research Abstract: Lightning Round
GradXcepUNet: Explainable AI Based Medical Image Segmentation
Medical images segmentation is an important research area. Physicians and radiologists can diagnose diseases in their patients by observing the visual features using various imaging methods like CT, MRI, X-ray, and Ultrasound. AI-based medical image segmentation models can help radiologists and experts analyze various ailments. However, the prediction results are only trustworthy when the results can be interpreted by a doctor. Our work utilizes “Explainable AI(XAI).” We propose GradXcepUNet, an XAI-based medical image segmentation model, that couples the segmentation power of U-Net and explainability features of the Xception classification network by Grad-CAM. The Grad-CAM trained images highlight the critical regions for the Xception classification network. Then, as the guidance, the visualized results for critical regions are combined with an existing segmentation model (U-Net) to produce the final segmentation results. With the assistance of XAI analysis and visualization, our GradXcepUNet outperforms the original U-Net and many state-of-the-art methods. The evaluation results show that we can reach a Dice coefficient of 97.73% and an Intersection over Union (IoU) score of 78.86% on the 3D-IRCADb-01 database.
Amandeep Kaur's Biography
Amandeep Kaur is a Master’s student in the Computational Physiology Team in the School of Computer Science, University of Waterloo, under the supervision of Prof. Anita Layton. Her research focuses on building domain adaptive models to improve healthcare outcomes for people using Artificial Intelligence that may help lower costs and increase efficiency and accuracy. Advancement in technology brings a massive and scalable change in community health outcomes. This idea of creating the most positive impact for the most number of people is what motivates, inspires, and propels her to pursue her passion for using computing technologies for the greater good.
She was a MITACS Globalink Research intern at the University of Alberta, Canada, under the guidance of Prof. Anup Basu, and an awardee of the MITACS Graduate Fellowship worth $15,000 to provide financial support for her master’s.
Melissa Stadt
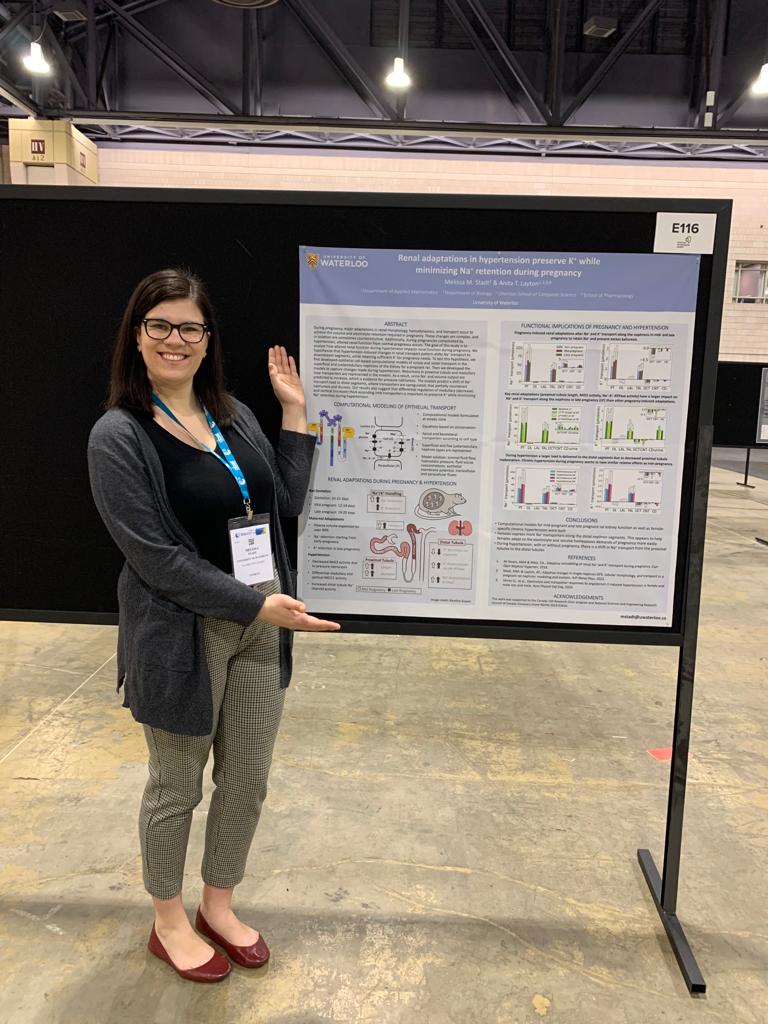
Research Abstract: Lightning Round
Impact of pregnancy and lactation on maternal calcium homeostasis: Mathematical model and analysis
During pregnancy and lactation there are significant changes in the maternal body. In fact, physiological adaptations occur in almost all tissues and organs. In particular, calcium homeostasis is impacted due to the large demand for calcium by the fetus and breastmilk. Despite having a similar additional calcium demand, maternal adaptations in pregnancy and lactation are different. During pregnancy, the mother’s body increases intestinal absorption of calcium. However, during lactation, intestinal absorption returns to normal levels and the calcium needs of breastmilk are instead met by increased bone resorption and calcium reabsorption from the kidneys. The multitude of physiological processes involved in calcium homeostasis along with the complex and dynamic adaptations involved in lactation and pregnancy make this problem well-suited for investigation using mathematical modeling. In this project, we explore the differential impacts of pregnancy and lactation on calcium demand in the mother’s body. Understanding these mechanisms are key for understanding the complicated physiologies of pregnancy and lactation.
Melissa Stadt's Biography
Melissa Stadt is a PhD student in applied mathematics under the supervision of Prof. Anita Layton. She also received her MMath degree from the University of Waterloo under Prof. Layton’s supervision. Her research focuses on using mathematical models to investigate problems in physiology related to women’s health. Currently, she is developing mathematical models of calcium homeostasis to study the effects of sex differences as well as the multi-organ adaptations that occur during pregnancy and lactation. These models can be used to unravel how maternal adaptations sustain the calcium additional demands of the fetus and milk in pregnancy and lactation, respectively.
Lena Podina
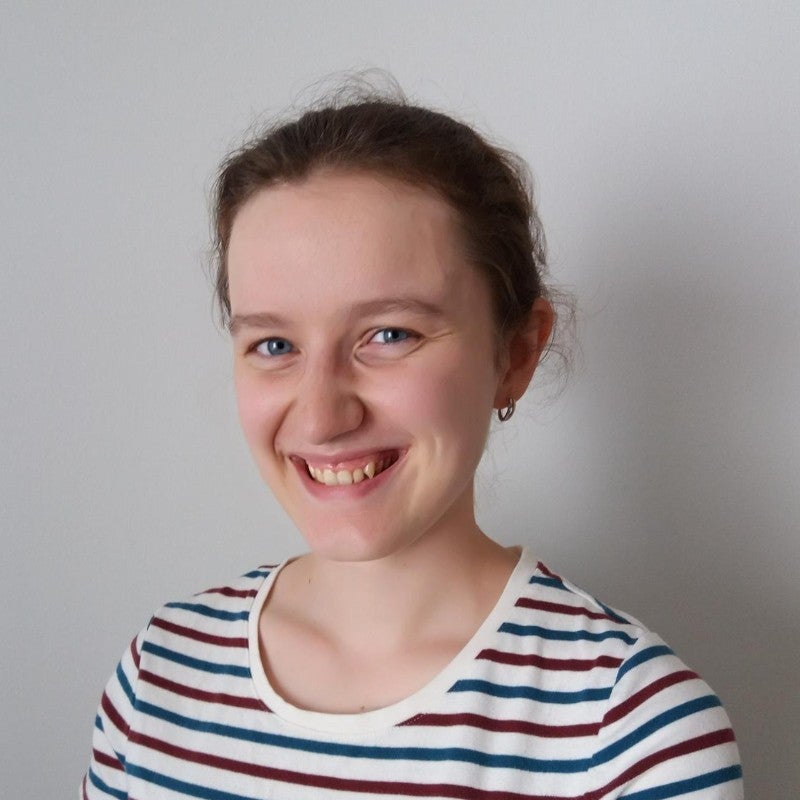
Research Abstract: Lightning Round
A PINN Approach to Symbolic Differential Operator Discovery with Sparse Data
Lena Podina, Brydon Eastman, Mohammad Kohandel
Given ample experimental data from a system governed by differential equations, it is possible to use deep learning techniques to construct the underlying differential operators.This small data regime in machine learning can be made tractable by providing our algorithms with prior information about the underlying dynamics. Physics Informed Neural Networks (PINNs) have been very successful in this regime (reconstructing entire ODE solutions using only a single point or entire PDE solutions with very few measurements of the initial condition). We modify the PINN approach by adding a neural network that learns a representation of unknown hidden terms in the differential equation. The algorithm yields both a surrogate solution to the differential equation and a black-box representation of the hidden terms. These hidden term neural networks can then be converted into symbolic equations using symbolic regression techniques like AI Feynman. In order to achieve convergence of these neural networks, we provide our algorithms with (noisy) measurements of both the initial condition as well as (synthetic) experimental data obtained at later times.
Lena Podina's Biography
Lena is completing her Master of Mathematics with a focus on machine learning applications in biology. She grew up in Vancouver and did her undergrad at UBC. She is a bird enthusiast, and in her free time, she enjoys reading, doing yoga, and ice skating.
Stéphanie Abo
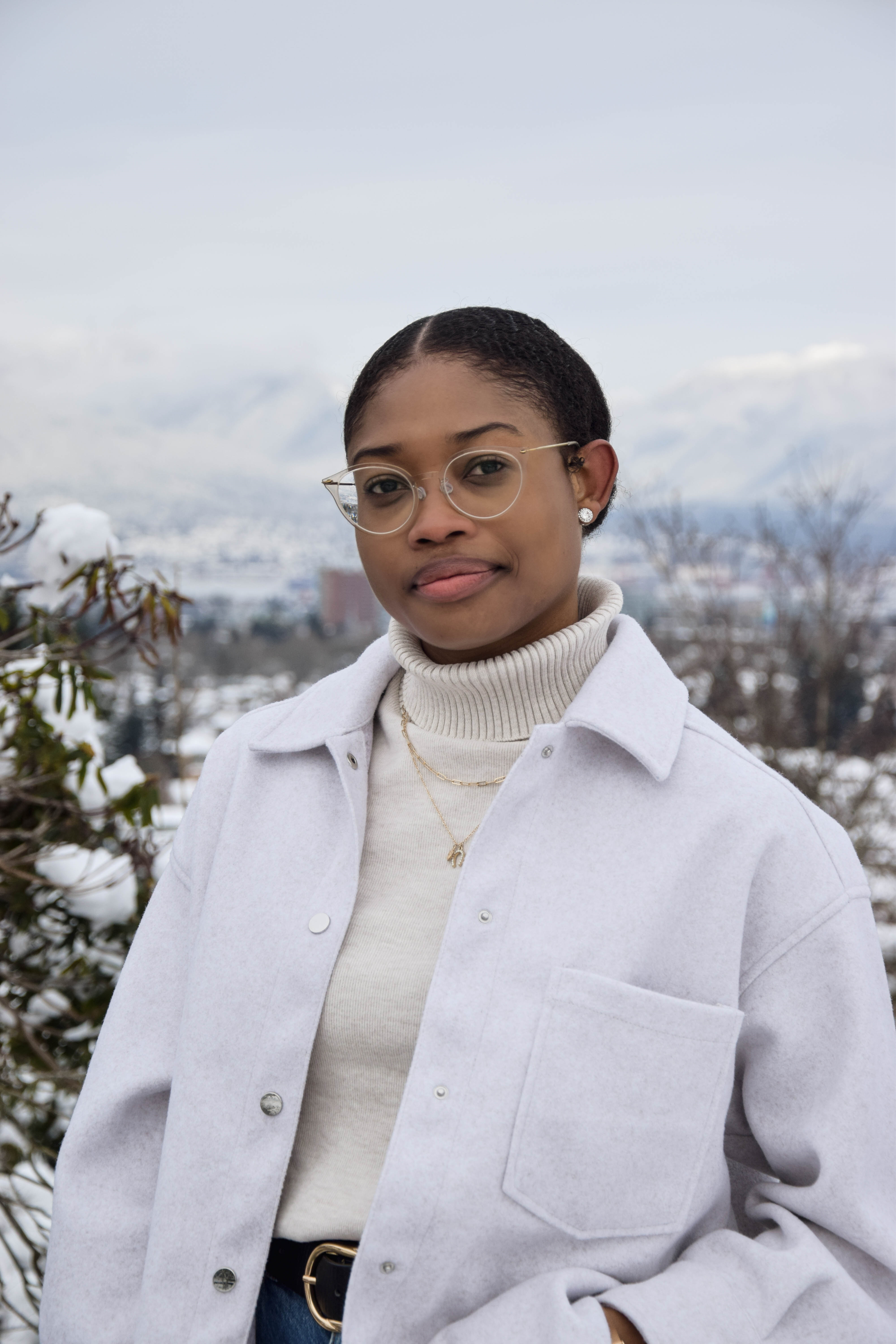
Research Abstract: Lightning Round
Investigating the role of circadian rhythm-based exercise and diet in type 2 diabetes
Justification of the importance of the work being presented
We develop mathematical models of metabolism and its interactions with our body's internal clock, also known as the circadian clock. Circadian rhythm disruption can contribute to metabolic dysregulation (diabetes, hypertension) and memory issues. Although the brain centrally controls circadian rhythms, peripheral tissues like the liver and muscles impose their own rhythms. It is therefore important to conduct research that directly compares exercise at various times versus meal ingestion. We present a whole-body exercise and diet model that incorporates controls from the liver and skeletal muscle peripheral clocks. Our model predicts that post-meal exercise improves glycemia in patients with type 2 diabetes mellitus (T2DM), and that the benefits of morning or afternoon exercise vary according to chronotype. The model could be used to guide future experimental studies aimed at determining the effect of circadian rhythms on the efficacy of exercise and diet for T2DM.
Stéphanie Abo's Biography
My name is Stéphanie Abo, and I'm a fourth-year PhD student in applied mathematics. My research is devoted to understanding biological clocks, also called circadian clocks. I specialize in the development of mathematical models of the immune system and metabolism and their interactions with our body's clock, both in healthy conditions and diseases like diabetes or immune infections. To understand biological timekeeping, I use techniques from a variety of fields, including computer simulations, detailed mathematical modeling, and mathematical analysis. My research aims to make predictions that can be verified experimentally.
Yuzhe You
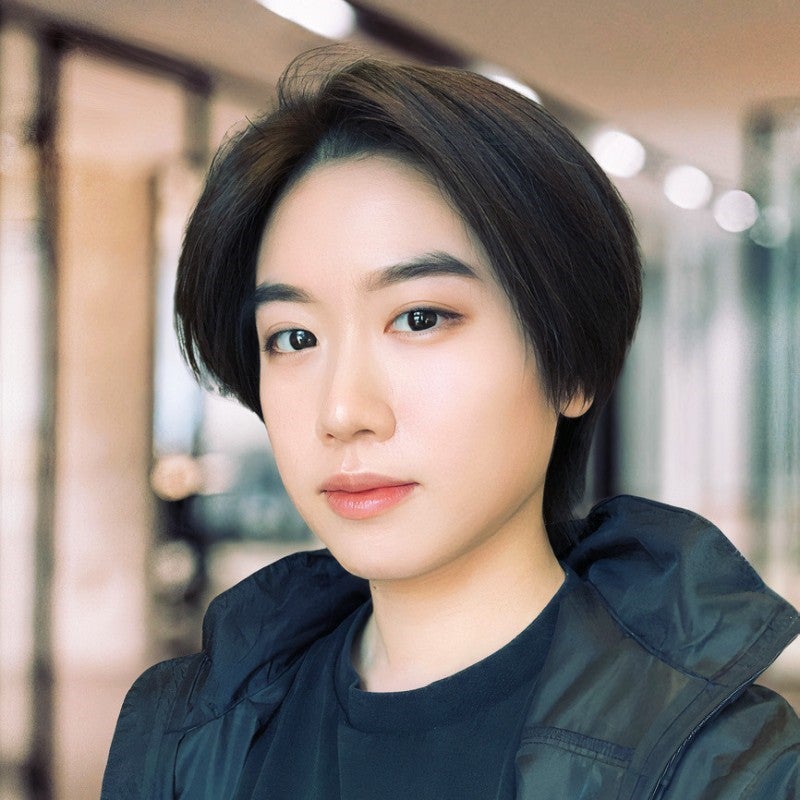
Research Abstract: Lightning Round
Adversarial machine learning focuses on studying attacks that target machine learning algorithms and the
defenses against worst-case attacks to strengthen the adversarial robustness of existing models. Specifically for image classification tasks, it is often difficult to comprehend the underlying logic behind adversarial examples even for machine learning practitioners due to key challenges such as 1) the attacks exploiting "non-robust" features that are not human-interpretable and 2) the perturbations applied being almost imperceptible to human eyes. In this study, we propose an interactive web-based application that aims to visualize the properties and consequences of evasion attacks for adversarial machine learning learners and provide data and model performance analytics for more experienced practitioners. In addition, we design the visualization to be informative in terms of robustness comparison evaluation between different models and show how prediction results may alter when varying levels of perturbations are applied to the data instances.
Yuzhe You's Biography
Yuzhe is a Master of Mathematics student from the Cheriton School of Computer Science, University of Waterloo. She is under the supervision of Dr. Jian Zhao and has a background in Information Visualization, Machine Learning, Transportation Analysis, Data Analytics & more. Yuzhe is a member of both WatVis research group and iTSS lab, where she conducts research in the area of InfoVis for AI Explainability with a focus on visualizing Adversarial Machine Learning and traffic analysis. Specifically, she designs interactive visualization tools that enhance AI interpretability & help improve the adversarial robustness of machine learning models.
Mackenzie Cameron
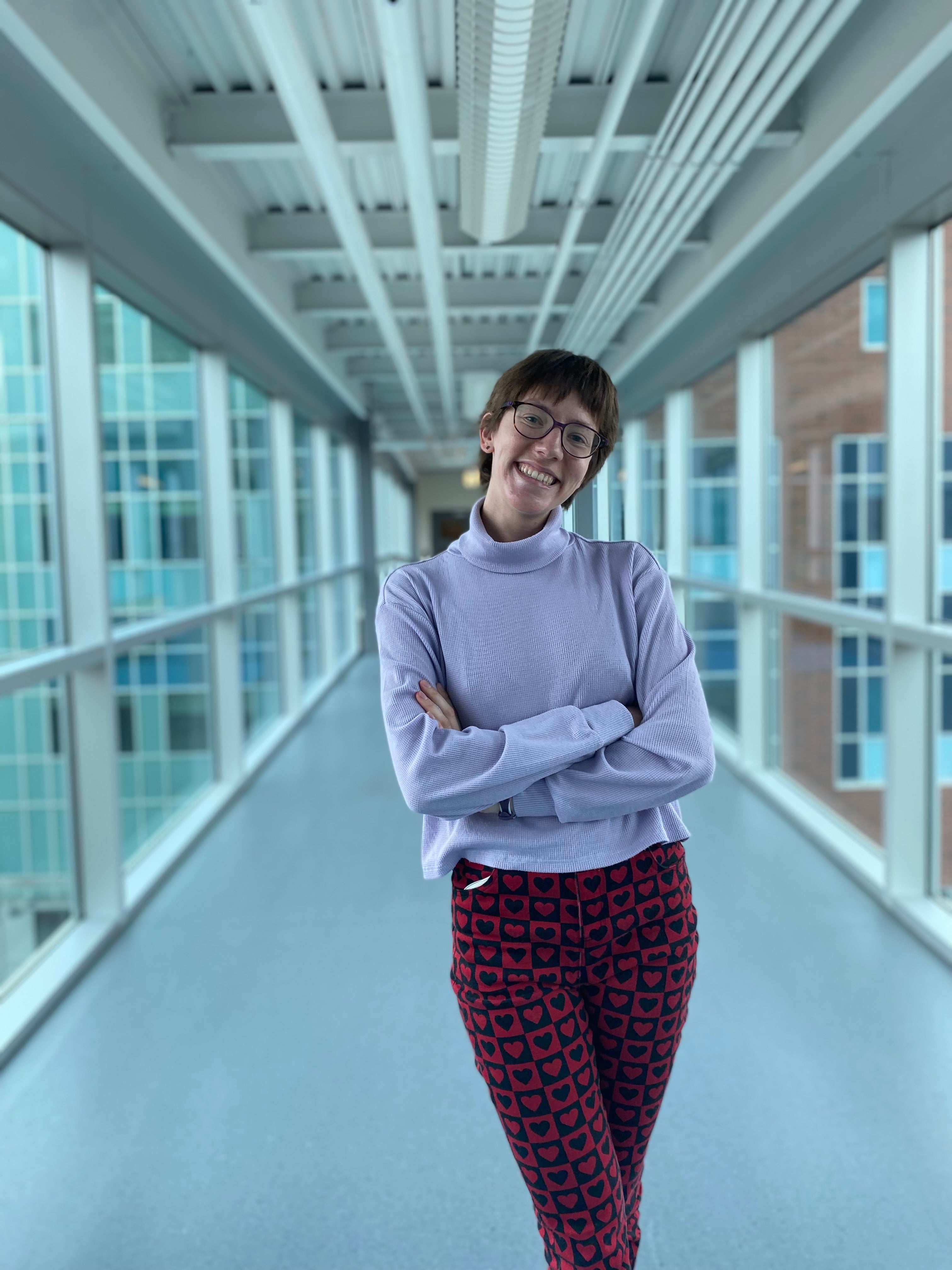
Research Abstract: Lightning Round
Spatial and Temporal Discounting in a Coupled Social-Climate Model
This research discovers the effects on mitigation and projected temperature anomalies through applying spatial and temporal discounting in human decision making in a coupled social – climate model. While past climate models have implemented a static human contribution to climate change through Representative Concentration Pathways and Shared Socioeconomic Pathways, this research develops and implements a dynamic social system coupled to the climate system. This idealizes a two-way feedback between human decision making, social norms, and human behaviour with changes in the climate. The spatial and temporal discounting addressed in this research is guided by the definition of judgemental discounting, such that individuals consider climate change conditions to be worse in other locations and further in time. We begin by implementing a coupled system of differential equations where a two-patch model for the human subsystem includes spatial discounting in the form of updated utility functions. The utilities determine the impacts of individuals in different populations taking up mitigative or non-mitigative strategies. Then temporal discounting is applied through percentage discounting per year within the system rather than a varied parameter. Results will be obtained through implementing the coupled feedback model with climate, social, and economic data. We hypothesize that the results will directly address the fundamental problem in dealing with climate change: where the negative effects of climate change are often portrayed as “there and later”, thus reducing the motivation to mitigate “here and now”.
Mackenzie Cameron's Biography
Mackenzie is a second year Master's student in Applied Math studying coupled human environment systems. Her research focuses on the interactions between human behaviour and climate change, stiving to model key barriers to mitigation. Apart from research, Mackenzie is an active member of the math faculty community through her role on the Math Graduate Student Association.
Amrita Punnavajhala
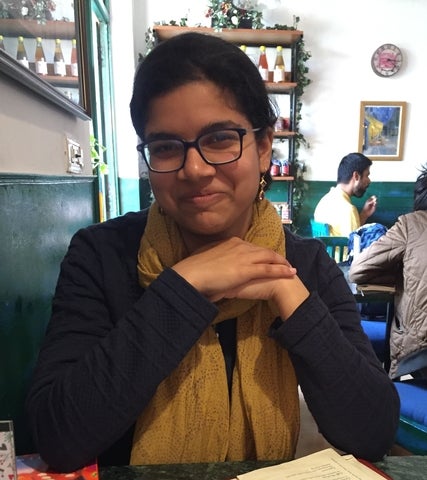
Research Abstract: Lightning Round
Climate change mitigation efforts across the world are informed by results from mathematical models of climate change. These models, while immensely useful, have been criticized for assuming that anthropogenic carbon emissions are driven solely by demographic and economic forces, thus neglecting descriptions of human behaviour. Recently, social and behavioural frameworks have started making their way into models of climate change, resulting in ‘coupled social-climate models’. Results from these models show that the inclusion of human behaviour, driven by social and psychological forces, can significantly alter climate change projections.
We build on existing work by constructing a coupled social-climate model with country-level structure and parameterization. This allows us to introduce the element of diverse, dynamic human behaviour, which we model using tools from evolutionary game theory. Ultimately, if we are to leverage individual behavioural change to aid climate change mitigation, we need to develop mathematical models to understand the complex interactions between human behaviour and climate change. This effort could also benefit us by predicting outcomes, yet unforeseen, which may prove crucial in helping us prepare our response to climate change.
Amrita Punnavajhala's Biography
Amrita is a third-year PhD candidate in Applied Mathematics. She works in the area of coupled social-climate models, focusing on the mathematical representation of diverse and dynamic human behaviour. She hopes that the outcomes of her research will be useful to climate change mitigation efforts.
Shervin Hakimi
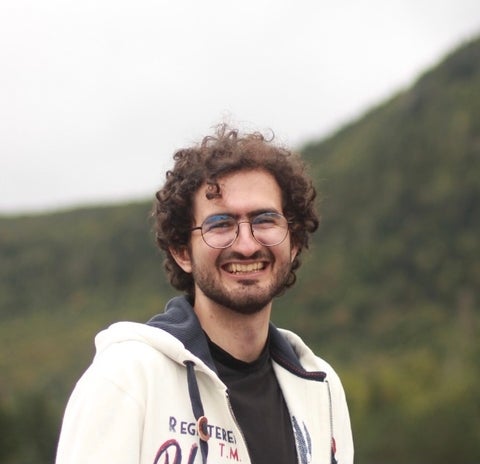
Research Abstract: Lightning Round
Beyond the Bones: Calcium Transport and Regulation in the Kidney
Calcium homeostasis is essential for the proper functioning of various cells and the kidney plays a vital role in this balance. The goal of this study is to expand our previous mathematical model of transepithelial transport along the male rat nephron to include the transport of calcium and to investigate the effects of inhibiting transepithelial sodium transport on urinary calcium excretion and its reabsorption along different nephron
segments. We simulated the effects of inhibiting the Na-K-2Cl transporter (NKCC2), reflecting the effects of the hypertensive drug, furosemide, the inhibition of the Na+/H+ exchanger (NHE3), the Na-Cl cotransporter (NCC), and the Epithelial Na channel (ENaC). Our results suggest that disruption in sodium transport significantly impacts renal calcium transport: NKCC2 inhibition significantly decreased calcium renal reabsorption along the thick ascending limb (TAL) and substantially increased calcium excretion by 8.7 folds in the case of complete inhibition. Our simulations of NHE3 inhibitions predicted reduced calcium reabsorption along the proximal tubule (PT) by ~46% and slightly increased calcium urine excretion. NCC inhibitions had slight effects on calcium reabsorption. The most notable effect of ENaC inhibition was the increased calcium reabsorption along the connecting tubule (CNT), resulting in a considerably reduced calcium excretion.
Shervin Hakimi's Biography
I'm currently pursuing a MMath degree in Applied Mathematics under the supervision of Dr. Anita Layton with a focus on calcium transport and regulation in the kidney. I'm particularly interested in studying the effects of drugs and investigating sex differences in these processes.
Before starting my Master's, I worked as a data scientist at Mindpax, a digital health company based in Prague that's committed to improving mental health outcomes through innovative technology. I received my Bachelor's degree in Electrical Engineering from Sharif University of Technology, where I focused on control theory. My thesis project involved investigating EEG signal applications to control wheelchair driving. In my free time, I enjoy learning new languages (currently fluent in English, Persian, German and a bit of French), reading, or being outdoors.
Cameron Meaney
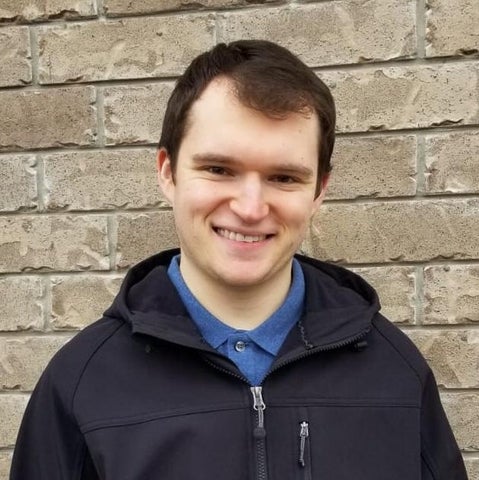
Research Abstract: Lightning Round
A longstanding problem in mathematical oncology is to accurately estimate the mathematical parameters which govern the growth of glioblastomas, a particularly aggressive form of brain tumour. While a method exists to derive these parameter estimates, it is plagued by problems including a high sensitivity to measurement error, a reliance on unrealistic mathematical assumptions, and a dependence on precise human measurements. Using deep learning techniques such as PINNs, it is possible to develop an algorithm that is capable of producing more accurate estimates of the key parameters while eliminating the shortcomings of the existing method. As part of my PhD research, I created a deep learning algorithm capable of making patient-specific predictions more accurately than the existing method. The data used in the research was collected by my collaborators at St. Michael’s Hospital and the University of Toronto. My computational approach allows for improved insight into the fundamental biological mechanisms that govern
tissue heterogeneity. This work has numerous implications in treatment planning, drug development, and patient survival.
Cameron Meaney's Biography
Cameron Meaney is a 4th year PhD candidate in the University of Waterloo Department of Applied Mathematics whose research focus is in mathematical oncology. His specializes in using mathematical models and machine learning approaches to better our understanding and treatment of cancer. In his previous work, he has developed improved procedures for radiation, chemo-, and nanoparticle therapies, as well as developed methods for furthering precision cancer medicine.
David Thomas Radke
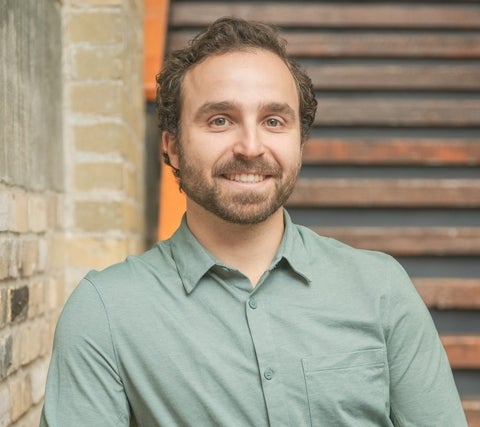
Research Abstract: Lightning Round
Individual artificial intelligence (AI) agents learning to work together is an important problem in multiagent systems (MAS). We propose a model for multi-objective optimization, a credo, for agents in a system that are configured into multiple groups (i.e., teams). Our model of credo regulates how agents optimize their behavior for the groups they belong to. We evaluate credo in the context of challenging social dilemmas with reinforcement learning agents. Our results indicate that the interests of teammates, or the entire system, are not required to be fully aligned for achieving globally beneficial outcomes. We identify two scenarios without full common interest that achieve high equality and significantly higher mean population rewards compared to when the interests of all agents are aligned.
David Thomas Radke's Biography
I am in my 5th year of my PhD at the University of Waterloo studying Artificial Intelligence with Dr. Kate Larson and Dr. Tim Brecht. My research focuses on understanding how teams and mixed incentives influence the behavior of learning agents. This includes research in multiagent reinforcement learning, multiagent systems, and team structures.
Pritha Dutta
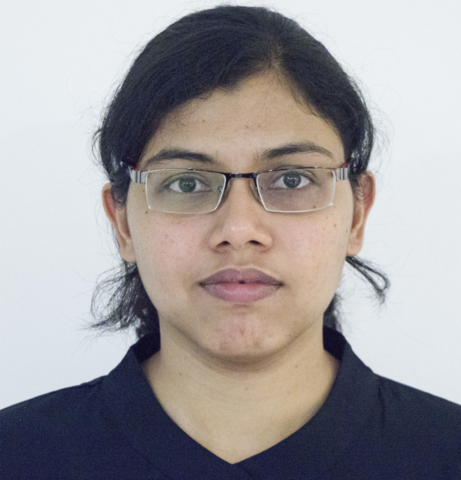
Research Abstract: Lightning Round
Kidneys: regulator of magnesium homeostasis
Magnesium plays an important role in several physiological functions and metabolic and biochemical processes within the cell and its deficiency has been associated with neurological disorders, heart disorders, hypertension, and type 2 diabetes. The kidneys play a central role in maintaining serum magnesium within a narrow range (0.70–1.10 mmol/L). In this study, an epithelial cell-based computational model of solute transport in rat kidneys was developed to understand the mechanism of magnesium transport along different nephron segments. Since magnesium transport is tightly coupled to sodium transport, the model was used to simulate the effects of different antihypertensive diuretics on renal magnesium reabsorption. The simulations showed that loop and thiazide diuretics result in increased magnesium excretion, whereas potassium-sparing diuretics favor magnesium retention.
Pritha Dutta's Biography
Pritha Dutta is a Postdoctoral Fellow in the Department of Applied Mathematics, University of Waterloo, Canada. She is currently working on sex-specific computational models of calcium and magnesium transport in the kidney and whole-body magnesium homeostasis in Professor Anita Layton’s lab. She completed her Ph.D. from Nanyang Technological University, Singapore in 2022. During her Ph.D., she worked on mathematical models of pancreatic beta-cell dysfunction and insulin resistance in the presence of hyperglycemia. She is interested in Computational Biology and Complex Systems.
Mojtaba Valipour
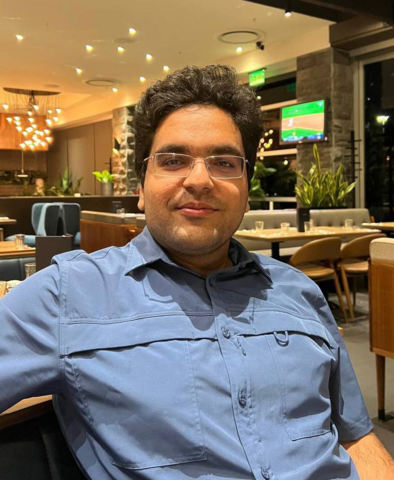
Research Abstract: Lightning Round
Daneshbaz: Open Research, Open Problem, Open Ideas.
Publishing a paper can take a long time, and collaborating with someone you don't know can be challenging. Throughout their careers, researchers encounter these issues, but it is particularly crucial for those in their early stages and newcomers, such as graduate students. Their small network limits their ability to facilitate such collaborations due to their inexperience and lack of publications. In addition, because the status quo usually prevents publication updates, papers will no longer be subject to revisions. This can create a unique challenge for those just entering the research field, as they may not have the resources or experience to navigate these obstacles. Moreover, without periodic revisions, the integrity of the published work is put at risk. Daneshbaz.com supports current practices while improving the whole process, advocating for a transparent, fast, and hassle-free publication system. Our proposed approach creates a more efficient publishing ecosystem, facilitating collaboration among researchers, introducing an alternative to dead papers, and helping shape research's future. There is no doubt that Daneshbaz is a revolution in research.
Mojtaba Valipour's Biography
Mojtaba Valipour is a Ph.D. candidate studying computer science at the University of Waterloo. He is passionate about Artificial General Intelligence, Machine Learning, and Causal Inference. His research focuses on devising novel approaches to make large language models more efficient and controllable. In addition, he views the current scholarly publication system as outdated and inefficient. He aims to develop a platform that removes barriers for researchers, allowing them to focus on their research and publish scientific results without hassles.
Kanav Mehra
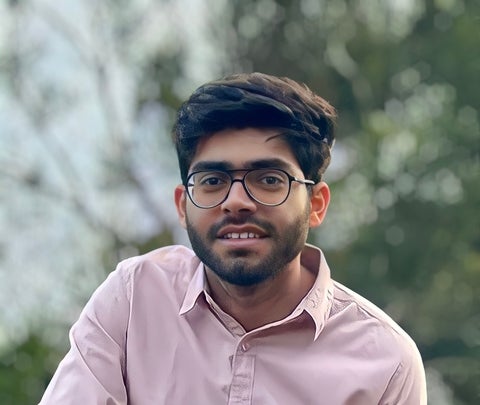
Research Abstract: Lightning Round
Citizen-focused democratic processes where participants deliberate on alternatives and then vote to make the final decision are increasingly popular today. While the computational social choice literature has extensively investigated voting rules, there is limited work that explicitly looks at the interplay of the deliberative process and voting. In this paper, we build a deliberation model using established models from the opinion-dynamics literature and study the effect of different deliberation mechanisms on voting outcomes achieved when using well-studied voting rules. Our results show that deliberation generally improves welfare and representation guarantees, but the results are sensitive to how the deliberation process is organized. We also show, experimentally, that simple voting rules, such as approval voting, perform as well as more sophisticated rules such as proportional approval voting or method of equal shares if deliberation is properly supported. This has ramifications on the practical use of such voting rules in citizen-focused democratic processes.
Kanav Mehra's Biography
Kanav Mehra is a Master’s student in computer science at the University of Waterloo. His broad research interests lie in the domain of artificial intelligence, computational social science, and multiagent systems, especially where these methods can be applied to derive insights for governance, policy-making, and human-system interactions. His current research is focused on designing fair and proportional deliberation and voting mechanisms for elections in participatory democratic processes, with the objective of improving minority representation in public decision-making and local governance. Furthermore, he is also studying how well-studied notions of fairness and proportionality developed for social choice literature (voting systems) can be appropriately modified to develop fair and proportional search and recommender systems. Previously, his research focused on building novel, scalable methods that leverage social media data to assist relief operations during disasters and emergencies. In this capacity, he also volunteered as a Data Scientist for the Data Science for Social Good foundation (DSSG Solve) and the International Water Management Institute (IWMI) to help his local community impacted by a devastating cyclone.
Rafael F. Toledo
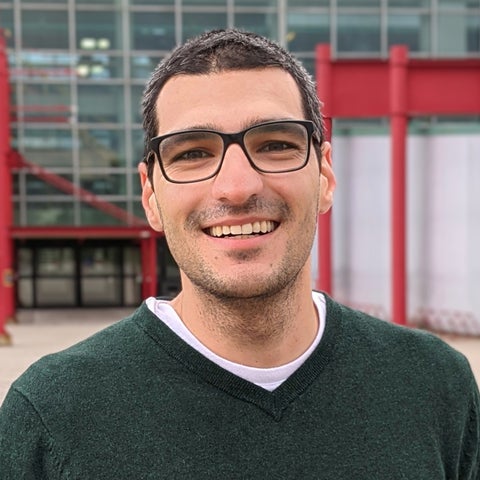
Research Abstract: Lightning Round
Visualizing Analysis Results of Industrial Automotive Software Product Line Models
Program analysis of automotive software has several unique challenges, including that the code base is ultra large, comprising over a hundred million lines of code running on a single vehicle; the code is structured as a software product line (SPL) for managing a family of related software products from a common set of artifacts; and the analysis results (despite being numerous and despite being variable) need to be presented to the engineer in a way that is manageable. My research focus is investigating effective ways to report to engineers conditional analysis results (i.e., results conditioned on SPL program variants).
Rafael F. Toledo's Biography
Rafael is a PhD candidate and a member of the Waterloo Formal Methods Group under the supervision of Prof. Joanne Atlee. His research investigates the use of visual interfaces for the inspection and comprehension of analysis results of large heterogeneous systems. He focuses in developing interactive lightweight models to represent the variability-aware analysis results of software product lines. Such models enable engineers to visualize, explore, and compare the behaviour of specific software products
Mehrshad Sadria
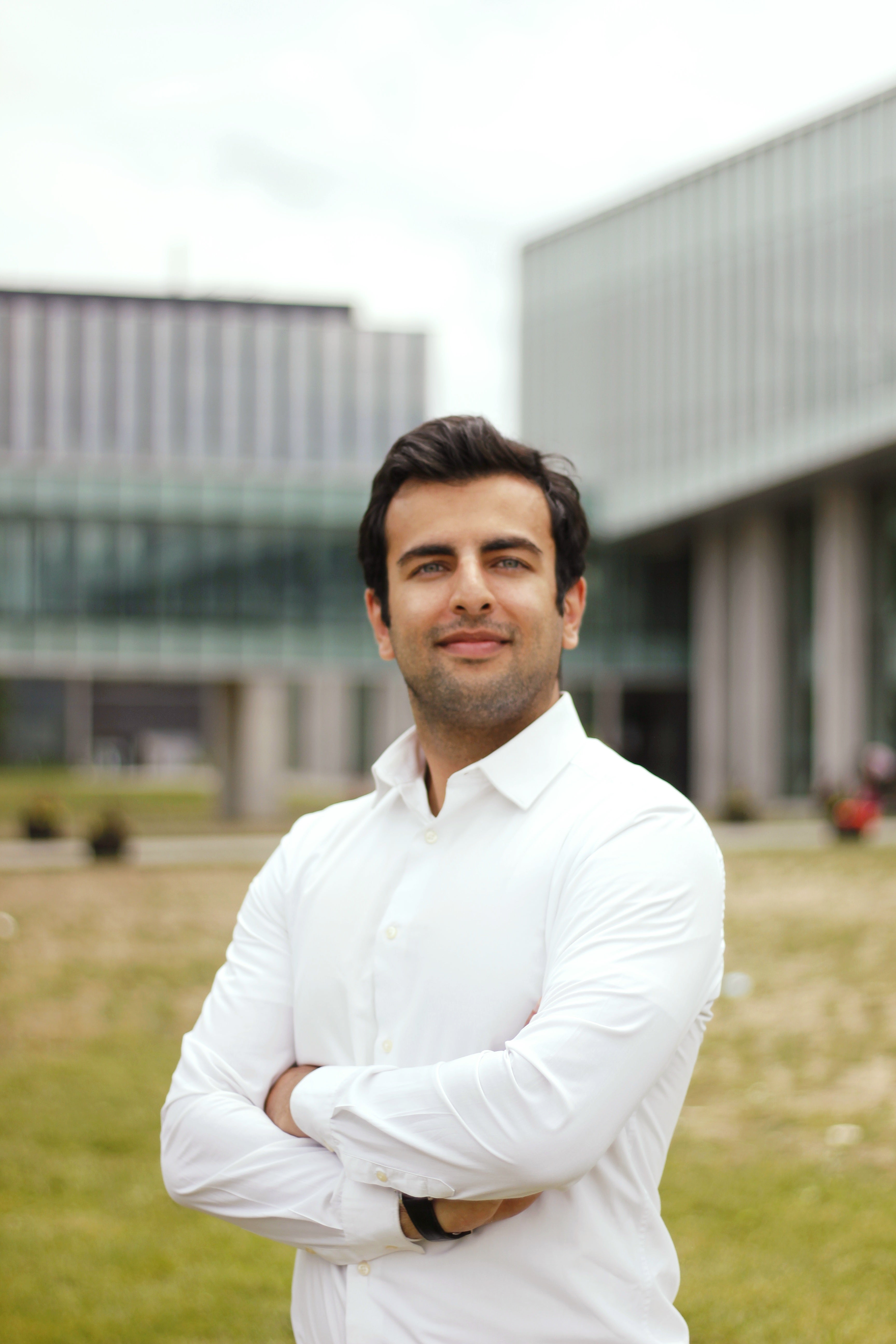
Research Abstract: Lightning Round
Deep Learning (DL) methods have demonstrated significant potential in solving various challenges in biology and medicine, from early diagnosis to predicting the impact of cellular perturbations. One of the promising areas for applying DL is in cell reprogramming, which involves transforming one cell type into another. DL models can be trained on gene expression data to recognize the critical genes and master regulators that govern cell fate, enabling researchers to create more effective reprogramming techniques. Furthermore, DL may assist in identifying fresh targets for therapeutic intervention. Also, these models can be used to understand morphological and structural changes in disease progression. However, one of the
main issues with these models is that they challenging to interpret and may not always learn the correct embedding, leading to potentially unreliable predictions. During my talk, I will introduce new methods that can improve the interpretability and precision of DL models in biological settings.
Mehrshad Sadria's Biography
Mehrshad Sadria is a PhD candidate under the supervision of Prof. Anita Layton at UW and Prof. Gary Bader at U of T. His research is comprehensive and covers a diverse range of subjects. A significant aspect of his work is centred around developing computational methods, specifically deep learning models to analyze data generated from single-cell technology. These models have the potential to facilitate the discovery of novel treatments for various diseases and can enhance the field of regenerative medicine. Furthermore, he is actively exploring the combination of deep learning approaches with physics-based models to create more precise, efficient, and generalizable.
Research Abstract: Lightning Round
Childhood absence epilepsy (CAE) is an idiopathic generalized epilepsy disorder which affects children between the ages of 4-12 years. It is characterized by sudden brief periods of impaired consciousness occurring several times a day. One of the most confounding features of CAE is its ability to spontaneously resolve in adolescence in roughly 80% of cases, while in others it can progress into more severe types of epilepsy. There remains an inadequate understanding of some of the factors involved in remission that can inform early intervention practices and our research will help fill in those gaps. According to some functional connectivity studies, there exist pre-treatment connectivity differences between patients who ultimately experience remission and those who do not, namely increased frontal cortical connections at the time of diagnosis. There is also substantial evidence on the effect of sex steroids on human (and animal) brain rhythmic discharges, particularly the effect of progesterone metabolite allopregnanolone on the action of gamma-Aminobutyric acid (GABA), an inhibitory neurotransmitter. We have developed a computational model of the thalamocortical network to study the role of allopregnanolone on network behaviour in the eventual resolution of CAE. The results from this research can possibly better inform therapeutic decisions relating to early interventions tailored to intrinsic network connectivity arrangements, which can be evaluated prior to the start of treatment and at the time of diagnosis.
Maliha Ahmed's Biography
Maliha Ahmed is a third-year PhD candidate in the Department of Applied Mathematics under the supervision of Prof. Sue Ann Campbell. Her research interest is in computational neuroscience, with a particular focus on models of the thalamocortical system. She has previously worked on modelling genetic defects in a cortical circuit model associated with childhood absence epilepsy. Currently, she is developing a thalamocortical network model to study intrinsic differences in connectivity as well as the effect of sex steroid hormones on the resolution of absence seizures. Understanding the role of sex hormones in the resolution of childhood absence epilepsy may explain why remission does not occur in other cases. Results from this research can therefore lead to better informed therapeutic decisions relating to early interventions such as hormonal therapy and/or antiepileptic drug treatment, which can be tailored to intrinsic network connectivity arrangements, based on functional connectivity analysis of neuroimaging data of patients.
November 2023 Mitigating Risks of AI
Keynotes| Mitigating the Risks of AI |November 2, 2023
Maura Grossman
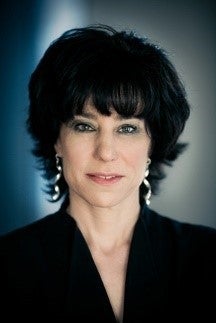
Biography
Maura R. Grossman, J.D., Ph.D., is a Research Professor in the School of Computer Science and the School of Public Health Sciences at the University of Waterloo, an Adjunct Professor at Osgoode Hall Law School of York University, and an affiliate faculty member at the Vector Institute of Artificial Intelligence, all in Ontario, Canada. She also is Principal at Maura Grossman Law, an eDiscovery law and consulting firm in Buffalo, New York. Maura has been widely recognized for her scholarly work evaluating the application of supervised machine-learning technologies (i.e., technology-assisted review or TAR) in the fields of law, government archives, and healthcare. Her studies have frequently been cited in the case law and literature, in North America and abroad. More recently, Maura’s scholarship has focused on responsible Artificial Intelligence (AI) and AI as evidence. Maura is also well known for her appointments as a special master and/or as an expert in many high-profile U.S. federal and state court cases. In addition to her J.D. from the Georgetown University Law Center, Maura also holds M.A. and Ph.D. degrees in Psychology from the Derner Institute of Adelphi University.
Keynote Address
Achieving Trustworthy AI: Is It Possible Today?
This talk will begin by laying the groundwork: What do we mean by “AI” and “Generative AI”? How do they work? What can they do? How are they being used in industry? Next, we’ll turn to the characteristics that make AI trustworthy and explore the questions of whether it is possible to achieve them all today and if not, what trade-offs we are prepared to make. There will be plenty of time for questions and discussion.
Florian Kerschbaum
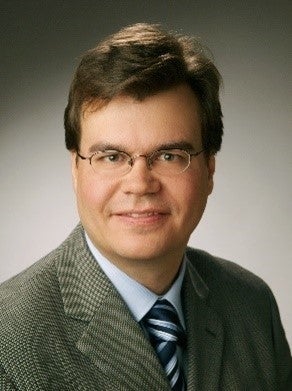
Biography
Dr. Kerschbaum is a Professor in the David R. Cheriton School of Computer Science at the University of Waterloo (joined in 2017), a member of the CrySP group, and NSERC/RBC chair in data security (since 2019). Before academia, he worked as chief research expert at SAP in Karlsruhe (2005 – 2016) and as a software architect at Arxan Technologies in San Francisco (2002 – 2004). He hold a Ph.D. in computer science from the Karlsruhe Institute of Technology (2010) and a master's degree from Purdue University (2000). He served as the inaugural director of the Waterloo Cybersecurity and Privacy Institute (2018 – 2021). He is an ACM Distinguished Scientist (2019), a winner of the Outstanding Young Computer Science Researcher Award from CS-Can/Info-Can (2019) and a winner of the Faculty of Math Golden Jubilee Research Excellence Award (2022). He is interested in security and privacy in the entire data science lifecycle. He extends real-world systems with cryptographic security mechanisms to achieve (some) provable security guarantees. His work is used in several business applications.
Keynote Address
Reliably Labelling AI Generated Content.
In this talk, Professor Kerschbaum will discuss the requirements and definitions for reliable AI content labelling, how to implement it using watermarking and review techniques for private content retrieval.
Mei Nagappan
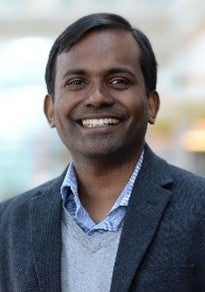
Biography
Mei Nagappan is an Associate Professor in the David R. Cheriton School of Computer Science at the University of Waterloo. He was a postdoctoral fellow at the Software Analysis and Intelligence Lab at Queen's University and completed his Ph.D. in the Computer Science Department of North Carolina State University. His research interests are in Empirical Software Engineering by mining software repositories. He currently looks at how large language models will impact software development.
Keynote Address
A Hopeful Case For Generative AI In Software Engineering.
Can generative AI automate away software engineering jobs? The answer right now is, "No, but they are still useful." While generative AI is not going to automate away SE jobs, it is certainly going to change the way software engineers work. If someone's expertise is only writing code, they will almost certainly be replaced by generative AI in the near future. However, the parts of software engineering before and after writing code will gain increased prominence. As a call to action to my fellow researchers, I say that we should be working to make generative AI more usable for software engineers and figure out how to incorporate them into our workflows.
Panel Discussion|November 2, 2023
Axel Ngonga
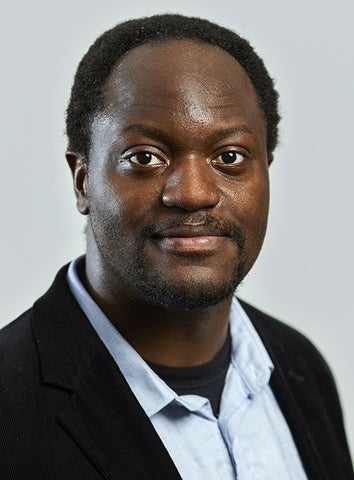
Biography
Axel Ngonga is a full professor of Data Science at Paderborn University in Germany. He studied Computer Science in Leipzig. In his PhD thesis, he developed knowledge-poor methods for the extraction of taxonomies from large text corpora.
After completing his PhD, he wrote a habilitation on link discovery with a focus on machine learning and runtime optimization. In his current research, he focuses on data-driven methods to improve the lifecycle of knowledge graphs. These include techniques for the extraction, verification, integration and fusion of knowledge graphs, their use in machine learning, and their exploitation in user-facing applications such as question answering systems and chatbots. He is the grateful recipient of over 25 international research prizes, including a Next Einstein Fellowship, a Lamarr Fellowship, and 6 best research paper awards. Axel currently leads two European training networks on knowledge graphs and machine learning. He is also the coordinator of the European research innovation action ENEXA, which develops scalable algorithms for inconsistent data.
Ehsan Amjadian
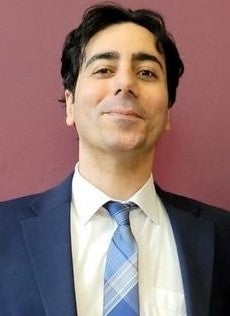
Biography
Dr. Ehsan Amjadian is the Head of AI Solution Acceleration & Innovation at the Royal Bank of Canada (RBC). He has led numerous advanced AI products and initiatives from ideation to production and has filed multiple patents in the areas of Data Protection, Finance & Climate, and Computer Vision & its applications to Satellite Images.
He earned his Ph.D. in Deep Learning & Natural Language Processing from Carleton University, Canada and is presently an Adjunct Professor of Computer Science at University of Waterloo. He is published in a variety of additional Artificial Intelligence and Computer Science domains including Recommender Engines, Information Extraction, Computer Vision, and Cybersecurity.
Koray Karabina
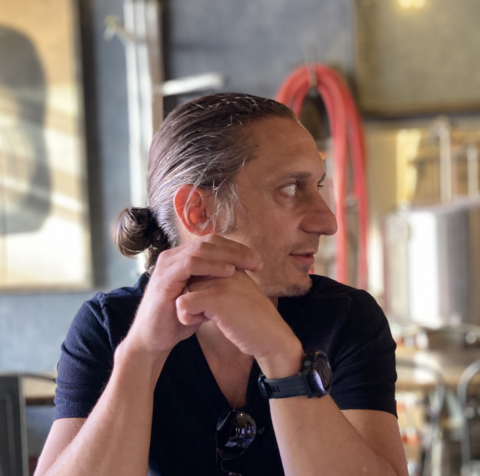
Biography
Koray Karabina leads the Cryptography and Quantum Computing Team at the National Research Council of Canada, and he is appointed as an adjunct associate professor at the University of Waterloo. Koray Karabina’s research focuses on the design, implementation, and analysis of cryptographic algorithms and protocols. He has a strong interest in the interaction between AI, privacy, and cryptography.
Lai-Tze Fan
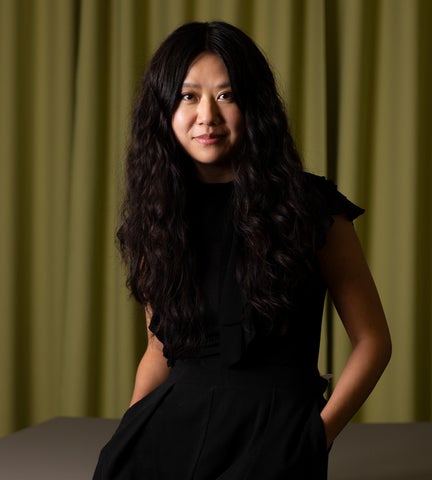
Biography
Lai-Tze Fan is the Canada Research Chair of Technology and Social Change, and an Assistant Professor of Sociology & Legal Studies and English Literature at the University of Waterloo, Canada. She is Professor II at the Center for Digital Narrative in the University of Bergen, Norway, and the Founder and Director of the Unseen-AI Lab at Waterloo, which uses artistic and creative methods for enhanced EDI outcomes in AI design. She is on the Board of Directors of Waterloo’s TRuST research network, targeting misinformation and trust in AI. Her work focuses on systemic inequalities in technological design and labour, digital storytelling, research-creation, critical making, and media theory and infrastructure.
Research Spotlights|November 2, 2023
Xinyu Crystina Zhang
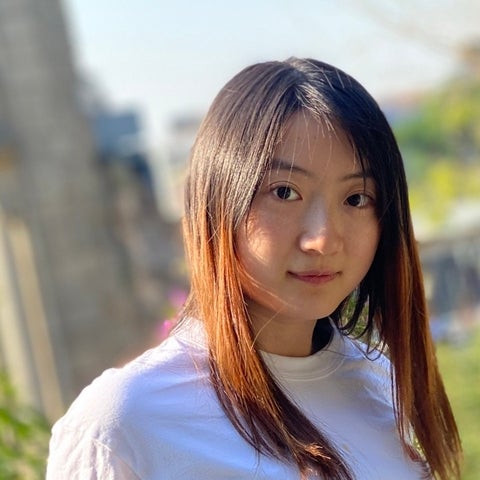
Biography
Crystina is a PhD student at University of Waterloo, supervised by Prof. Jimmy Lin. Crystina received a Bachelor's degree of Computer Science at Hong Kong University of Science and Technology (HKUST). She has interned in NAVER Clova Lab, MPI, and Cohere, and will start the student researcher journey at Google shortly. Her research interests lie in-between IR and NLP, while especially focusing on IR in multilingual settings.
Research Spotlight
No Language Left Behind from the Lens of IR
Information retrieval allows users to access desired information from massive collections. To satisfy this demand, it is essential that work in IR covers diverse languages. While more IR resources have been developed for non-English languages, there is still a clear disparity in the amount of research activity across different languages, even on the languages that have a large base of native speakers.
Thomas Humphries
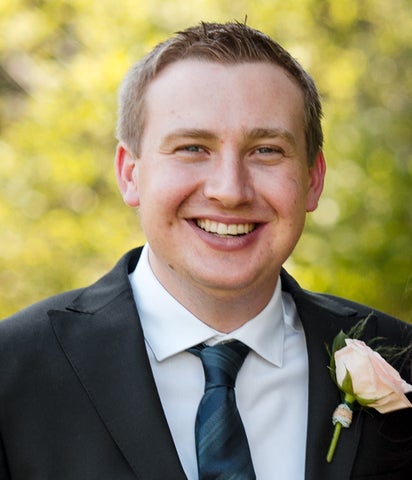
Biography
Thomas Humphries is a PhD student supervised by Prof. Florian Kerschbaum in the David R. Cheriton School of Computer Science at the University of Waterloo. His research interests include private machine learning and protocols at the intersection of differential privacy and secure multi-party computation. Thomas is a NSERC Postgraduate Scholar, and his work has appeared in top-tier venues such as ACM CCS, VLDB, and PETS.
Research Spotlight
Investigating Membership Inference Attacks Under Data Dependencies
In this talk, we discuss our work evaluating what happens when we relax the IID assumption and consider more realistic scenarios. We show that without the IID assumption, the previous theoretical analysis linking differential privacy to membership inference is no longer meaningful (the privacy parameter scales with the training set size). We conduct a series of empirical evaluations with off-the-shelf MIAs using training sets built from real-world data showing different types of dependencies among samples. Our results reveal that training set dependencies can severely increase the performance of MIAs and surpass the previous theoretical upper bounds. We first shared our findings in 2020 and they were successfully peer-reviewed in 2023. Today, our work is corroborated by an increasing number of works in large language models.
Noble Saji Mathews
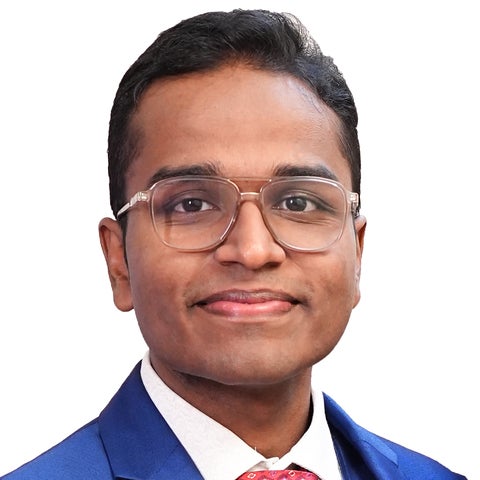
Biography
Noble Saji Mathews is an MMath scholar at the University of Waterloo, where he works under the mentorship of Dr. Mei Nagappan, as part of the SoftWare Analytics Group (SWAG). Noble’s work is defined by his deep interest in leveraging technologies to refine and improve software development processes. Straddling the exciting intersection of Software Engineering and Artificial Intelligence, Noble has conducted research on a plethora of topics, including Cross Language Static analysis, Dynamic analysis, Exploit Generation, Code View Generation, and Source Code Representation Learning.
Currently, Noble is focusing on harnessing the incredible potential of Large Language Models (LLMs) for Neural Code Generation, He is a Mitacs Globalink Graduate Fellow and an AI Engineer at an avant-garde start-up dedicated to Automated Vulnerability Detection and Code Remediation using LLMs.
Research Spotlight
LLMs In Action: Towards Automating Bug Fixes And Enhancing Code Security
How can we harness the power of Large Language Models (LLMs) in building effective, real-world applications? This talk aims to shed light on the immense potential of LLMs in code development. Featuring a case study on code security and building automatic code remediation systems we highlight how addressing fundamental limitations of these models can lead to the creation of powerful systems, making impressive feats in software engineering achievable.