Research Description
We are developing model-predictive control (MPC) algorithms to integrate planning and motion control for autonomous vehicles. These algorithms are deployed using ROS on the University of Waterloo “Autonomoose”. Vehicle and tire models are developed from experimental tests using our intelligent vehicle measurement system and controllers are evaluated in virtual simulations and hardware-in-loop experiments prior to vehicle testing.
Student Researchers
•
Yuan
Lin
•
Chi
Jin
•
Anson
Maitland (Alumnus)
•
Mohammad
Hassanpour (Alumnus)
Keywords and Themes
•
Model-Predictive
Controllers
•
Autonomous
and
Connected
Vehicles
•
Intelligent
Vehicle
Systems
•
Automated
Planning
and
Control
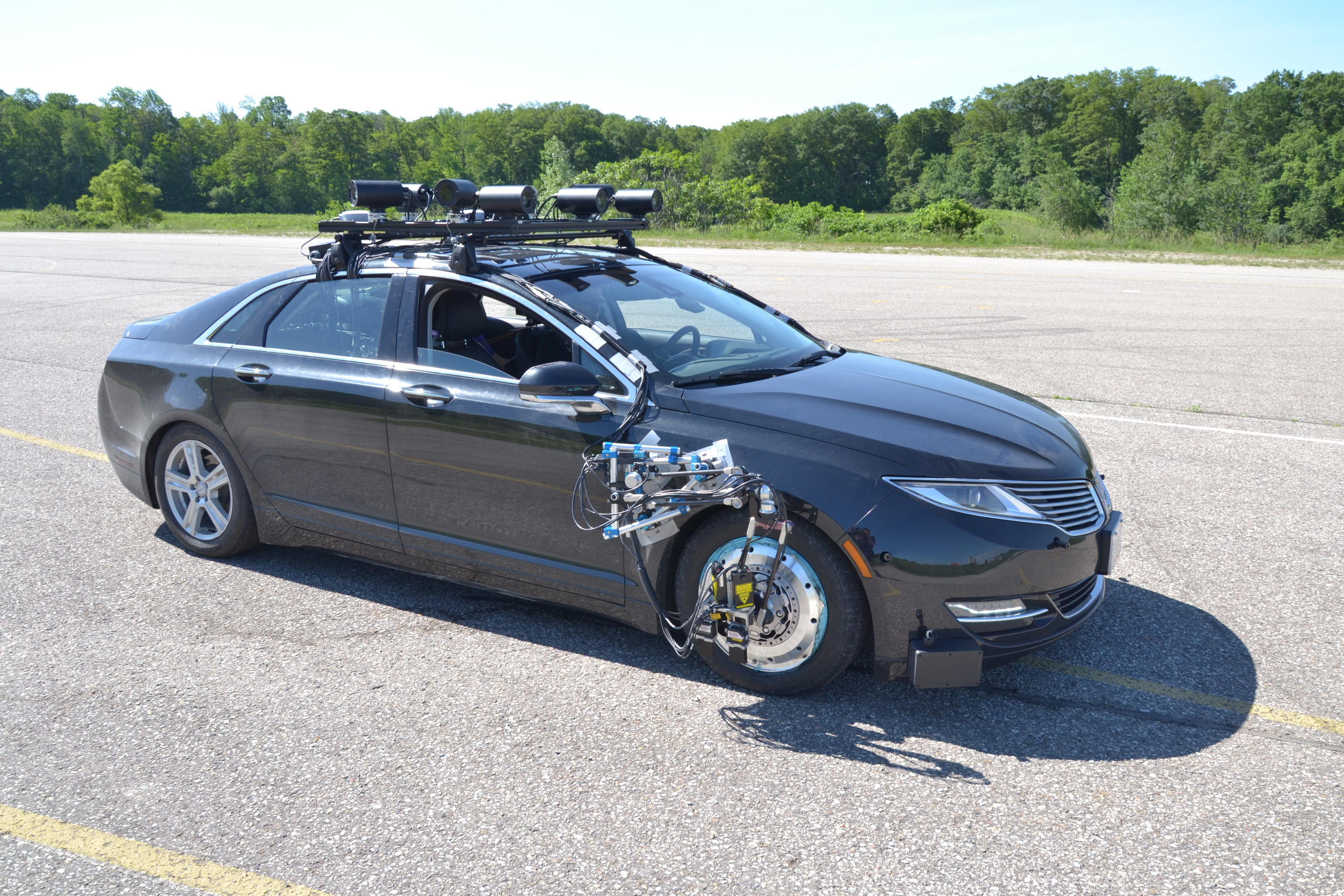
Related Publications
•
Lin
Y,
McPhee
J,
and
Azad
NL.
(2019).
Longitudinal
Dynamic
versus
Kinematic
Models
for
Car-Following
Control
Using
Deep
Reinforcement
Learning.
IEEE
Intelligent
Transportation
Systems
Conference.
arXiv:1905.08314.
• Batra
M,
McPhee
J,
and
Azad
NL.
(2018).
Real-Time
Model
Predictive
Control
of
Connected
Electric
Vehicles.
Vehicle
System
Dynamics:
International
Journal
of
Vehicle
Mechanics
and
Mobility.
DOI:
10.1080/00423114.2018.1552004.
• Maitland
A
and
McPhee
J.
(2018).
Towards
Integrated
Planning
and
Control
of
Autonomous
Vehicles
Using
Nested
MPCs.
ASME
2018
Dynamic
Systems
and
Control
Conference.
DOI:
10.1115/DSCC2018-9224.