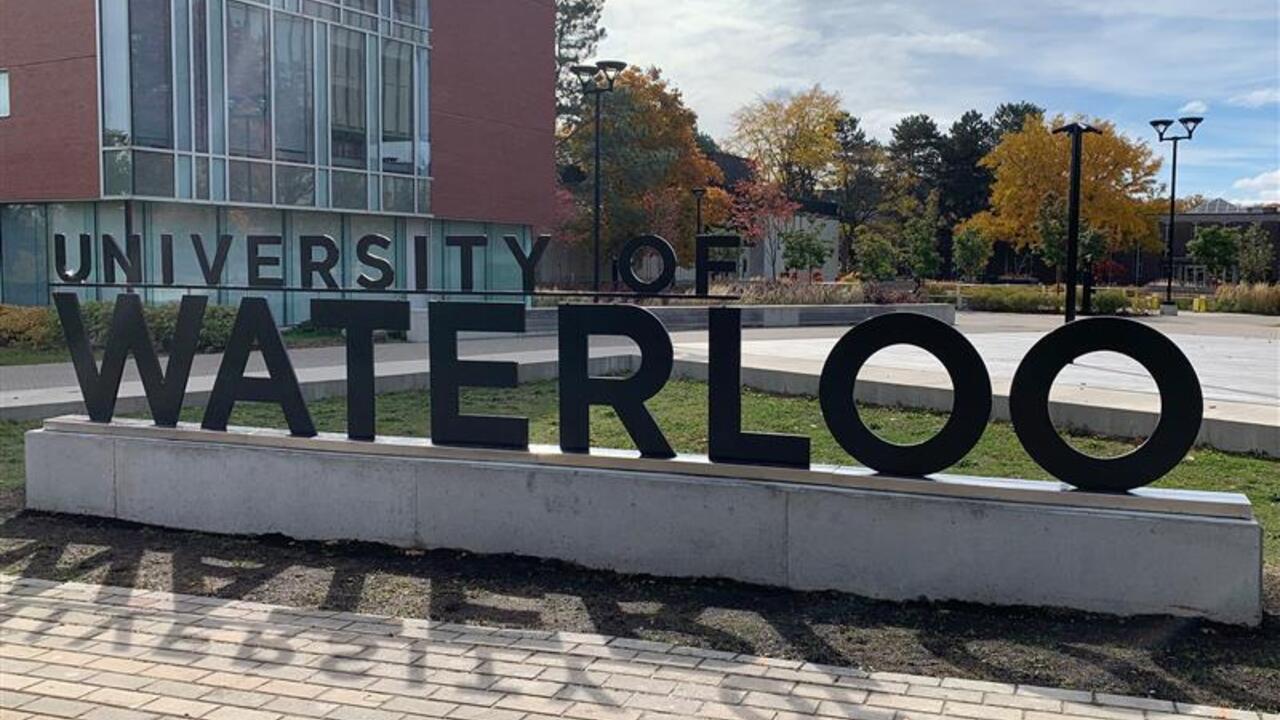
Machine-learning earnings: Novel approach triples investment success
University of Waterloo researchers have developed a new framework that increases the probability of meeting your investment target by threefold.
University of Waterloo researchers have developed a new framework that increases the probability of meeting your investment target by threefold.
By Media RelationsThe data-driven approach, which is enhanced by machine learning, significantly outperforms traditional methods used by investors and people saving for retirement.
Their research was motivated by the fact that both the private and public sectors are shifting away from defined benefit (DB) pension plans to defined contribution (DC) plans – a trend recently highlighted in a Federal Reserve Economics Research Note.
The novel approach provides a strategy for employees who, as participants in a DC pension plan, will have to make decisions on how to allocate their assets to ensure a reasonable level of salary replacement upon retirement.
“If one considers that the DC fund will be managed by the employee for over 30 years of employment, it is clear that asset allocation will be of crucial importance,” said Peter Forsyth, co-author of a paper detailing the new framework and a Distinguished Professor Emeritus in Waterloo’s David R. Cheriton School of Computer Science.
“A standard strategy that financial advisors use when guiding clients on how to invest their retirement savings, is to put 60 per cent in stocks and 40 per cent in bonds, with periodic rebalancing. It’s not a bad strategy but we can do significantly better. Our strategy reduces the probability of not reaching your savings goal from 50 per cent to 15 per cent.”
In developing the multi-period neural network optimization framework, the researchers directly imputed historical data into the machine learning algorithm, and in doing so bypassed the traditional method of constructing a parametric model with a fixed set of parameters.
As a test, they generated some scenarios from a parametric model and asked the machine-learning algorithm to provide the best possible strategy without revealing to it what the model was, where it came from or any other detail. The machine learning algorithm was able to produce the correct strategy despite having only been provided with the scenarios.
“We found that the machine learning can actually learn the decision-making function very accurately which allows us to bypass the error-prone assumption making steps used in traditional methods,” said Yuying Li, co-author of the paper and a computer scientist in Waterloo’s Faculty of Mathematics.
“We then looked at real market data and found that our approach offers great potential for incorporating much more information into decision making. It’s also better able to solve high-dimensional problems where there are many more uncertain factors, while the classical approach could not solve these problems.”
The paper, titled A Data-driven Neural Network Approach to Optimal Asset Allocation for Target Based Defined Contribution Pension Plans, was recently published in the journal Insurance: Mathematics and Economics.
Read more
Velocity pitch competition winners share exciting startup ideas using artificial intelligence and deep tech, showcasing creativity and entrepreneurial prowess
Read more
University of Waterloo establishes prestigious industry-sponsored research chair in network automation to be held by Raouf Boutaba
Read more
New statistics research provides insight on when a track-and-field athlete’s performance peaks
The University of Waterloo acknowledges that much of our work takes place on the traditional territory of the Neutral, Anishinaabeg, and Haudenosaunee peoples. Our main campus is situated on the Haldimand Tract, the land granted to the Six Nations that includes six miles on each side of the Grand River. Our active work toward reconciliation takes place across our campuses through research, learning, teaching, and community building, and is co-ordinated within the Office of Indigenous Relations.