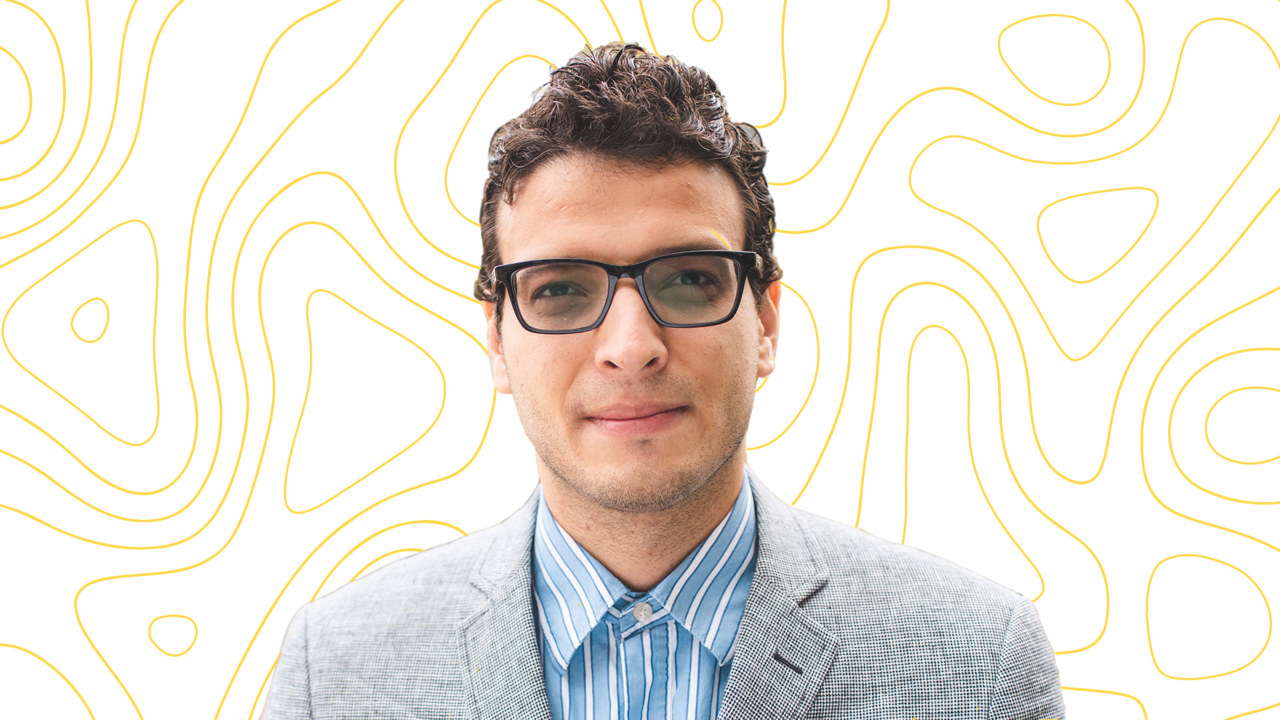
Alumni Know: How do we know public policies worked?
Data is easier to collect than ever before. Andres Arcila (PhD '20) explains why data is changing public policy and how you can find truth in the numbers.
Data is easier to collect than ever before. Andres Arcila (PhD '20) explains why data is changing public policy and how you can find truth in the numbers.
By Megan Vander Woude Office of AdvancementAs tax-payers, we have a right to know what policies our dollars support — and how effective they are. Politicians and public agencies want to tell us how many people were helped by a new program, how many new jobs they created and how much money was saved because of their choices. But where do those numbers come from? And how do we know they're credible?
Today, public agencies are under pressure to collect and interpret data that reveals exactly what happened when a policy was implemented. Andres Arcila (PhD '20) joins to explain how this is changing public policy and help us understand the truth behind public data.
Andres is a senior research data scientist with AB Inbev, where he develops demand estimations and economic forecasts in the brewing industry. He also holds a PhD from Waterloo, specializing in policy evaluation and applied economics. This fall, Andres will return to Waterloo to teach a data analytics certificate program for public servants and business professionals, offered through WatSPEED.
In the past, we were more limited in the data we had to evaluated policies. Often, we relied on simple regression analysis, where you analyze two variables (one dependent and the other independent), to say that a certain outcome was affected by a policy. But this can leave out other variables that exist, and it left policymakers with the challenging task of finding "good" data. (1:41) Thanks to new tools and software, public agencies have become much better at collecting and analyzing information from their own policy interventions. Plus, the vast majority of people today own smartphones and wearable devices that allow us to generate and record data every second. Andres points to a recent UWaterloo study that used Google mobility data as a good example. (2:20)
Correlation refers to the degree that a pair of variables are related. Causation refers to the influence one variable has on the production of another. To better explain the difference, Andres points to a Twitter meme about soccer player Aaron Ramsey. A few years ago, Twitter users discovered a correlation between Ramsey's goals and celebrity deaths. Every time Ramsey scored a goal, a celebrity seemed to die. These two variables are correlated, but obviously goals scored in a soccer game have no effect on death rates. Correlation between two variable sets doesn't mean one caused the other. (4:21) Andres is quick to point out that correlation isn't a bad thing to analyze. Identifying correlations between two variables can also provide valuable information, and he points to one study that uses light intensity from satellite maps to determine the locations of urban developments. (5:40)
This is a very difficult question to answer because it's very easy to lie or disguise with statistics. The best advice Andres can give is to look at the story behind the data. Ask yourself: Do the details of the story make sense? How did they collect this information? Are the numbers consistent with the story being told? (10:45)
Read more
Financial advisor Alexandra Horwood (BA '10) explains the truth behind financial services and fees
Read more
Arjun Moorthy (BASc '99) speaks on sensationalism, bias and being a good news consumer
Read more
Anne Fannon (MA '03) offers valuable insights about today's young workers and how employers can offer top talent the best experience
The University of Waterloo acknowledges that much of our work takes place on the traditional territory of the Neutral, Anishinaabeg, and Haudenosaunee peoples. Our main campus is situated on the Haldimand Tract, the land granted to the Six Nations that includes six miles on each side of the Grand River. Our active work toward reconciliation takes place across our campuses through research, learning, teaching, and community building, and is co-ordinated within the Office of Indigenous Relations.