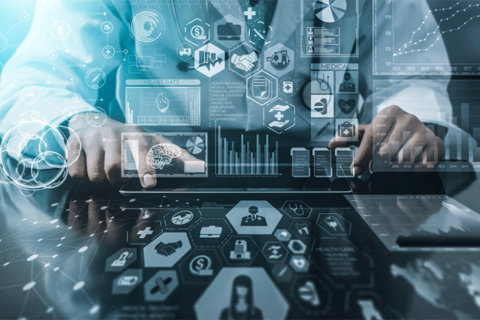
Big data is changing the way data assessment is done. The challenges in dealing with complex datasets are present throughout the whole data pipeline, especially in the healthcare domain. Technical limitations (e.g. database structure, programming language, etc.), as well as ethical implications (e.g. privacy and consent), impose constraints, creating siloed databases, and, therefore, making it technically hard to integrate and combine information. Ultimately the main goal, from a healthcare standpoint, is to achieve a holistic understanding of the patients’ condition. Data visualization is, therefore, an essential tool to ground decision-making processes of clinicians.
A new approach to healthcare that encompasses caregiving in a holistically manner is what have emerged in the past decade as precision medicine. An individual's health is perceived as a combination of factors that goes beyond their clinical status (e.g., EMR). It includes factors such as behaviour and lifestyle (e.g., level of physical activity, smoking habits, weight), socio-economic conditions, and genetic characteristics (e.g. omics data, molecular biomarkers). In order for these datasets to be used for decision making in healthcare, challenges regarding analytics and visualization need to be addressed. Dashboarding is a data visualization technique that incorporates the variety of data visualizations for the myriad of datasets in a flexible and adaptive way for different end-users (with different needs from a usability perspective).
While considering the dashboarding solution, we have two main aspects. First, the analytics algorithms used to make use and sense of the datasets and, secondly, the cognitive aspect from the user-perspective, to interpret the data displayed. However, most solutions in the market are developed solely from the analytics engineering and systems design. My thesis research aims to study data visualization with a human-factors approach. The main objective of my research is to develop the guidelines to design dashboards for complex datasets. Using precision medicine as a study case, my study aims to: understand what data visualization types are being used for precision medicine through a scoping review; identify what are the key concepts to be visualized in the precision medicine context, through a thematic analysis; and, create guidelines for knowledge translation through prototyping and running usability testing on data visualization platforms.
In the context of complexity, such as precision medicine, data and information to be assessed and shown represent highly complex knowledge with equally complex relations between them. It is believed that to assess the interactions between datasets may unveil knowledge yet untapped. Best practices of information visualizations methods and techniques may exist within the precision medicine context in order to make data interpretation and knowledge translation more efficient amongst its users (caregivers, patients and more). These practices need to be considered and improved in this new domain with integrated data and expanded knowledge.
Project members:
Tatiana Silva Bevilacqua, PhD
Last updated: April 06, 2021