Providing powerful forecasting tools for virtual power plants
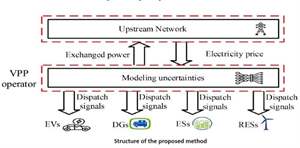
Today’s electricity system includes more and more smaller-scale sources of energy, plus more and more electric vehicles (EVs) that act as small-scale storage systems when they’re plugged in. Virtual power plants (VPPs) help manage all those “distributed energy resources” by pooling them into larger packages that can be included in day-ahead electricity markets.
In those markets, participants buy and sell electricity at binding prices for the following day. But making accurate bids can be challenging for VPP operators, as variable factors like wind speed and what time EV owners plug in their vehicles create lots of uncertainty. That means current forecasting models for VPPs are often off by more than 10 percent.
So WISE researchers Kumaraswamy Ponnambalam and Ali Elkamel and their colleagues set out to create a better approach. Using mixed-integer linear programming, they developed an optimized VPP model that considers load flow, emission costs, and a wide range of distributed energy resources.
The researchers also developed a highly accurate method for forecasting uncertainties, based on a deep-learning neural network. Next, they supplied their model with data on historical electricity loads and prices, wind speed, and EV usage.
They then tested it by simulating a VPP consisting of two wind turbines, 200 connected EVs, and other distributed generation and storage systems. Impressively, the resulting forecasts had an average error rate of just 1.47 percent — a huge improvement over other methods. And with that improved accuracy, VPP operators can now get more from their distributed energy resources.
Researchers: Kumaraswamy Ponnambalam, Ali Elkamel, Ali Ahmadian, Ali Almansoori
Source: Ahmadian, A., Ponnambalam, K., Almansoori, A., & Elkamel, A. (2023). Optimal Management of a Virtual Power Plant Consisting of Renewable Energy Resources and Electric Vehicles Using Mixed‑Integer Linear Programming and Deep Learning. Energies, 16(1000), 1-17. https://doi.org/10.3390/en16021000