Learn more about some of the artificial intelligence-related research undertaken at the University of Waterloo:
Deep Learning for Driver's Behaviour Recognition
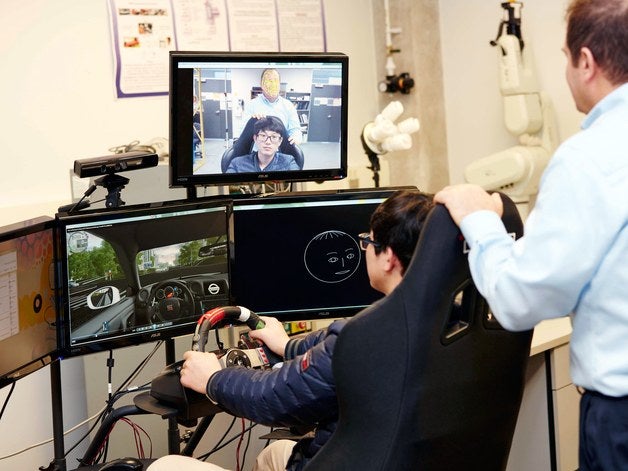
Centre for Pattern Analysis and Machine Intelligence
Researchers in the Centre for Pattern Analysis and Machine Intelligence have developed a new algorithm for predicting and alerting in real time, the behaviour and status of the driver of a vehicle. This solution can operate via a connection to the cloud, or on a stand-alone basis. It will contribute to the design of "level-4" automation in autonomous vehicles. This development has been featured in media including the Washington Post, DigitalTrend, Wired, CBC, Vice Media, Globe and Mail, and Daily Mail.
The "Tricorder"
Vision and Image Processing Lab
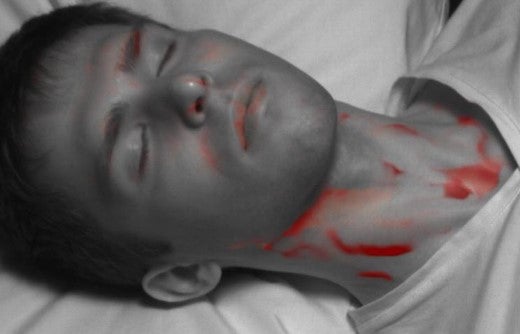
Waterloo’s Vision and Image Processing Lab has developed a revolutionary "tricorder" technology, termed Coded Hemodynamic Imaging (CHI), that is the first-of-its-kind to enable touchless imaging of blood flow across the body, and which could lead to improved detection and prevention of a wide range of heart and lung diseases. Current techniques for diagnosing heart failure rely on invasive catheterization to obtain a single-point jugular venous pulse measurement, but CHI enables non-contact imaging of blood flow across entire jugular veins, as one might measure traffic flow across a city. CHI projects light and captures light fluctuations on the skin surface, and relays them to a digital signal processing unit which computes blood-flow patterns. This research was ranked in the Top 5% of all research outputs scored by Altmetric, and received media coverage across the globe, with an appearance on the popular science show Daily Planet on the Discovery Channel.
Conversational Agents
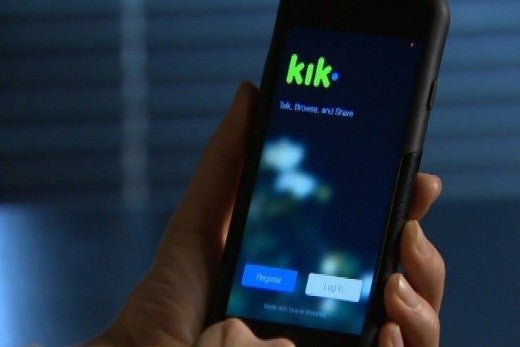
Text messaging applications have become the most popular and most engaging features of smart phones in recent years. In collaboration with Kik Interactive, Artificial Intelligence researchers at the University of Waterloo have developed adaptive and emotionally aware conversational agents for text messaging. Leveraging machine learning and affect control theory, the algorithms automatically adapt to the affective personality of users by recognizing their emotions in short text messages and responding with messages that are emotionally aligned. This work has already led to two patents on adaptive and emotionally aware conversational agents.
Functionally-safe automotive autonomy
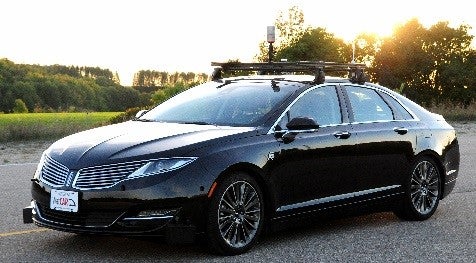
In November 2016, Waterloo’s ‘Autonomoose’ became the first autonomous test vehicle licensed to operate on Canada’s public roads. The fleet will grow in 2017 with multimillion dollar support from NSERC, CFI, the Province of Ontario and several private sector partners. The team’s machine learning approach targets perception and prediction in adverse conditions – tackling the complexities of snow, sleet, low light, and reflective surfaces. A major practical focus is safety assurance – efficiently validating and certifying the safety of machine learning algorithms so we can incorporate AI into safety critical functions.
Resource sharing for better control of forest fires
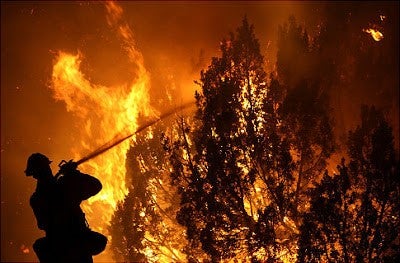
Forest fires threaten communities across North America, cost billions of dollars annually, and destroy vast quantities of natural resources. But controlling fires is expensive and local resource demand often outstrips availability. Researchers from Waterloo’s Artificial Intelligence Group have built the first model for wildfire fire resource sharing, using ideas from multi-agent systems and game theory. Developed in partnership with the Ontario Ministry of Natural Resources and in consultation with experts in the field, their analysis has identified the key strategic issues confronting fire-response agencies, and opens up a valuable new application area for the Artificial Intelligence community.
Evolutionary deep intelligence
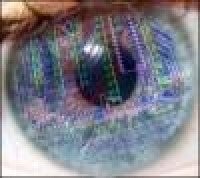
Vision and Image Processing Lab
Researchers in Waterloo’s Vision and Image Processing Lab have developed new techniques to obtain highly efficient deep neural network architectures. Their evolutionary deep intelligence approach drives the formation, over successive generations, of highly sparse synaptic clusters. The offspring algorithms, trained on image classification, can achieve state-of-the-art performance despite an up to 125-fold decrease in synapses. Such architectures are ideal for low-power embedded CPUs. This research received the best paper award at the efficient deep learning workshop, held at the NIPS Conference in Barcelona, December 2016. A spinoff company, DarwinAI, is aiming to accelerate operational AI from the edge to the cloud for all verticals.
Identifying cancer through imaging
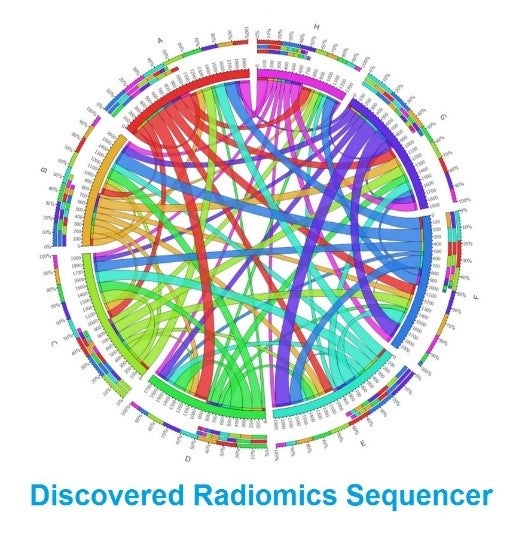
Vision and Image Processing Lab
A team from the Vision and Image Processing Lab was honoured with two Magnum Cum Laude awards at the 2016 Annual Meeting of the Imaging Network of Ontario for their work in discovery radiomics, in collaboration with Sunnybrook Health Sciences clinicians. They developed a breakthrough strategy for quicker and more effective cancer identification based on the quantitative identification of tumour biomarkers, tapping into the wealth of information contained within medical imaging archives. The image to the right shows the discovered sequencer, encoding the cancer biomarker data.
Embedded speech engines
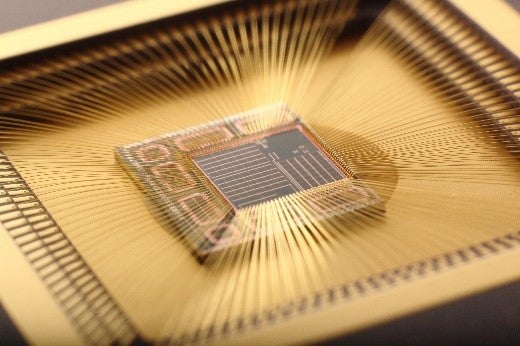
Centre for Pattern Analysis and Machine Intelligence
The Centre for Pattern Analysis and Machine Intelligence has developed a set of AI-based platforms that outperform standard keyword spotting techniques, both theoretically and experimentally. These embedded speech devices, untethered to any cloud-based speech engine, are based on deep learning algorithms and advanced noise suppression techniques; an embedded Natural Language Understanding version of the system is also being tested. A spinoff company, Cognitive Computing Technologies, is targeting automotive environments, cognitive/social robotics, and small office/home appliances.
Autonomy in human-robot teams
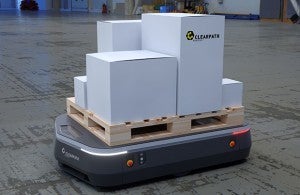
As robots move beyond the production line and into shared human environments, efficient and effective communication with human operators will be essential. In a three-year collaboration initiated with Clearpath Robotics, researchers from Waterloo’s Adaptive Systems and Autonomous Vehicles labs will develop machine learning strategies for fully autonomous warehouse robots to automatically elicit human preferences via simple interactions with staff, with no need for expert programming and supervision. Clearpath is one of several robotics companies scaling up in Waterloo Region - founded in 2009 by Waterloo engineering students, the company now employs over 150 people.
Assistive technologies for the elderly
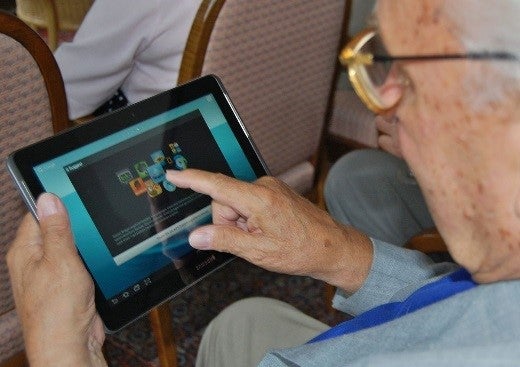
Computational Health Informatics Lab
Waterloo’s Computational Health Informatics Lab are developing technologies to assist persons with cognitive disabilities. The ACT@HOME project is building an emotionally intelligent cognitive assistant to engage and help older adults with Alzheimer's disease to lead more independent and active daily lives. The Do-It-Yourself Smarthome project is connecting end users with developers by building a person-specific logical knowledge base of user needs, assistance dynamics, sensors, actuators and care solutions. These programs are conducted in conjunction with the AGEWELL Inc. Network of Centres of Excellence, a $35 million dollar initiative that includes 29 research centres across Canada and over 110 industry, government and non-profit partners.
Living architecture systems
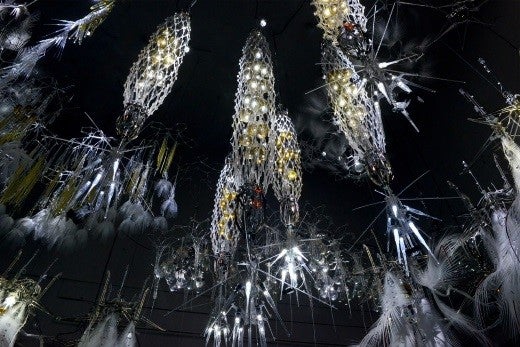
Waterloo Architecture
Waterloo is leading a new $2.5M SSHRC Partnership to explore living architecture that uses curiosity-based reinforcement learning algorithms to continuously generate novel behaviours. With over 40 collaborators across North America, Europe and Asia, the School of Architecture will team up with researchers in the Electrical and Computer Engineering and Knowledge Integration departments to explore ways to generate genuine and sustainable social interaction with autonomous systems, and design buildings that can continuously adapt to create a cleaner and healthier environment.
RoboHub in Building E7
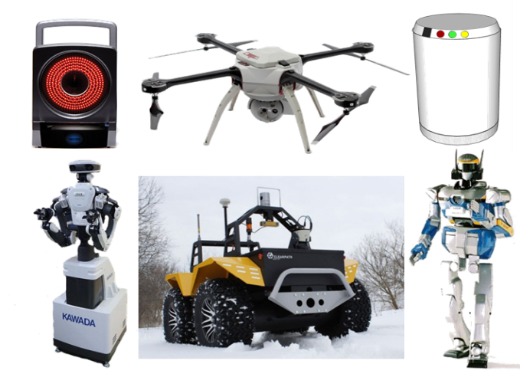
The $4.5M RoboHub, supported by CFI, will provide the only multi-robot test facility in the world with autonomous ground, aerial, humanoid and magnetically levitated platforms under one roof. Located at the heart of the new Engineering 7 building, the RoboHub will support a host of research programs into robot co-ordination and autonomy. For example, Bayesian learning models will enable state estimation for localization and autonomous mapping (SLAM) of intelligent mobile robotics agents and unmanned aerial vehicles (UAVs) to enable navigation in unstructured environments. Meanwhile, recurrent neural networks will enhance quadrotor flight modelling, predicting aerodynamics with greater accuracy for precision manoeuvring at speed and near obstacles.
Hockey analytics
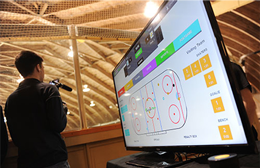
Hockey is a fast-paced game for which there is increasing demand for data analytics. In partnership with HockeyTech, researchers in Artificial Intelligence and Economics at the University of Waterloo are developing automated algorithms that process low-level position data about each player and the puck and which can recognize events such as shots, passes, puck possession, takeaways, giveaways, and faceoff wins, and then use this data to predict goals, wins and player performance. These new statistics are helping players, coaches, scouts and fans of professional and semi-professional hockey leagues across North America.
Barcoded Medical Imaging
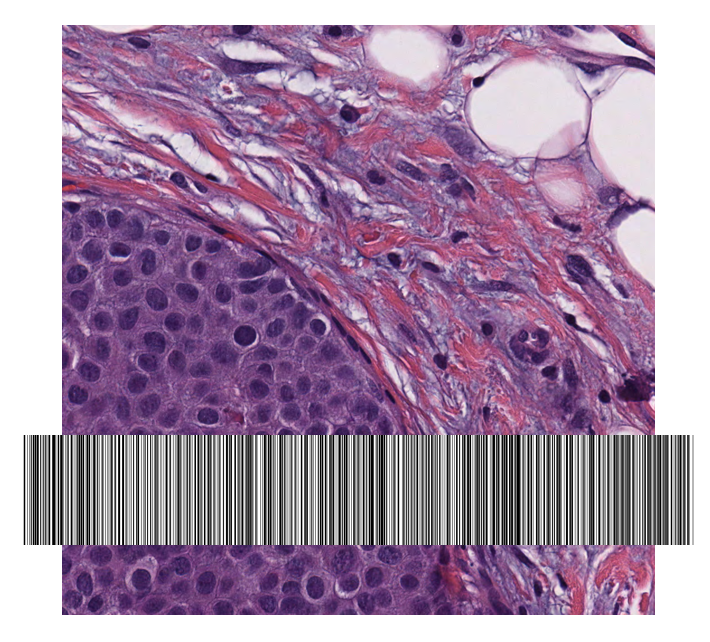
Kimia Lab members have been working on medical images and artificial neural networks for many years. The lab's research focuses on generation of “deep barcodes” for medical images that uniquely represent that image, e.g. a pathology scan, to facilitate searching for similar images. Similar cases retrieved and displayed to clinical experts (e.g., radiologists, pathologists) along with biopsy reports and treatment results can indeed immensely reduce diagnostic error; Training a deep neural network with a large number of images is a multifaceted challenge if there is not enough data, the data is imbalanced, or most of it is not labelled. Researchers in the Kimia lab work on extensions and improvements of several AI and computer vision technologies to enable image recognition in digital pathology.
Spaun
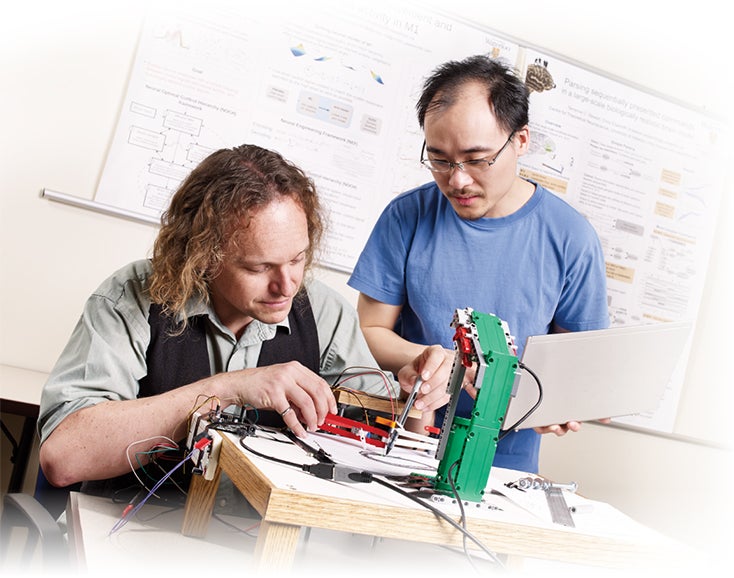
Centre for Theoretical Neuroscience
Meet Spaun, the world’s largest model of a functional brain. Developed by Chris Eliasmith and his multidisciplinary team at Waterloo’s Centre for Theoretical Neuroscience (CTN), Spaun combines 2.5 million simulated neurons with a visual recognition system and a simulated mechanical arm. Just like its human counterpart, it can read, answer questions, play simple games and memorize lists. It can decode numbers written in unfamiliar handwriting and tackle basic logic problems. Like people, it even makes mistakes, faltering at complex questions or getting tripped up when lists get too long. And Spaun learns, adapting its behaviour based on feedback from the world around it.
Machine Learning, SAT/SMT Solvers, and Deduction
Read more about MapleSAT here.
Since the early part of this century we have witnessed the amazing rise of machine
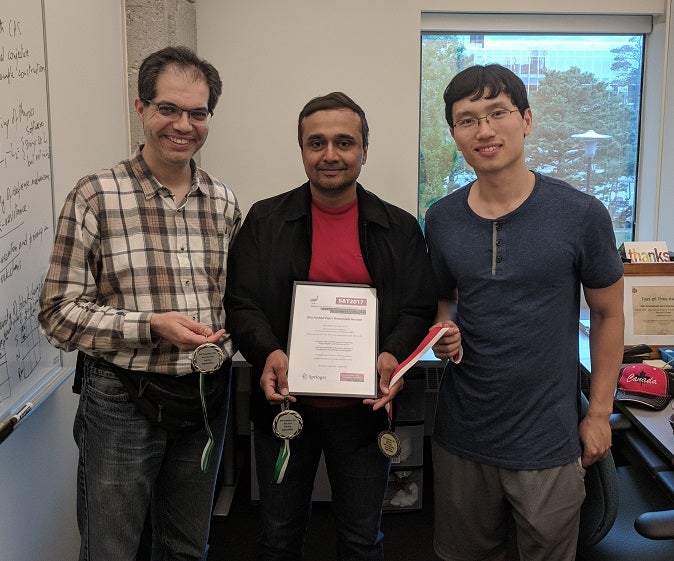
learning methods in solving problems that were once considered near-impossible. Contemporaneously with the rise of machine learning, we are witnessing a quieter revolution in another part of AI, namely, logical deduction. Logical deduction engines such as SAT/SMT solvers are tools that are aimed at solving mathematical equations. These tools are increasingly being used in solving all manner of software engineering, security, and verification problems that were once deemed impossible. However, for most of their history, these two areas of AI, namely, machine learning and logical deduction, developed separately with little cross-pollination of ideas.
Two researchers at the University of Waterloo, Dr. Vijay Ganesh and Dr. Pascal Poupart, have been working to bring machine learning and logical deduction together in ways that positively impacts both. Jointly with their student, Jia Hui (Jimmy) Liang, they developed a set of machine learning methods to guide the "search for proofs" that logical deduction engines perform in order to solve mathematical equations. They showed for the first time that machine learning methods, such as reinforcement learning, are very effective in helping logical deduction engines find "short proofs" that otherwise would be very challenging to find. The result has been the MapleSAT solver, that won gold and silver medals at the highly competitive SAT competition 2016 and 2017, and is currently one of the leading solvers in academia and industry.
We are witnessing a new revolution that brings together inductive machine learning and deductive logic methods to dramatically enhance the efficiency of these logic engines in solving problems that were once deemed impossible.