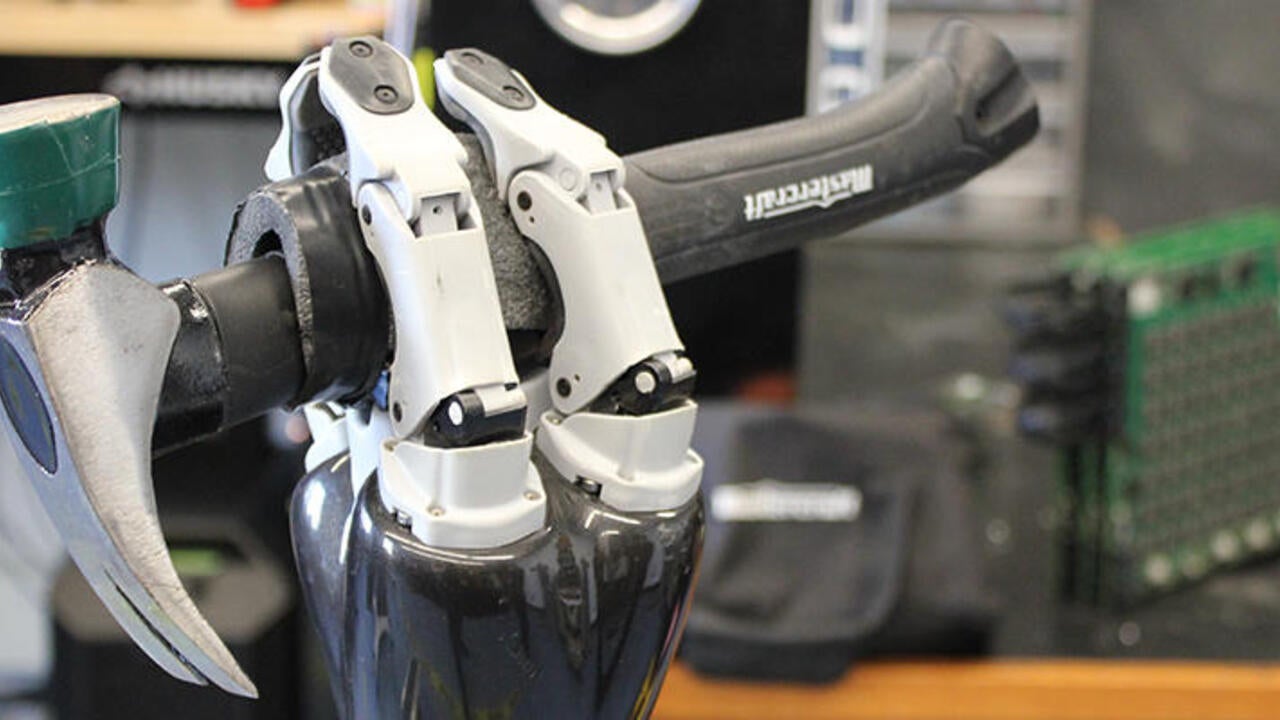
AI with a difference
The keys to operational AI are maximizing efficiency and minimizing required resources.
The keys to operational AI are maximizing efficiency and minimizing required resources.
By Brian Caldwell Faculty of EngineeringAlexander Wong is explaining the concept at the core of his groundbreaking artificial intelligence (AI) research when he realizes a comparison would probably help.
But it isn’t in computer science or computer engineering that the Waterloo systems design engineering professor and alumnus finds a useful analogy to convey how the process of progressively compacting expansive deep neural networks to fit on small computer chips basically works.
His practical example is the Mexican blind cavefish, which has endured over time in a dark, underground environment by gradually shedding both its eyes and the area of the brain that normally controls vision. With food and oxygen in short supply, scientists theorize, the blind, colourless fish has adapted by sacrificing a sense that was of limited use to gain crucial energy savings of about 15 per cent.
“That’s what it needed to survive,” says Wong, impressed by the efficiency of the evolutionary forces involved.
Similar forces are at work in “evolutionary deep intelligence,” one of many promising areas of AI that Waterloo Engineering researchers are pursuing in fields including medicine, robotics, self-driving vehicles, speech recognition and living architecture.
In effect, Wong and doctoral student Mohammad Javad Shafiee discovered that large deep neural networks — powerful programs with layers and layers of processing units at the heart of the most prominent kind of AI — will find efficiencies and shrink themselves when denied resources in their numerical environments.
Done repeatedly, generation after generation, they have used the process to produce deep neural networks over 100 times smaller than their originals while maintaining similar performance for particular functions. The results are compressed deep neural networks — essentially complex computer programs designed to mimic the workings of neurons and synapses in the human brain — able to fit on computer chips the size of a coin or smaller.
Significantly, those chips can then be built right into devices and machines rather than requiring connections to enormous cloud-based computing power to provide their intelligence.
“There are certain tasks for which the ‘brain’ they create, this large AI, is overkill,” says Wong, who co-directs the Vision and Image Processing (VIP) Lab and is a Canada Research Chair in medical imaging systems. “There are many places where we can slim down, so to speak.”
Leaner. Smaller. Less expensive. More accessible.
Those are the goals pursued by Wong and numerous colleagues across multiple disciplines within Waterloo Engineering, plus key collaborators from computer science, in a branch of AI they call “operational AI.”
The keys to operational AI, as they were to the Mexican blind cavefish, are maximizing efficiency and minimizing required resources.
“It’s application of AI for the masses, if you like,” says Fakhri Karray, an electrical and computer engineering professor. “It should be available everywhere to almost everyone, giving access to as many people as possible.”
Much of the current buzz about AI as technology that will dramatically change the world concerns the use of deep neural networks, or artificial neural networks, to achieve what is known as deep learning.
Technology giants including Google, Microsoft and Amazon are investing heavily in research to produce super-human performance by increasing the layers of algorithms and the computing power needed to process massive amounts of data.
Their efforts have yielded impressive results such as AlphaGo, a computer program that exceeded expectations and defeated a top professional last year at Go, a strategy board game that was invented in China over 2,500 years ago and is more complex than chess.
The federal Liberal government of Prime Minister Justin Trudeau, meanwhile, demonstrated its belief in the importance of AI to the future by committing $125 million in its March 2017 budget to create a Pan-Canadian Artificial Intelligence Strategy.
...with our approach, you democratize AI in a way that makes it accessible to everybody and affordable for everybody.
- Willem Melek
Despite its awesome potential, however, experts say the use of this deep learning AI is limited in many situations by cost, computational complexity and other factors.
Operational AI, the practical approach taken at Waterloo Engineering to complement fundamental, foundational research in the field, is focused on overcoming those limitations by developing stand-alone systems tailored to specific applications.
William Melek, a professor and director of mechatronics engineering at Waterloo, says most small and mid-sized businesses, for instance, “see the promise” of AI, but are daunted by its imagined price tag and need for highly trained employees to operate it.
“They think it’s not affordable, not within their reach,” he says. “But with our approach, you democratize AI in a way that makes it accessible to everybody and affordable for everybody.”
Central to the solution for many forms of the technology is distilling AI down until what is needed to do the job can fit on a chip and be implanted, or embedded, within devices and machines, eliminating the need for a connection to powerful servers or cloud computing networks.
Other variations of operational AI still utilize the Internet for reasons including lower upfront costs and convenience, but do not require huge computational resources to efficiently perform their particular tasks.
The evolutionary approach taken by Wong achieves its goal by shrinking or compacting deep neural networks to their essential elements.
“Despite the fact we don’t have access to these giant clouds, we’re still able to produce artificial intelligence that performs similarly, just as well, or close to just as well, but with much more constrained operational requirements,” he says.
At the Waterloo Engineering Bionics Lab, researchers led by Ning Jiang are using self-contained operational AI to tackle medical challenges involving Parkinson’s disease, stroke rehabilitation, autism, manipulation of artificial limbs and phantom pain for amputees via human-machine interface.
At the heart of the technology are algorithms — which Jiang helped develop while working in Germany before coming to Waterloo two years ago — capable of identifying and interpreting electrical signals in the brain picked up by electrodes on the scalp.
“We can detect that you want to move your toe, for example, even before the toe moves,” says Jiang, a systems design engineering professor and director of the Waterloo Engineering Bionics Lab.
To demonstrate how the software works, he writes an ‘A’ in the middle of a piece of paper to represent the signal of interest, then crumples it into a tight ball. The ‘A,’ of course, can’t be seen.
Jiang then uncrumples the paper and smooths it out as best he can. Although it is creased and crinkled, the ‘A’ is easy to spot and read.
Jan Lau’s modular exoskeleton design to help people with Parkinson’s disease who freeze when walking.
In effect, he says, that’s what the algorithms do — smooth out a dense mass of data on brain activity so the signal of interest, such as the desire to wiggle a toe, can be pinpointed using relatively small computers.
Applications of that breakthrough now being pursued in his lab include an exoskeleton for Parkinson’s patients who freeze while walking. Brain signals would be detected, read and sent to a small computer, which would then activate the mechanical exoskeleton to start a step.
“I’m developing something to help people improve their independence,” says Jan Lau, a second-year biomedical engineering student who is working on the project. “That’s what really fires me.”
Another application being developed is a mind-control robotic hand. Although there are several multi-function prosthetic hands currently on the market, the one Jiang’s lab is working on involves a new direction on dexterous myoelectric control mimicking the neuromuscular system.
The goal is to develop algorithms and control strategies that would offer amputees intuitive control of the sophisticated prostheses providing them with greater autonomy and better quality of life.
A second approach to operational AI at Waterloo utilizes powerful machine learning algorithms that are accessible on the cloud to process and mine knowledge from reams of data.
That knowledge can then be used in the design of smart devices and machines, directly equipping them with deeper AI technology.
Chaojie Ou (left), a doctoral candidate, and Fakhri Karray, work together developing AI technology for driver behaviour recognition and human-car cognitive score assessment
As an example in this category, Karray cites a system to alert drivers in danger of falling asleep at the wheel based on the frequency of their blinking and other physical cues read by an in-car camera.
Accurately pinpointing the warning signs requires analysis of huge amounts of data via complex learning systems. Once it is known, however, software imparted with that knowledge need only be tailored, not built again from scratch, to intelligently execute a particular function.
By combining existing human-machine interaction technologies, cameras and alert mechanisms, in fact, several teams of graduating students developed such systems for their Capstone Design projects at Waterloo this year.
“This is operational AI,” says Karray, who is also a University Research Chair and director of the Centre for Pattern Analysis and Machine Intelligence (CPAMI). “You don’t need hundreds of scientists at Microsoft or Google to develop the biggest algorithm on earth. Simply impart the extracted knowledge into embedded devices and you are able to produce the smartest machine possible.”
In addition to dramatically cutting costs and complexity, severing the connection to the cloud with built-in, self-contained AI systems also deals with concerns around reliability, security and confidentiality in several areas of application.
With its ongoing, advanced fundamental research complemented by such a wide variety of practical applications and deployments, Melek says Waterloo Engineering is well-positioned to stake a claim at the forefront of the AI revolution.
“We have it covered from one end of the spectrum to the other — end-to-end, as we like to call it,” he says.
Read more
The Games Institute brings together a diverse community of artists, engineers, writers and scientists to collaborate on research projects
Read more
Waterloo researchers are testing innovative new AI and machine learning technologies to address Canada’s pilot shortage
Read more
Nanotech startup raises $6.5 million USD seed funding to produce its novel coating for biodegradable materials at scale
The University of Waterloo acknowledges that much of our work takes place on the traditional territory of the Neutral, Anishinaabeg and Haudenosaunee peoples. Our main campus is situated on the Haldimand Tract, the land granted to the Six Nations that includes six miles on each side of the Grand River. Our active work toward reconciliation takes place across our campuses through research, learning, teaching, and community building, and is co-ordinated within the Office of Indigenous Relations.