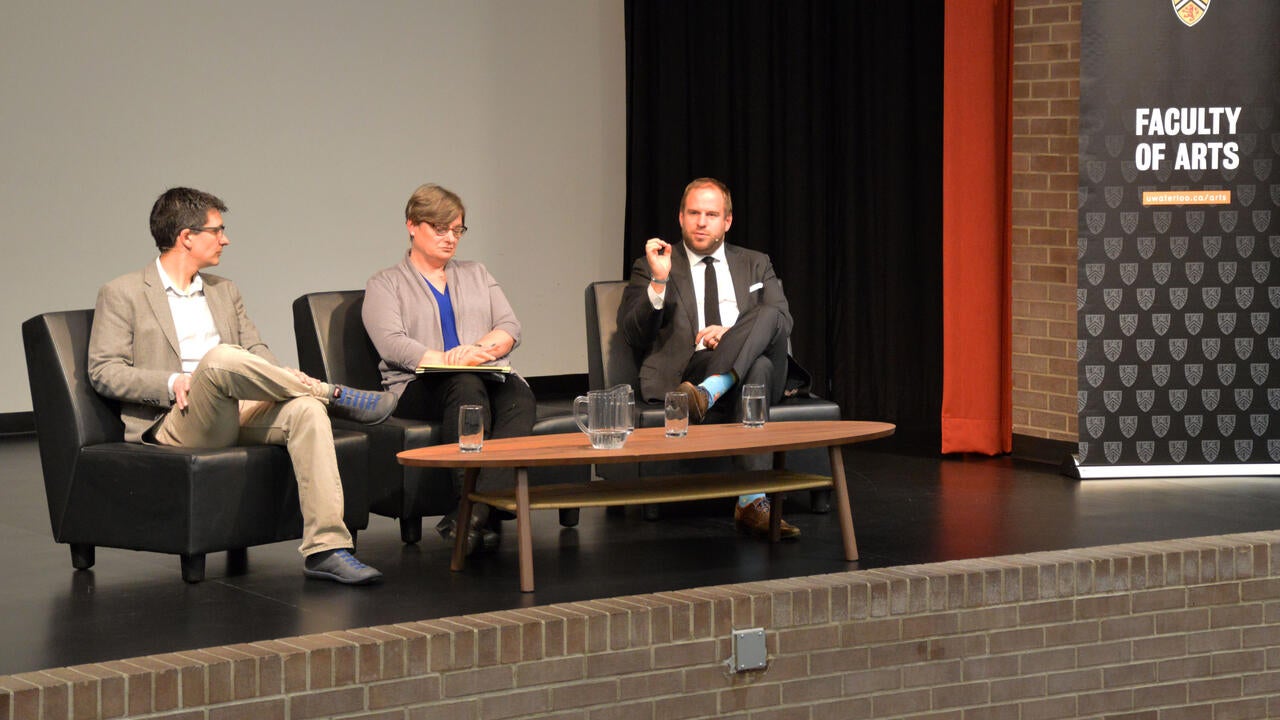
Keeping the human in Artificial Intelligence
AI needs diverse human perspectives to ensure everyone benefits from technology, Waterloo experts say
AI needs diverse human perspectives to ensure everyone benefits from technology, Waterloo experts say
By Rose Simone University RelationsThe power of artificial intelligence is already permeating throughout our work and social lives.
But as artificial intelligence (AI) systems “learn” from millions of interactions or case examples, it also has the potential to be disruptive, said experts from the University of Waterloo during a panel discussion on ‘Keeping the Human in AI’ at the Kitchener Public Library last week.
The professors with expertise in economics, philosophy, and human-computer interaction discussed the implications and how to mitigate against the dangers at the talk and during interviews.
Like the steam engine, electricity or semiconductors, AI is a “general purpose technology,” with far-reaching implications, says Joel Blit, a Waterloo economics professor who co-wrote a policy paper on Automation and the Future of Work for the Centre for International Governance Innovation.
“I absolutely think this is going to change the way our economy is organized, the way jobs are done and who gets what wages,” Blit says.
Along with the huge increase in computing power, our digital era also generates massive data sets that the AI systems learn from. These data sets are now being used to teach AI systems how to recognize faces, understand natural language, and even play chess. They can assess political inclinations from Facebook posts, spot criminals from faces in a crowd or guess a person’s emotional state from their facial expressions.
Blit says in many cases, the AI will automate certain tasks, but not completely replace the job. An AI can help spot a tumor on a radiology image, but human radiologists need to verify that diagnosis and consult with physicians about the results and treatment options.
But overall, as with any type of automation, it allows jobs to be done with fewer people. Blit points out that a new company that emerges on the AI landscape might generate billions of dollars in value and revenue, but only have 50 employees. That has already been true of a number of companies in the digital era, such as YouTube, Instagram and WhatsApp, all of which were sold for billions but only had handfuls of employees to share in that wealth.
In the long run, AI can improve efficiency and bring down costs of some types of services, which in turn can increase the demand for those services. Also, as with any technological revolution, new applications for the tools will be found that will create jobs, Blit says.
The problem is that there is a transition period, Blit adds. He gives the example of the Industrial Revolution that started around 1770 but it took about 50 years before overall wages started steadily rising.
But the bigger worry is the potential for economic disruption leading to greater inequality and political instability, Blit says.
He stresses the need for policies that encourage entrepreneurship and gear education toward fostering leadership, empathy, communication, creativity and critical thinking skills so that young people will be flexible and ready for the jobs that will still exist in the future.
Yet economic disruption is just one type of impact that AI will have. Other experts, such as Carla Fehr, a Waterloo feminist philosopher and the Wolfe Chair in Scientific and Technological Literacy, says it can also amplify prejudices and biases in a society.
Fehr says even though people think of machines as objective, the data sets that train AI systems can have built-in biases. “Human beings are really bad at recognizing their own biases,” she adds.
She gives the example of the work of Joy Buolamwini, an African-American computer scientist at the M.I.T. Media Lab who experienced the bias of facial recognition software firsthand.
The facial recognition software didn’t work on her face, even though it worked on her white friends. At first, she thought this was a flaw that would soon be fixed, but she kept running into the problem. She could only get the system to recognize her as a human when she wore a white mask. She conducted a study that found that, in fact, commercial face recognition software was bad at identifying women with darker skin tones. The error rate was as high as 46 per cent, almost no better than random coin toss, Fehr says.
Since then, Boulamwini found problems in Microsoft, IBM, and Face++ systems. She collaborated with a Microsoft scientist while conducting her research, and Microsoft and IBM have said that they are addressing this issue, while Face ++ has not responded. But to Fehr, what is interesting is that companies developing and using the software had not previously noticed this built-in bias.
That speaks to the critical importance of having diversity in research and development, Fehr adds. “It is not a coincidence that it took a computer scientist who was politically active and a black woman to figure this out.”
There are situations where built-in AI biases can do a lot of harm, Fehr adds. A good example was when Amazon discovered that the AI system it used to sort through employee applications tended to favour of male applicants. To its credit, Amazon stopped using the system, but such biases can also affect many other types of AI systems used in areas such as law enforcement or insurance, she says.
“We know these biases prevail in our culture, so we shouldn’t be surprised to find them in AI systems,” Fehr says.
It’s not only important from a human rights perspective to mitigate against biases in software, it is also important for accuracy in research and it makes good business sense, given that white men only represent about 40 per cent of the population in North America, she says.
Lennart Nacke, a Waterloo professor with expertise in user experience design at the Stratford School of Interaction Design & Business, says with more users, and more diverse users to learn from, an AI system gets better at what it does.
Artificial intelligence is Software 2.0 that can “optimize itself” instead of having the improvements programmed into it, Nacke says.
But for AI to improve, it needs lots of people who engage with it. That’s where user design comes in. Gamification, which involves using game-like features to engage people in fitness apps for smartphones for example, can be applied to keep people contributing to various AI applications as well, he adds. But just as a child can learn bad behaviour from bad examples, the same is true for AI. Within 24 hours of Microsoft releasing a chatbot named Tay on Twitter, with the intent of improving the AI system’s understanding of conversational language, it had to be taken down because people were tweeting misogynistic, homophobic and racist slurs, and so the chatbot started parroting that type of foul language back to people, Nacke says.
Also, while the goal is to make AI systems engaging, the other problem is that they can be so human-like, that people will unwittingly provide personal information to the system.
Nacke gives the example of some colleagues who sent a cute smiling robot out to ask people questions. In almost all the cases, people were happy to answer the friendly robot’s questions. But with that information, along with what it could find about the individual on Google, the robot was able to produce a shocking amount of data about those individuals.
“It is up to the makers of the software to not trick humans by emulating behaviours that will cause people to let their guard down, thinking it is a harmless environment,” he says.
The experts at the talk said the lesson is that AI cannot just be left to its own devices, or to the whims of a marketplace. As Fehr says, “an important role for humans will always be to make sure that we hold the people who create and market our AI systems responsible and that we pay attention to issues of justice across the board.”
Read more
Waterloo research group joins global partnership on AI
Read more
Waterloo faculty and alumni featured at Canada's largest technology festival.
Read more
Combining machine learning techniques with real-world scenarios to combat forest fires
The University of Waterloo acknowledges that much of our work takes place on the traditional territory of the Neutral, Anishinaabeg, and Haudenosaunee peoples. Our main campus is situated on the Haldimand Tract, the land granted to the Six Nations that includes six miles on each side of the Grand River. Our active work toward reconciliation takes place across our campuses through research, learning, teaching, and community building, and is co-ordinated within the Office of Indigenous Relations.