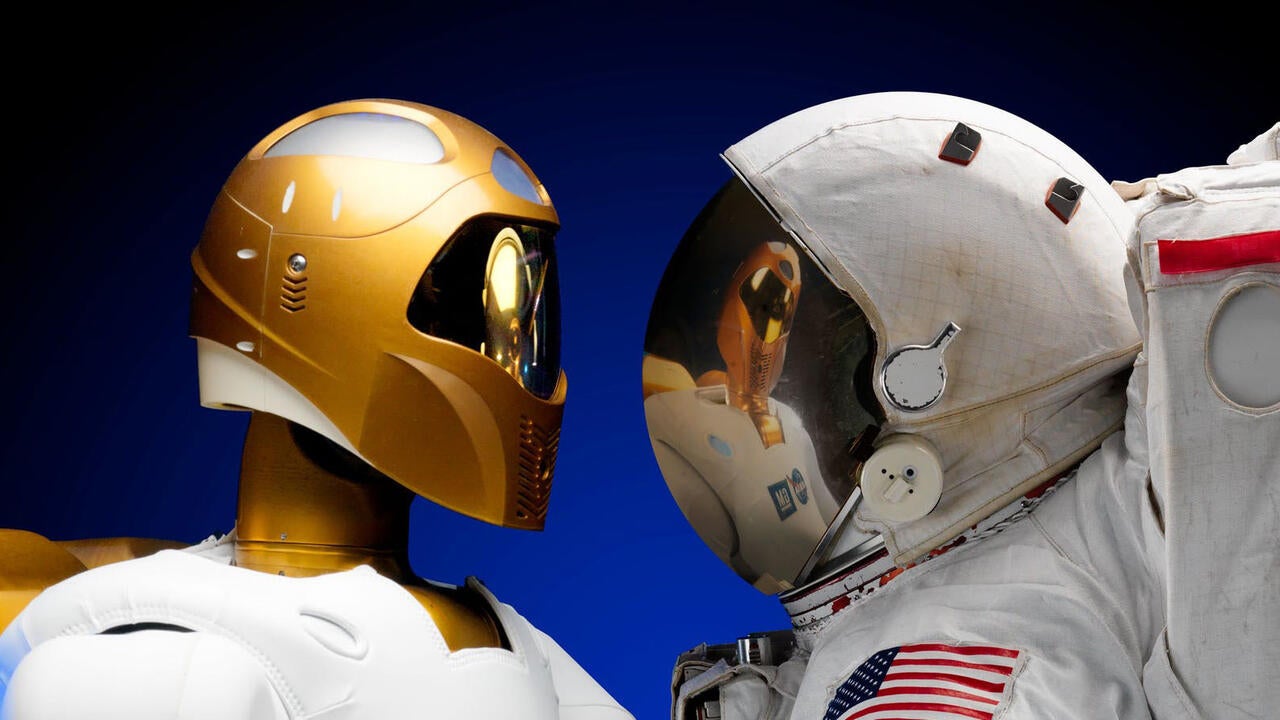
Breaking free of the internet with stand-alone AI
Taking a page from nature and applied it to making artificial intelligence (AI) more efficient
Taking a page from nature and applied it to making artificial intelligence (AI) more efficient
By Brian Caldwell Faculty of EngineeringAlexander Wong and Mohammad Javad Shafiee weren’t sure it would work when they took a page from nature and applied it to making artificial intelligence (AI) more efficient.
Three years of research and refinement later, however, the Waterloo Engineering colleagues are confident their innovative approach is paving the way for powerful, stand-alone AI that is so compact it can break free of the internet.
“We feel this has enormous potential,” says Wong, a systems design engineering professor, Canada Research Chair and director of the Vision and Image Processing (VIP) Lab at Waterloo. “This could be an enabler in many fields where people are struggling to get deep-learning AI in an operational form.”
Deep-learning AI software, which mimics the workings of the human brain by processing data through layers and layers of artificial neurons, typically requires considerable computational power, memory and energy to function.
In an effort to improve its efficiency, Wong and Shafiee hit on a strategy to place AI in a virtual environment, then progressively and repeatedly deprive it of resources in that environment.
Their theory – that AI neural networks might change and adapt in the same way organisms respond to evolutionary forces in nature – worked like a charm.
In research presented at the International Conference on Computer Vision in Venice, Italy in the fall, they achieved a 200-fold reduction in the size of deep-learning AI software used for a particular object recognition task.
“These networks evolve themselves through generations and make themselves smaller to be able to survive in these environments,” says Shafiee, a systems design engineering research professor at the VIP Lab.
Technology created by Wong and Shafiee can produce deep-learning AI software that is compact enough to fit on mobile chips for use in everything from smartphones to industrial robots.
Significantly, that means those devices could operate independent of the internet and cloud-based computing resources while still using AI that performs almost as well as tethered neural networks.
Such stand-alone deep-learning AI could lead to much lower data processing and transmission costs, greater privacy in sensitive fields including health and security, and use in areas where existing AI technology is impractical due to the expense and other factors.
“There are many tasks for which you can’t stream raw data to the cloud,” says Wong. “There is just too much of it.”
When put on a mobile chip and embedded in a smartphone, for example, compact AI could run its speech-activated assistant and other intelligent features, greatly reducing data usage and continuing to function even without internet service.
Other potential applications range from use in low-cost drones and smart grids, to surveillance cameras and robots in manufacturing plants, where there are major issues around streaming sensitive or proprietary data to the cloud.
Wong and Shafiee have co-founded a company called DarwinAI to commercialize their efficient AI software.
“We are researchers, so we explore many different things,” Shafiee says of their evolutionary approach. “And if it works, we keep going and push harder.”
“This one has worked beyond our expectations,” adds Wong. “We were amazed, actually.”
Read more
Nanotech startup raises $6.5 million USD seed funding to produce its novel coating for biodegradable materials at scale
Read more
New study from Waterloo researchers creating climate change-proof buildings with deep learning-powered inspections
Read more
The University is partnering with HI3 Hub to improve wastewater detection and analysis of pathogens
Read
Engineering stories
Visit
Waterloo Engineering home
Contact
Waterloo Engineering
The University of Waterloo acknowledges that much of our work takes place on the traditional territory of the Neutral, Anishinaabeg and Haudenosaunee peoples. Our main campus is situated on the Haldimand Tract, the land granted to the Six Nations that includes six miles on each side of the Grand River. Our active work toward reconciliation takes place across our campuses through research, learning, teaching, and community building, and is co-ordinated within the Office of Indigenous Relations.