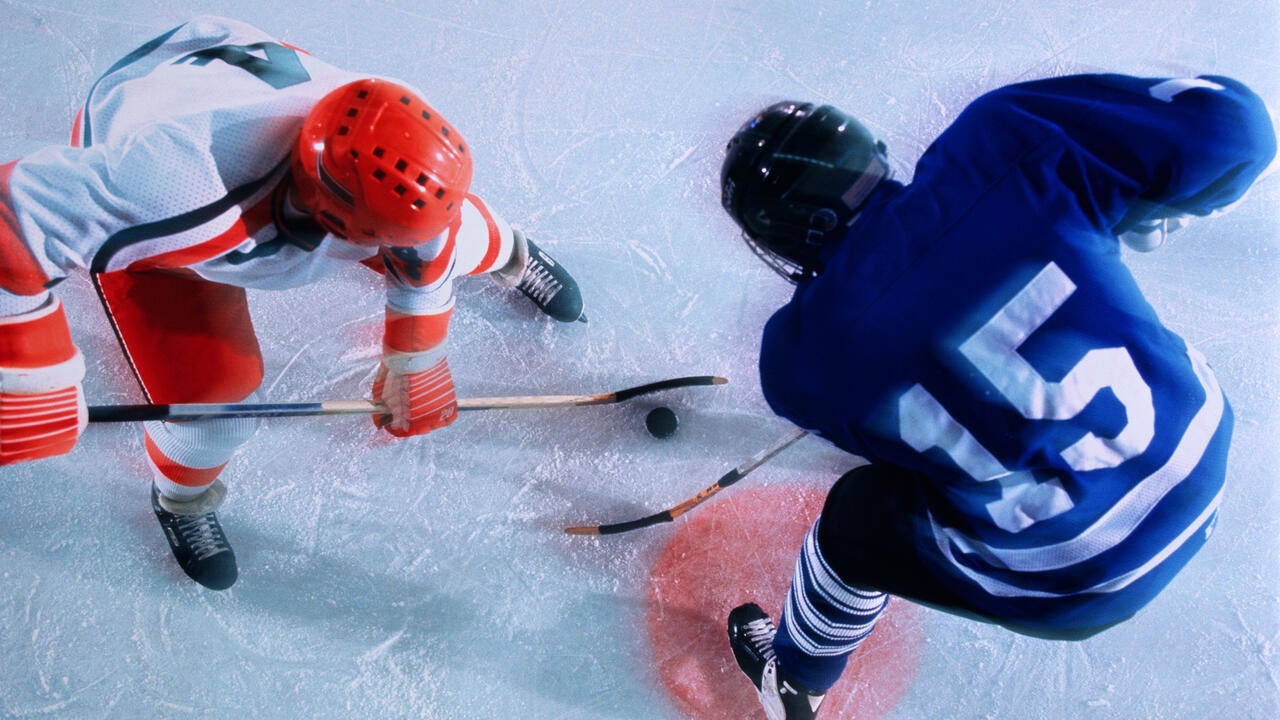
Artificial intelligence makes it faster, easier to analyze hockey video
Deep-learning AI techniques can identify players by their sweater numbers with 90-per-cent accuracy
Deep-learning AI techniques can identify players by their sweater numbers with 90-per-cent accuracy
By Media RelationsResearchers have made a key advancement in the development of technology to automatically analyze video of hockey games using artificial intelligence.
Engineers at the University of Waterloo combined two existing deep-learning AI techniques to identify players by their sweater numbers with 90-per-cent accuracy.
“That is significant because the only major cue you have to identify a particular player in a hockey video is jersey number,” said Kanav Vats, a PhD student in systems design engineering who led the project. “Players on a team otherwise appear very similar because of their helmets and uniforms.”
Player identification is one aspect of a complicated challenge as members of the Vision and Image Processing (VIP) Lab at Waterloo work with industry partner Stathletes Inc. on AI software to analyze player performance and produce other data-driven insights.
The researchers built a data set of more than 54,000 images from National Hockey League games – the largest data set of its kind – and used it to train AI algorithms to recognize sweater numbers in new images.
Accuracy was boosted by representing the number 12, for instance, as both a two-digit number and two single digits, 1 and 2, put together, an approach known in the field of AI as multi-task learning.
“Using different representations to teach the same thing can improve performance,” Vats said. “We combined a wholistic representation and a digit-wise representation with great results.”
The research team is also developing AI to track players in video, locate them on the ice and recognize what they are doing, such as taking a shot or checking an opposing player, for integration in a single system.
Detailed analytics have made great strides in hockey and other sports in recent years, but much of the work is still done by people watching broadcast video and taking notes.
“As you can imagine, a person manually annotating video of a full hockey game of three periods would take hours,” Vats said. “Machine-learning systems can produce data from videos in a matter of minutes.”
While they have focused so far on hockey, the researchers expect their technology could be transferred with modifications to other team sports, such as soccer.
Vats collaborated on the player identification work with his doctoral supervisors, Waterloo engineering professors David Clausi and John Zelek, and postdoctoral fellow Mehrnaz Fani.
He is scheduled to present a paper, Multi-task learning for jersey number recognition in Ice Hockey, at the 4th International ACM Workshop on Multimedia Analysis in Sports this month.
Read more
12 Waterloo students and postdoctoral fellows receive up to $10,000 in funding to develop their green-tech solutions
Read more
Equipment allows researchers to see inside tissues and medical devices
Read more
Waterloo Engineering researchers create an AI-powered digital imaging system to speed up biopsy results
The University of Waterloo acknowledges that much of our work takes place on the traditional territory of the Neutral, Anishinaabeg and Haudenosaunee peoples. Our main campus is situated on the Haldimand Tract, the land granted to the Six Nations that includes six miles on each side of the Grand River. Our active work toward reconciliation takes place across our campuses through research, learning, teaching, and community building, and is co-ordinated within the Office of Indigenous Relations.