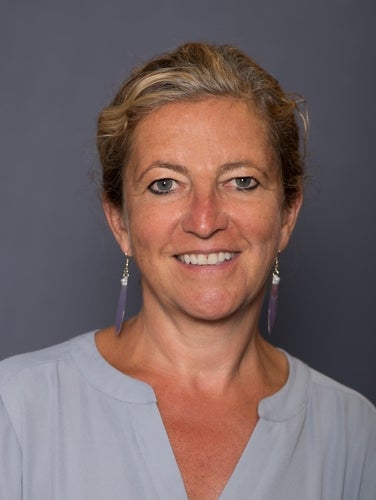
Title: Stochastic Oracles and Where to Find Them
Speaker: | Katya Scheinberg |
Affiliation: | Cornell University |
Location: | MC 5501 |
Abstract: Continuous optimization is a mature field, which has recently undergone major expansion and change. One of the key new directions is the development of methods that do not require exact information about the objective function. Nevertheless, the majority of these methods, from stochastic gradient descent to "zero-th order" methods use some kind of approximate first order information. We will overview different methods of obtaining this information, including simple stochastic gradient via sampling, robust gradient estimation in adversarial settings, traditional and randomized finite difference methods and more.
We will discuss what key properties of these inexact, stochastic first order oracles are useful for convergence analysis of optimization methods that use them.