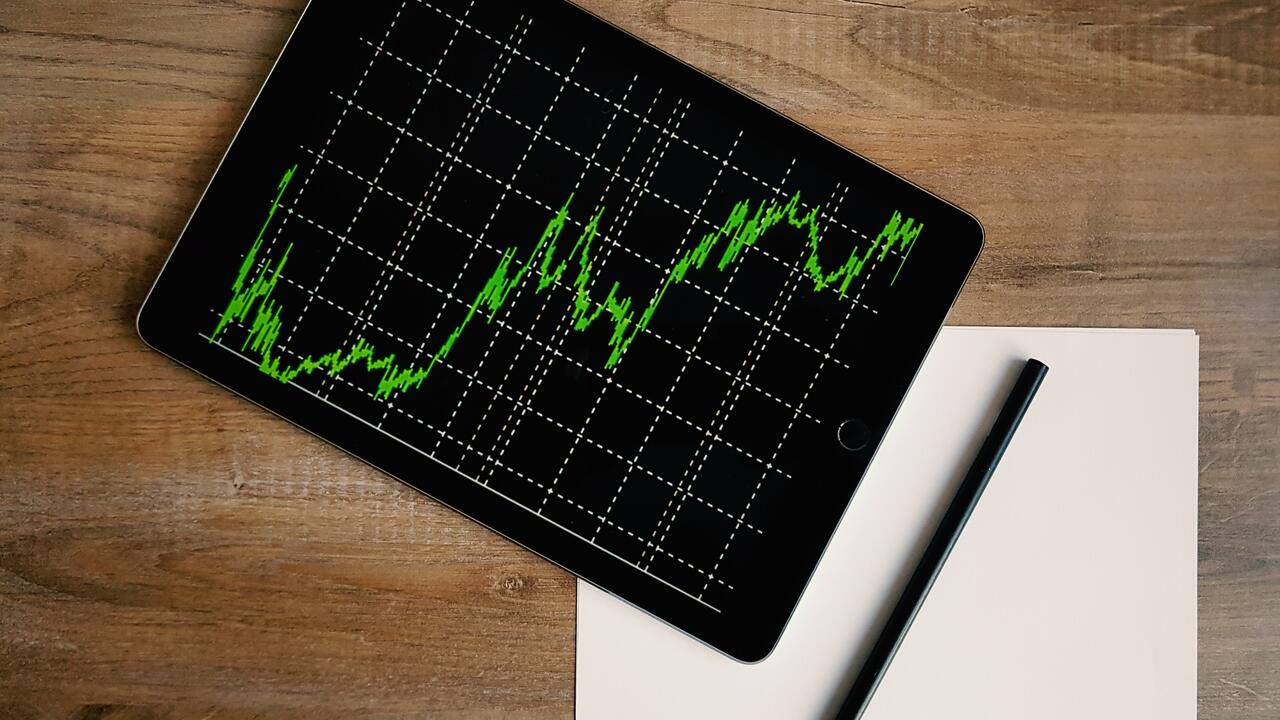
Unleashing the power of AI in the world of finance
PhD candidate Devinder Kumar led research to develop software capable of explaining the decisions made by deep-learning AI systems
PhD candidate Devinder Kumar led research to develop software capable of explaining the decisions made by deep-learning AI systems
By Brian Caldwell Faculty of EngineeringThe use of powerful artificial intelligence (AI) systems in the financial world is a step closer thanks to research at Waterloo Engineering to explain how they work.
Deep-learning AI software has the potential to generate stock market predictions, assess applicants for mortgages, set insurance premiums and perform other key financial functions.
So far, however, widespread adoption of the technology has been thwarted by a fundamental problem: understanding how and why complex AI algorithms make their decisions.
That information is crucial in financial fields to both satisfy regulatory authorities and give users confidence in those AI systems.
“If you’re investing millions of dollars, you can’t just blindly trust a machine when it says a stock will go up or down,” says Devinder Kumar, the lead researcher and a PhD candidate in systems design engineering at Waterloo.
The explainability problem, as it is called, stems from the fact that deep-learning AI algorithms essentially teach themselves by processing and detecting patterns in vast amounts of data. As a result, even their creators don’t know exactly how they make their decisions.
Kumar and his collaborators – engineering professors Alexander Wong of Waterloo and Graham Taylor of the University of Guelph – set out to solve that problem by first developing an algorithm to predict next-day movements on the S & P 500 stock index.
That system was trained with three years of historical data and programmed to make predictions based on market information – including high, low, open and close levels for the index, plus trading volume – from the previous 30 days.
The researchers then developed software called CLEAR-Trade to highlight, in colour-coded graphs and charts, the days and daily factors most relied on by the predictive AI system for each of its decisions.
A first for deep-learning AI systems in finance, those insights would allow analysts to use their experience and knowledge of world events, for example, to determine if the decisions make sense or not.
And although the stock market was used for research purposes, the explanatory software developed at Waterloo is potentially applicable to predictive deep-learning AI systems in all areas of finance.
“Our motivation was to create an explainability system rather than a very good predictive system,” says Kumar, a member of the Vision and Image Processing (VIP) Lab at Waterloo. “Whatever system you have, we can explain its decision-making processes and insights.”
The ability to explain deep-learning AI decisions is expected to become increasingly important as technology improves and authorities require financial institutions to provide reasons to the people affected by them. That could include rejected mortgage applicants, for example.
“Banks need an explainable model,” Kumar says. “They can’t just use a black box in that kind of situation. Regulators want them to be able tell their clients why they’re being denied service.”
Field trials of the software are expected to start within a year and hopes are running high for its commercial potential.
“This will allow institutions to use state-of-the-art AI systems for financial decisions,” Kumar says. “The potential impact, especially in regulatory settings, is massive.”
Photo by Burak Kebapci from Pexels
For more information – Journal of Computational Vision and Imaging Systems
Read more
A social robot could help children and families stick to a treatment for 'lazy eye'
Read more
Two researchers share their expertise for building better cities for humanity
Read more
The University of Waterloo inspires unconventional and boundary-breaking research to develop future-focused solutions for local and global challenges
Read
Engineering stories
Visit
Waterloo Engineering home
Contact
Waterloo Engineering
The University of Waterloo acknowledges that much of our work takes place on the traditional territory of the Neutral, Anishinaabeg, and Haudenosaunee peoples. Our main campus is situated on the Haldimand Tract, the land granted to the Six Nations that includes six miles on each side of the Grand River. Our active work toward reconciliation takes place across our campuses through research, learning, teaching, and community building, and is co-ordinated within the Office of Indigenous Relations.