
The University of Waterloo Cybersecurity and Privacy Institute was proud to host its Annual Conference on Thursday October 10, from 8:30 AM to 5:30 PM, at Federation Hall on the University of Waterloo Campus.
This event centred on our theme, “TACKLING CANADA’S CYBERSECURITY CHALLENGES", which highlighted current and future efforts within the cybersecurity and privacy sphere, with keynote speakers, panel discussions, and industry talks. This conference was open to undergraduate and graduate students, faculty, entrepreneurs, start ups, government, sponsors, and businesses.
Our intent was to facilitate conversation between CPI experts and experts in prominent application domains that have critical cybersecurity/privacy challenges including:
- Open banking
- Elections security
- Quantum technologies
- Societal surveillance
The following students presented their posters at the Annual Conference:
*NOTE: Numbering is purely sequential for ease of identification and has no other value.
Congratulations to the following students for their respective wins:
First Place worth $1,000: Abdulrahman Diaa
Second Place worth $500: Sina Kamali
Third Place worth $300: Yuzhe You and Jarvis Tse
This year's first place prize money was generously donated by Rogers with second and third place being funded by CPI.
1: Abdulrahman Diaa Optimizing Adaptive Attacks Against Content Watermarks for Language Models Supervisor: Florian Kerschbaum CS
2: Amandeep Kaur Moving Beyond LDA: A Comparison of Unsupervised Topic Modelling Techniques for Qualitative Data Analysis of Online Communities Supervisor: James R. Wallace CS
3: Aosen Xiong and Alex Cook Pluggable Properties for Program Correctness Supervisor: Werner Dietl ECE
4: Cameron Hadfield Generative Fuzz Grammars: Black-Box Grammar Generation Using Off-the-Shelf LLMs Supervisor: Sebastian Fischmeister ENG
5: Danielle Thompson Digital Workplace Surveillance in Ontario: Assessing the Impacts and Regulation of Employee Monitoring in the Remote Work Era Supervisor: Adam Molnar ARTS
6: Federico Mazzone PP-Klusty: Privacy-Preserving K-Means Clustering for Vertically Partitioned Data Using Homomorphic Encryption Supervisor: Florian Kerschbaum CS
7: JaeEun Shin Toward Safe Interaction with Social Robots: Children and Parents' Perception of Privacy in Humanoid Social Robots Supervisor: Leah Zhang-Kennedy & Yue Hu SIDB & ENG
8: Joseph Tafese eBPF Verification Supervisor: Arie Gurfinkel ECE
9: Julie Kate Seirlis Cybersecurity, Vulnerable Populations and the Global South United College
10: Martin Lochner Shivam Baikerikar Detecting Intrusion Stemming From Microsoft Copilot
11: Mehdi Aghakishiyev Detecting and transforming side-channel vulnerable code Supervisor: N. Asokan and Meng Xu CS
12: Michael Wrana TSA-WF: Enhancing Multi-Tab Website Fingerprinting Attacks with Time Series Analysis Supervisor: Diogo Barradas and N Asokan CS
13: Sina Kamali Nika: Anonymous Blackout-Resistant Microblogging with Message Endorsing Supervisor: Diogo Barradas CS
14: Vasisht Duddu SoK: Unintended Interactions among Machine Learning Defenses and Risks Supervisor: N. Asokan CS
15: Yuzhe You AdvEx: Understanding Adversarial Attacks with Interactive Visualizations Supervisor: Jian Zhao CS
Abstracts & PDF's
1: Abdulrahman Diaa Optimizing Adaptive Attacks Against Content Watermarks for Language Models Supervisor: Florian Kerschbaum CS
Large Language Models (LLMs) can be \emph{misused} to spread online spam and misinformation. Content watermarking deters misuse by hiding a message in model-generated outputs, enabling their detection using a secret watermarking key. Robustness is a core security property, stating that evading detection requires (significant) degradation of the content's quality. Many LLM watermarking methods have been proposed, but robustness is tested only against \emph{non-adaptive} attackers who lack knowledge of the watermarking method and can find only suboptimal attacks. We formulate the robustness of LLM watermarking as an objective function and propose preference-based optimization to tune \emph{adaptive} attacks against the specific watermarking method. Our evaluation shows that (i) adaptive attacks substantially outperform non-adaptive baselines. (ii) Even in a non-adaptive setting, adaptive attacks optimized against a few known watermarks remain highly effective when tested against other unseen watermarks, and (iii) optimization-based attacks are practical and require less than seven GPU hours. Our findings underscore the need to test robustness against adaptive attackers.

2: Amandeep Kaur Moving Beyond LDA: A Comparison of Unsupervised Topic Modelling Techniques for Qualitative Data Analysis of Online Communities Supervisor: James R. Wallace CS
Social media constitutes a rich and influential source of information for qualitative researchers. Although computational techniques like topic modelling assist with managing the volume and diversity of social media content, qualitative researcher's lack of programming expertise creates a significant barrier to their adoption. In this paper we explore how BERTopic, an advanced Large Language Model (LLM)-based topic modelling technique, can support qualitative data analysis of social media. We conducted interviews and hands-on evaluations in which qualitative researchers compared topics from three modelling techniques: LDA, NMF, and BERTopic. BERTopic was favoured by 8 of 12 participants for its ability to provide detailed, coherent clusters for deeper understanding and actionable insights. Participants also prioritised topic relevance, logical organisation, and the capacity to reveal unexpected relationships within the data. Our findings underscore the potential of LLM-based techniques for supporting qualitative analysis.

3: Aosen Xiong and Alex Cook Pluggable Properties for Program Correctness Supervisor: Werner Dietl ECE
Optional type systems are powerful tools to specify and enforce desired properties at compile time, thus preventing run time errors. In this poster, we present the unified framework EISOP (Enforcing, Inferring, and Synthesizing Optional Properties) Checker Framework (CF), and Checker Framework Inference (CFI) for developing optional type systems and constraint-based type inference tools for Java. The checkers produced by the CF can be used with the Java compiler seamlessly while inference tools based on CFI work as a stand alone engine for inserting annotations in source code. This is used in conjunction with solving constraints by SMT solvers in order to reduce annotation effort. Based on CF and CFI, the following have been developed: JSpecify Nullness Checker, Practical Class and Object Immutability Checker and Units of measurement Checker which prevent Null Pointer dereferences, unwanted side effects and dimensional inconsistency, respectively. We show the design decisions of each type system and the guarantees each provides. In addition, we present examples of our type systems correctly detecting errors and case study results for our type checker and inference tool.
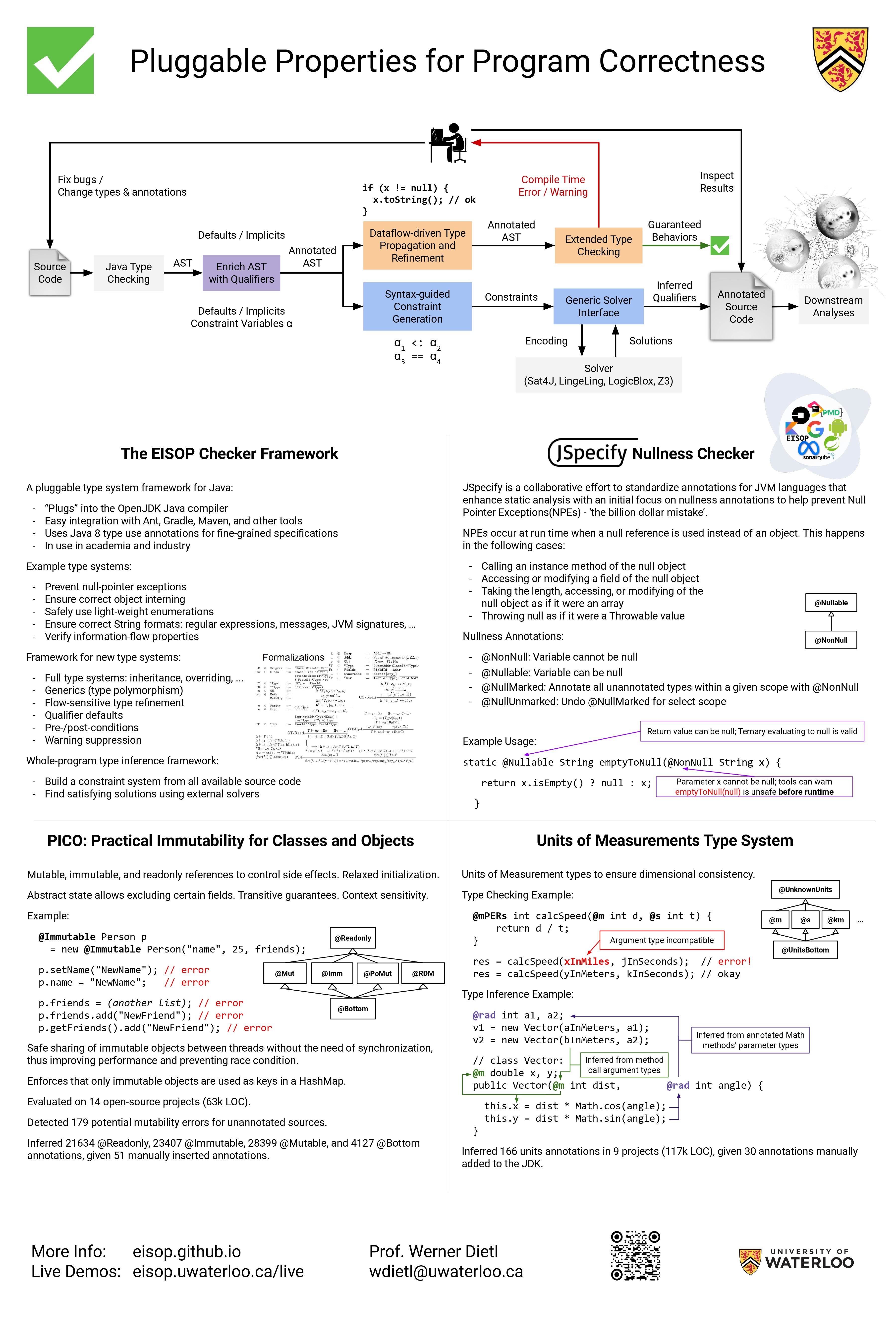
4: Cameron Hadfield Generative Fuzz Grammars: Black-Box Grammar Generation Using Off-the-Shelf LLMs Supervisor: Sebastian Fischmeister ENG
Black-box hardware fuzzing has two general problems: detecting system responses and grammar generation. This work addresses the latter problem of grammar generation. Typically, grammar generation combines all accessible information about the target to create a decisive format for the system's accepted commands and the semantic understanding of what example commands may look like. Such analysis is time-consuming and may not even touch on the space of commands that do not rigidly conform to this format. Our work improves this method, using off-the-shelf large language models (LLMs) to supplement command generation. Using our process, we demonstrate that such models can extrapolate additional accepted commands using only a text-based target description and a short capture of the observed communications. In doing so, we automate the fuzz testing process, allowing for the quicker development of testing tools.
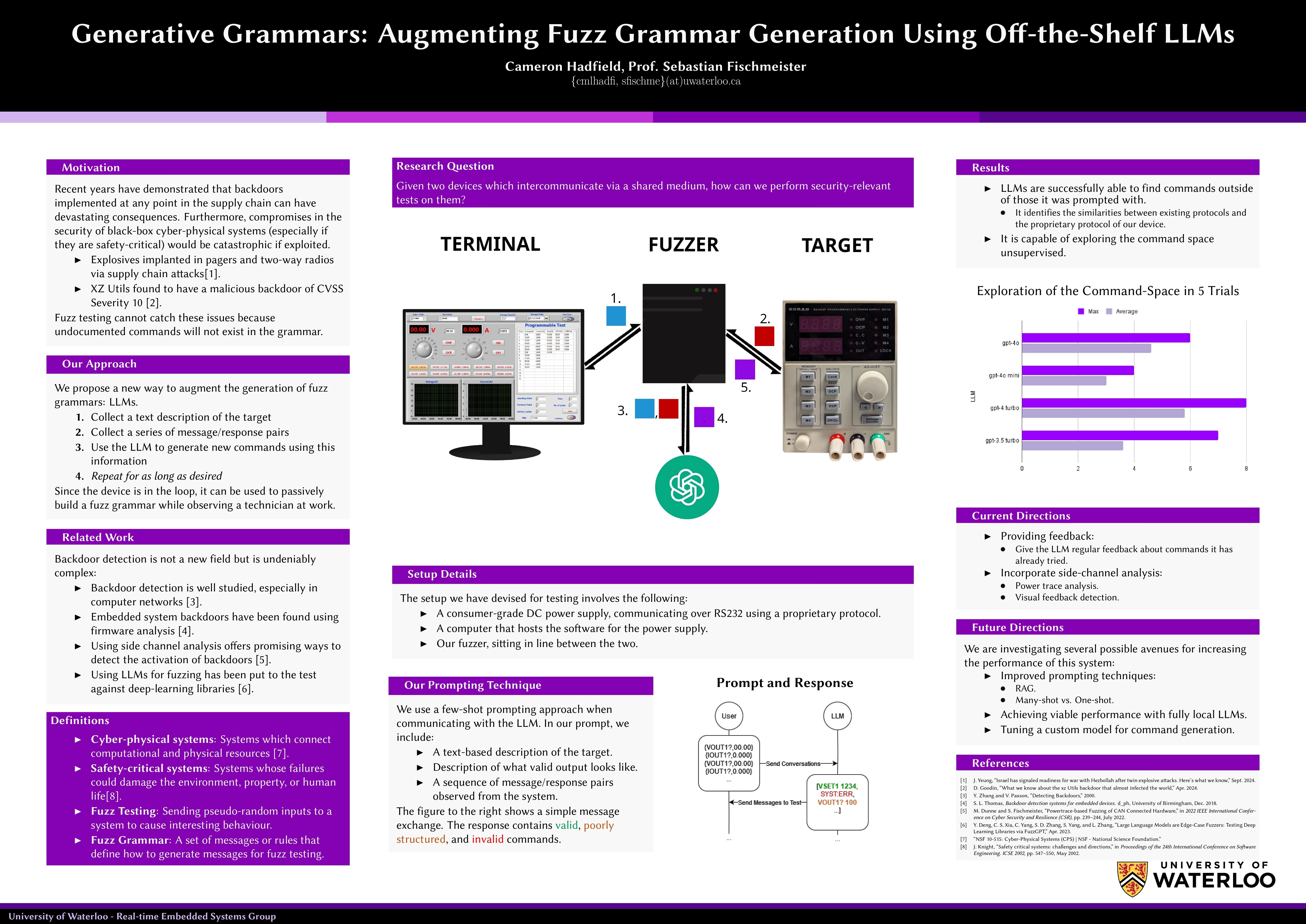
5: Danielle Thompson Digital Workplace Surveillance in Ontario: Assessing the Impacts and Regulation of Employee Monitoring in the Remote Work Era Supervisor: Adam Molnar ARTS
My PhD research examines the use, operation, and impacts of employee monitoring applications (EMAs) in Ontario. EMAs are a type of digital surveillance software that provide employers with the ability to monitor the behaviours of their workers through features like keystroke logging, screen recording, video surveillance, and communications monitoring. The use of these applications has greatly increased since the COVID-19 pandemic and raises serious concerns about the potential impacts of these technologies on employee privacy, wellness, and employee-employer trust relations, particularly when used in traditionally private spaces like the home (i.e., remote work). The urgency of these concerns, specifically that of privacy, has been acknowledged by the Ontario government in their passing of Bill 88, the “Working for Workers Act, 2022,” which requires employers with more than 25 employees to have a written policy stating whether and how they use EMAs (Mathews Dinsdale, 2022; Kosseim, 2022). Yet, the current literature on EMAs does not address how Canadian workplaces use these apps, how these apps are experienced by various workplace actors, and whether and/or how policy—such as privacy and other regulations—impact workplace monitoring practices and sentiment.
This research is guided by the following five objectives: (1) Examine workplace monitoring policies to understand whether, how, and for what purposes workplaces are monitoring their workers, (2) interview/survey managers/supervisors to examine the dominant framings employed about the benefits and drawbacks of EMAS, (3) interview/survey employees to examine how they experience privacy and wellness in the context of EMAs, (4) interview/survey security administrators to examine how they perceive the impact of EMAs on security, and (5) evaluate how laws and policies regulate EMA harms and whether/how regulation can be improved. This research will deepen our understanding of the uses of EMAs and the harms that they may produce. In addition to contributing to the literature on EMAs and remote work, this research will help to inform the development of legislation that protects the privacy and wellness of employees.

6: Federico Mazzone PP-Klusty: Privacy-Preserving K-Means Clustering for Vertically Partitioned Data Using Homomorphic Encryption Supervisor: Florian Kerschbaum CS
Clustering records in a dataset according to one or more features is a fundamental tool in data processing. When the data is distributed among different entities, each of which owns only a subset of the features, we encounter the so-called vertically partitioned scenario. The primary goal in this situation is to enable the involved parties to compute the joint clusters, while keeping each (partial) dataset private. Previous solutions relied on techniques such as secret sharing and garbled circuits to achieve this functionality by implementing a privacy-preserving variant of the Lloyd’s algorithm. Unfortunately, these methods often incur a very high communication cost, scaling as O(nkt), where n is the number of points, k is the number of clusters, and t is the number of iterations in Lloyd’s algorithm. In our work, we present a solution based on fully homomorphic encryption with a communication complexity of O(n + kt). Our approach introduces an innovative method for computing the argmin under encryption, which can be generalized to any order statistic and be of independent interest. Our empirical evaluations demonstrate the protocol’s practicality, achieving remarkable results with a communication requirement of only 68MB for clustering 100,000 points into five clusters, compared to 181GB for existing methods. Moreover, our protocol completes the clustering process in under 8 minutes, a substantial improvement over the 2-hour runtime of current state-of-the-art techniques, all while maintaining comparable accuracy to plaintext k-means algorithms.
PLEASE NOTE: This project will be presented in 2025, hence the poster is not currently available for public presentation.
7: JaeEun Shin Toward Safe Interaction with Social Robots: Children and Parents' Perception of Privacy in Humanoid Social Robots Supervisor: Leah Zhang-Kennedy & Yue Hu SIDB & ENG
As social robots become more prevalent, research has highlighted the educational and developmental benefits of child-robot interaction. However, it is crucial to address concerns and risks around data security and privacy when using these robots. The study aims to: 1) understand how children accept and perceive Humanoid Social Robots (HSRs) and their associated privacy risks, and 2) identify similarities and differences between children's and parents' risk perceptions in child-robot interactions. We conducted user studies with families using the educational robot, NAO, through pre-programmed activities designed to elicit privacy and security concerns. While children generally responded positively, showing trust and willingness to share private information with the robot, parents were more cautious. They expressed lower levels of acceptance than children and greater concern and about data collection, storage, and transfer, preferring that interactions be supervised by adults or school and remain under parental control. Parents' concerns stemmed from a lack of understanding of the robot's capabilities and a need for greater transparency regarding data collection, transfer, and storage. They were particularly concerned about the robot collecting information in private spaces or when children interacted with the robot independently or in others' home. The findings suggest that designing safer robot interactions in household and educational settings should prioritize family privacy concerns and address the need for great parental awareness, control, and transparency in children's interactions with HSRs.

8: Joseph Tafese eBPF Verification Supervisor: Arie Gurfinkel ECE
eBPF has been a revolutionary technology that has transformed the landscape of kernel extension. This has been enabled by tooling and support for the development of small programs that can be used to enhance a given kernel’s functionality. We present a tool that reads low level eBPF code and generates a custom representation with verification in mind. Our analysis is able to provide memory safety guarantees using mathematical logic, or a test case that shows a critical violation of memory safety.
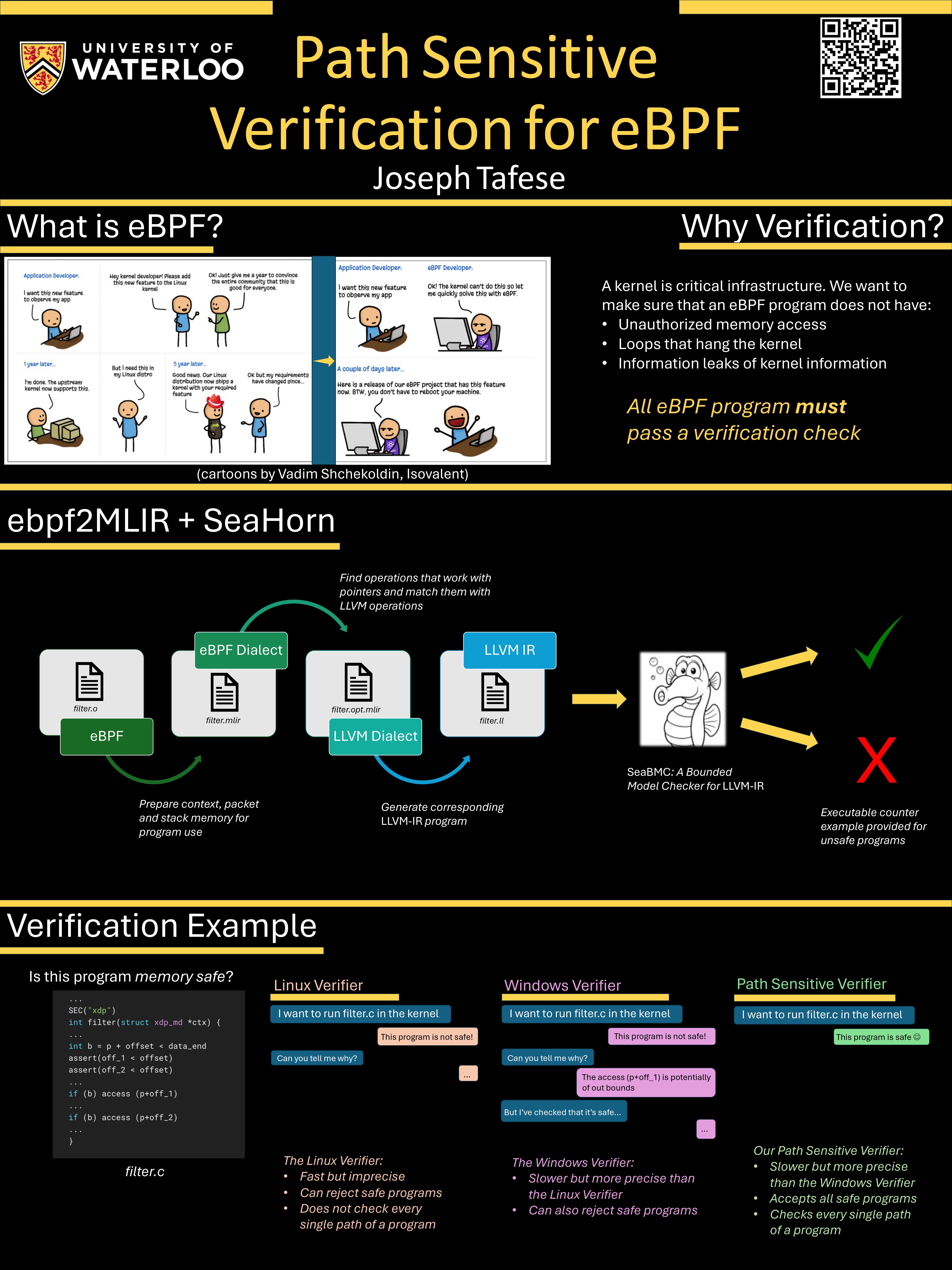
9: Julie Kate Seirlis Cybersecurity, Vulnerable Populations and the Global South United College
Our organisation designs education programs on cybersecurity for and in the Global South with a particular focus on vulnerable populations such as the elderly and young adolescents. Our study will explore the effectiveness of these programs using both quantitative surveys and qualitative interviews to ascertain and assess two issues: cybersecurity knowledge and subsequent on-line behaviour. Built in to our design and our study are sensitivity to the historical and current political, social, cultural and economic context in general and, more specifically, the role these play in shaping the technological and cybersecurity landscape. Our premise is that cyber education, when carefully planned and delivered, can both protect marginalised peoples and foster them as full digital citizens. Our initial field sites include Haiti and the anglophone and francophone Eastern Caribbean islands.
10: Martin Lochner Shivam Baikerikar Detecting Intrusion Stemming From Microsoft Copilot
In today’s rapidly evolving digital landscape, organizations are increasingly adopting AI-powered productivity tools, including Generative AI platforms like Microsoft Copilot. While these tools significantly enhance productivity, they also introduce potential security risks. The use of GenAI applications may unintentionally expose confidential data to the platform, resulting in potential leaks of sensitive information and intellectual property. To address these challenges, it is crucial to understand how employees are using GenAI tools like Microsoft Copilot and to maintain full visibility into their interactions. This project aims to bridge these gaps by utilizing existing data sources, such as log services, to provide comprehensive insights into user activities and behaviors. Our approach involves collecting interactions between LLMs and users through customer gateways, such as proxies and firewalls, where logs are generated and continuously monitored. By extracting relevant telemetry data, we can develop detection queries that analyze this information to generate alerts. The captured interactions are then presented as a dashboard in the Insights portal, providing visibility into user behavior and potential risks. These analyses enable us to identify key users, understand user behavior, and enhance the overall user experience while ensuring secure access to Microsoft Copilot.

11: Mehdi Aghakishiyev Detecting and transforming side-channel vulnerable code Supervisor: N. Asokan and Meng Xu CS
The BliMe architecture enhances secure outsourced computation by employing hardware-enforced taint tracking to protect sensitive client data from leaking into observable states. However, if the existing code is not BliMe compliant, the architecture will cause it to crash, limiting the usability of the BliMe architecture. This work presents a static analysis tool for identifying code segments that would violate BliMe’s taint-tracking policies, and develop code transformations for making the code BliMe-compliant. Static analysis aims to find code segments that can potentially cause BliMe violations, such as the usage of tainted data in control flow instructions or memory access. In our initial testing, we obtain similar results when running both the analysis and BliMe on relatively simple programs in the OISA benchmark. Currently, we are working on increasing the capability of the analysis to run on larger benchmarks. After obtaining analysis results, the goal is to implement code transformations using LLVM infrastructure to modify the source code to be BliMe-compliant without affecting its semantics.
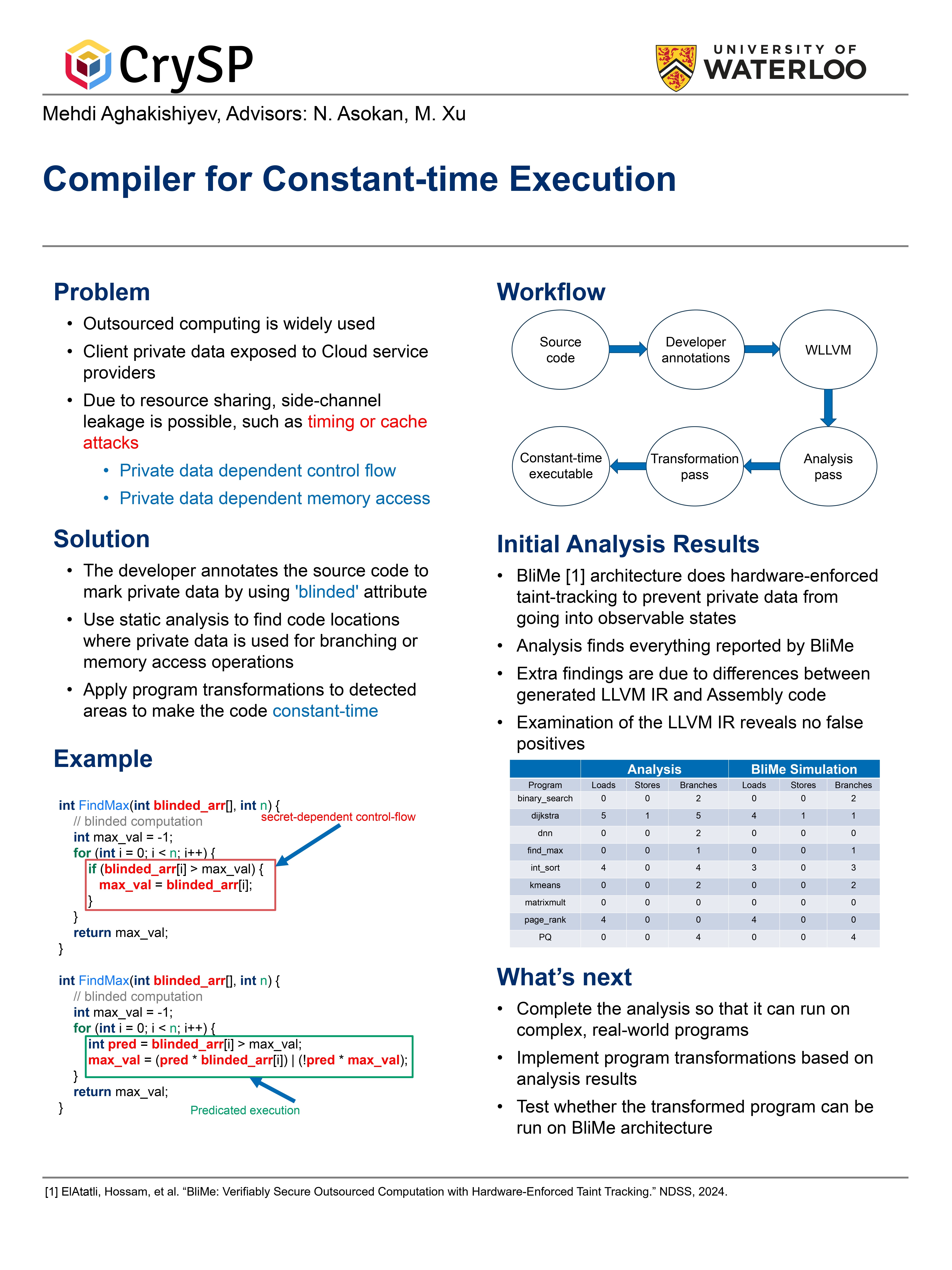
12: Michael Wrana TSA-WF: Enhancing Multi-Tab Website Fingerprinting Attacks with Time Series Analysis Supervisor: Diogo Barradas and N Asokan CS
Website fingerprinting (WF) is a technique that allows a local adversary to determine the website a target user is accessing by inspecting the metadata associated with packets exchanged via some encrypted tunnel. WF attacks typically rely on classifiers that reason over a set of features to describe this network trace metadata, but preprocessing steps performed over the original metadata often lead to information loss. Thus, predictions issued by WF classifiers often lack the inclusion of precise information about the instant when a given website is detected within a (potentially large) network trace comprised of multiple sequential website accesses (a setting known as multi-tab WF). In this paper, we present TSA-WF, a time series analysis-based pipeline for WF that operates on a data representation that more closely preserves network traces’ timing and direction characteristics to address the issues raised by feature engineering. TSA-WF outperforms existing WF attacks with an accuracy of 91.2% when classifying website accesses that can be singled-out from a given trace (i.e., the more common single-tab WF setting). Additionally, TSA-WF can pinpoint the precise instant in which a website was visited within a trace that combines multiple websites accessed in sequence (multi-tab) with over 98% accuracy.
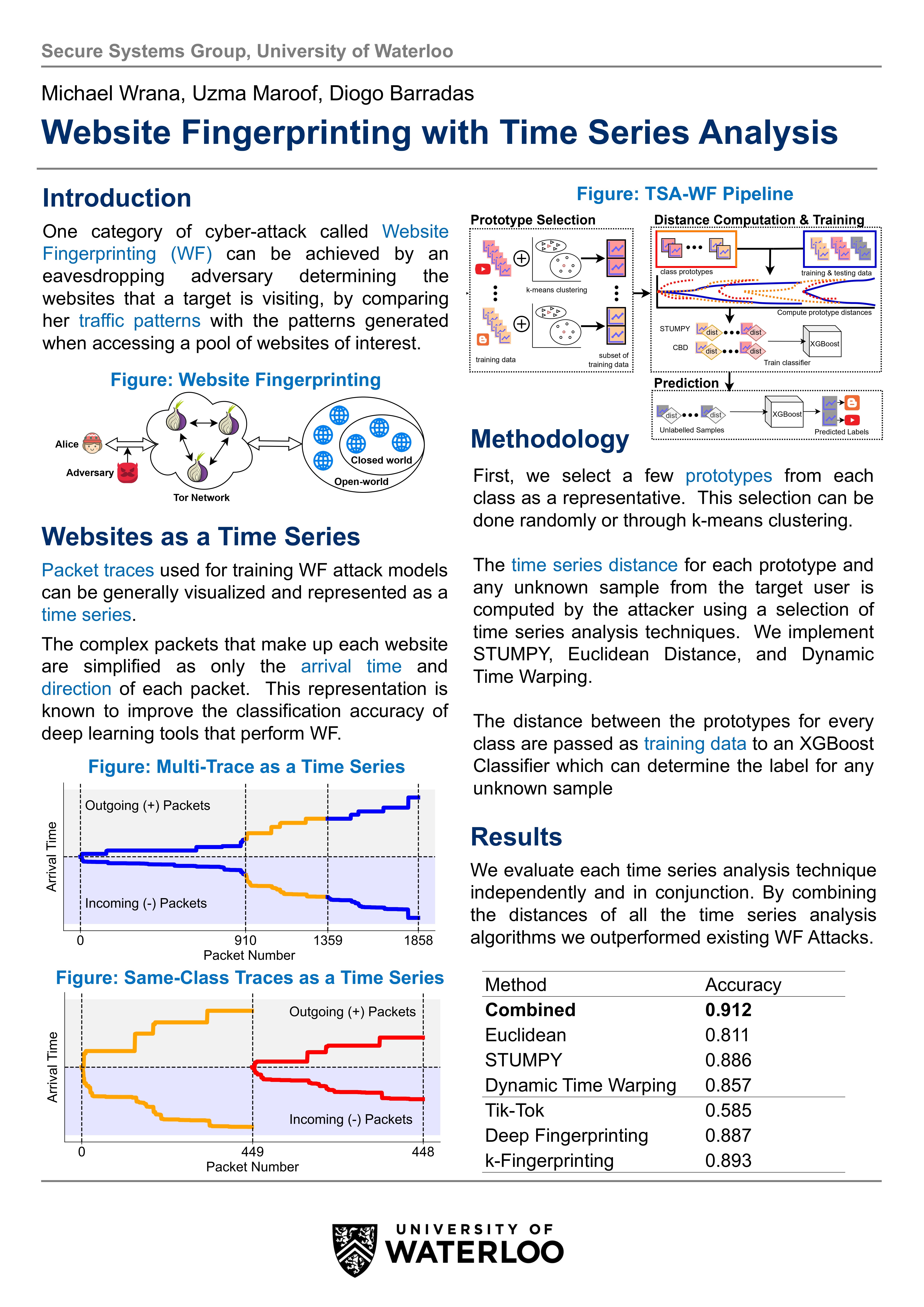
13: Sina Kamali Nika: Anonymous Blackout-Resistant Microblogging with Message Endorsing Supervisor: Diogo Barradas CS
Repressive governments are increasingly resorting to Internet shutdowns to control the flow of information during political unrest. In response, messaging apps built on top of mobile-based mesh networks have emerged as important communication tools for citizens and activists. While different flavors of these apps exist, those featuring microblogging functionalities are attractive for swiftly informing and mobilizing individuals. However, most apps fail to simultaneously uphold user anonymity while providing safe ways for users to build trust in others and the messages flowing through the mesh. We introduce Nika, a blackout-resistant app with two novel features: remote trust establishment and anonymous message endorsing. Nika also leverages a set of identity revocation primitives for the fine-grained management of trust relationships and to provide enhanced anonymity. Our evaluation of Nika through comprehensive micro-benchmarks and simulations showcases its practicality and resilience in shutdown scenarios.
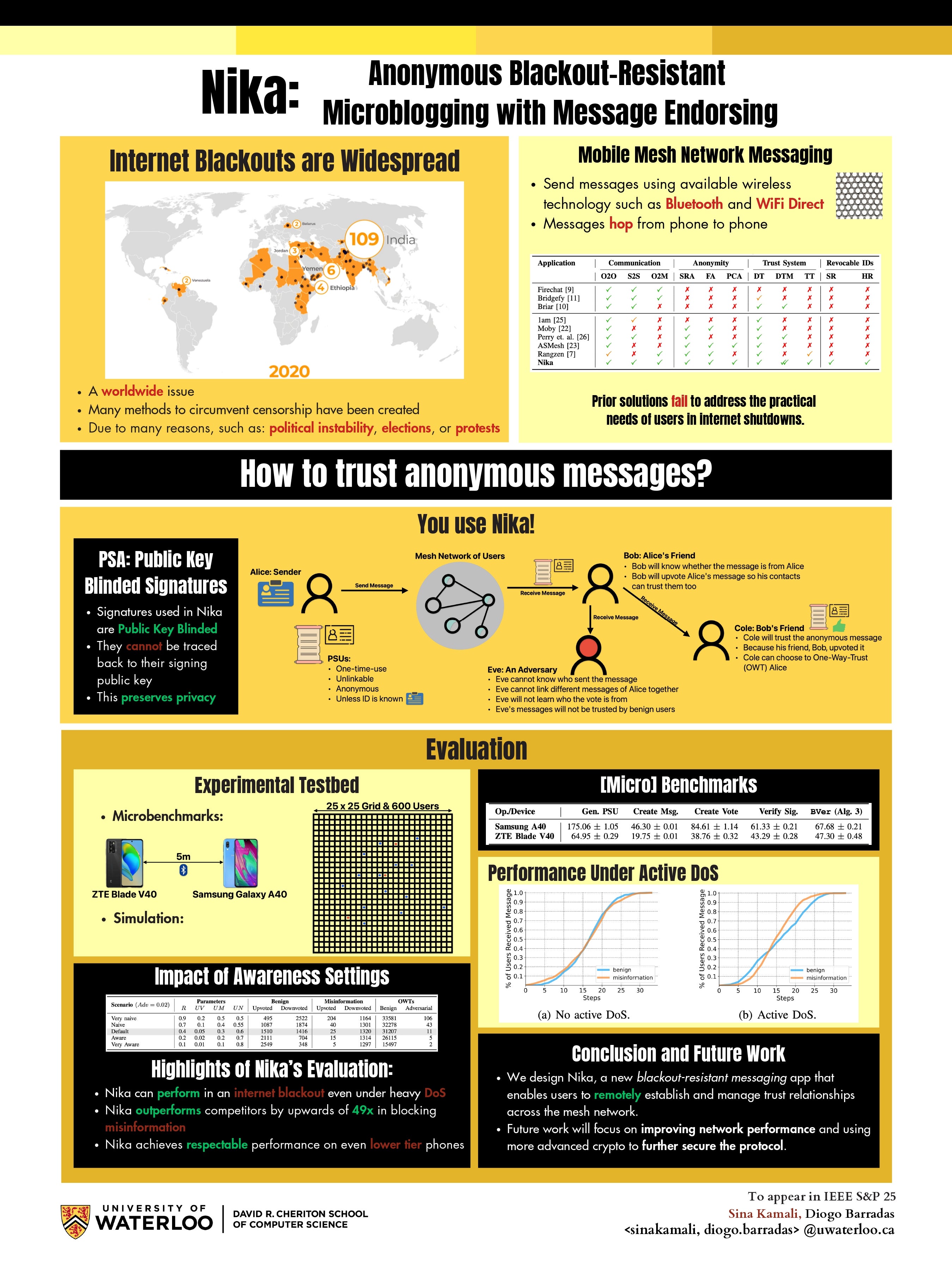
14: Vasisht Duddu SoK: Unintended Interactions among Machine Learning Defenses and Risks Supervisor: N. Asokan CS
Machine learning (ML) models cannot neglect risks to security, privacy, and fairness. Several defenses have been proposed to mitigate such risks. When a defense is effective in mitigating one risk, it may correspond to increased or decreased susceptibility to other risks. Existing research lacks an effective framework to recognize and explain these unintended interactions. We present such a framework, based on the conjecture that overfitting and memorization underlie unintended interactions. We survey existing literature on unintended interactions, accommodating them within our framework. We use our framework to conjecture on two previously unexplored interactions, and empirically validate our conjectures.
Link: https://arxiv.org/abs/2312.04542

15: Yuzhe You AdvEx: Understanding Adversarial Attacks with Interactive Visualizations Supervisor: Jian Zhao CS
Adversarial machine learning (AML) focuses on studying attacks that can fool machine learning algorithms into generating incorrect outcomes as well as the defenses against worst-case attacks to strengthen the adversarial robustness of machine learning models. Specifically for image classification tasks, it is difficult to comprehend the underlying logic behind adversarial attacks due to two key challenges: 1) the attacks exploiting “non-robust” features that are not human-interpretable and 2) the perturbations applied being almost imperceptible to human eyes. We propose an interactive visualization system, AdvEx, that presents the properties and consequences of evasion attacks as well as provides data and model performance analytics on both instance and population levels. We quantitatively and qualitatively assessed AdvEx in a two-part evaluation including user studies and expert interviews. Our results show that AdvEx is effective both as an educational tool for understanding AML mechanisms and a visual analytics tool for inspecting machine learning models, which can benefit both AML learners and experienced practitioners.
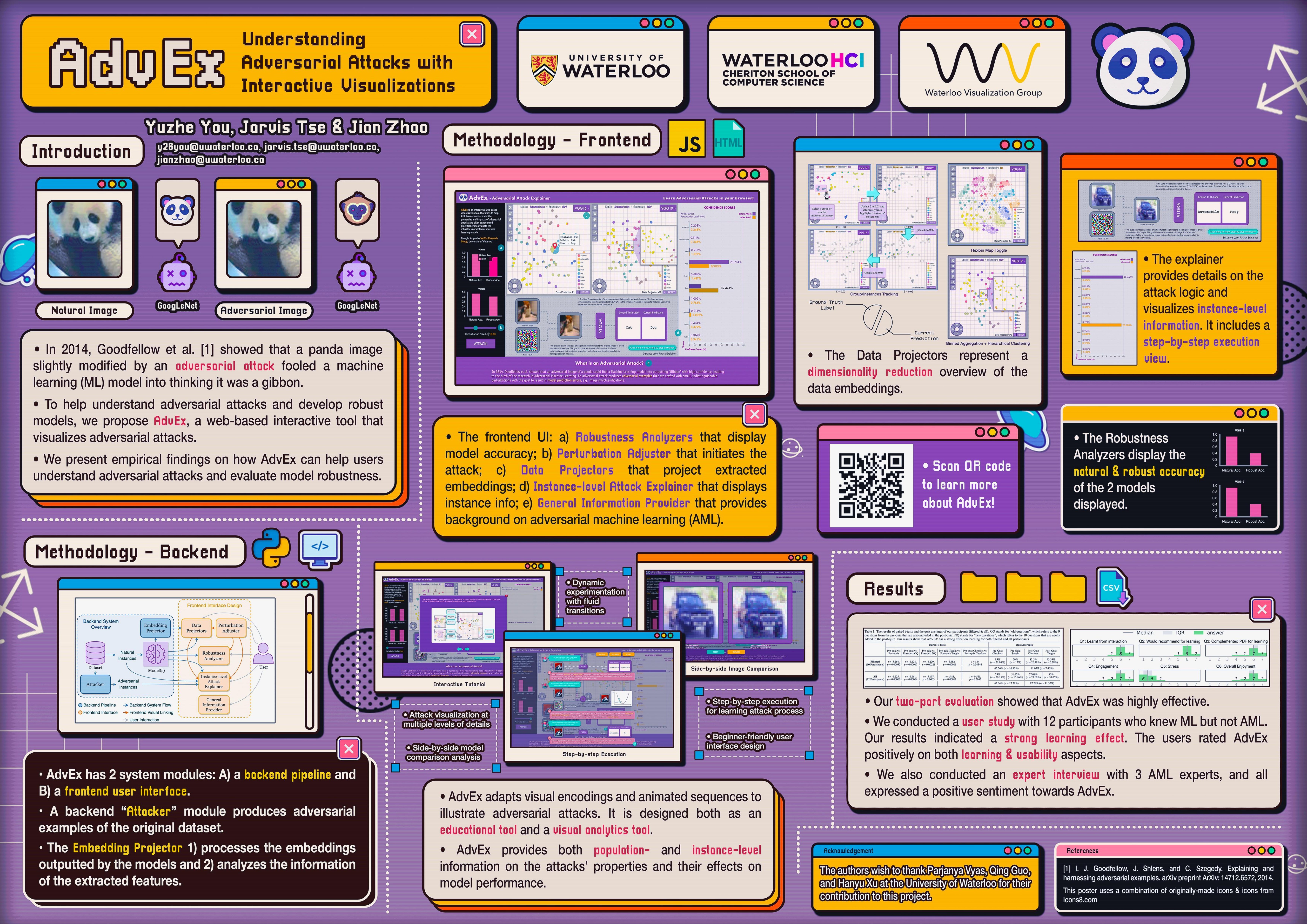