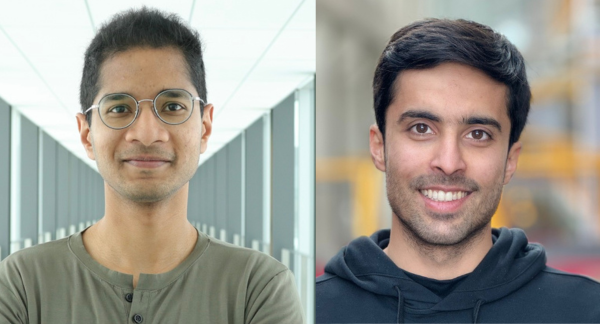
Congratulations to Vasisht Duddu and Rasoul Akhavan Mahdavi, the 2024 winners of the Cybersecurity and Privacy Excellence Graduate Scholarships. Generously sponsored by Mastercard and BTQ, each of these graduate students will receive $10,000 for attaining this prestigious award. We had many high calibre applicants for this year’s scholarships, the competition was the highest level it has been since the inception of this award in 2019.
Vasisht Duddu expressed the following upon receiving this award:
“My research examines how different machine learning defenses interact with various risks, emphasizing the need to consider unintended risks beyond those the defense was designed to address. I also work ensuring accountability in machine learning pipelines for regulatory compliance to establish trust in machine learning systems.
I am grateful to the Cybersecurity and Privacy Institute for recognizing my work and reinforcing my belief in its relevance to both academia and industry. This award will provide financial support as I work to design machine learning systems that positively impact society while minimizing associated harms.”
Rasoul Akhavan Mahdavi had this to add:
"This scholarship demonstrates how my work is valued by the research community and industry. It motivates me to further pursue my research and provides financial support necessary to stay focused on this path.
In my research, I focus on efficient implementations of privacy-preserving technologies for real-world applications using tools such as fully homomorphic encryption and secure multi-party computation. Privacy-preserving technologies have addressed the dilemma of privacy and usability, by allowing users to access services without disclosing private information. For example, accessing a database without revealing the query or training and inferring machine learning models without disclosing private inputs. However, the overhead of privacy-preserving solutions is high for many applications, which is a barrier to adopting these technologies in real-world applications. In my work, I focus on efficient constructions with small overhead, both in network costs and in computation, which bridge the gap between theory and practice. This is achieved by designing new algorithms for applications and enhancing the necessary cryptographic primitives such as fully homomorphic encryption. By lowering the overhead of privacy-preserving technologies, we can lower the barrier to entry and unlock a new set of private applications for users."
For further information on past winners and our scholarship program, please click here.
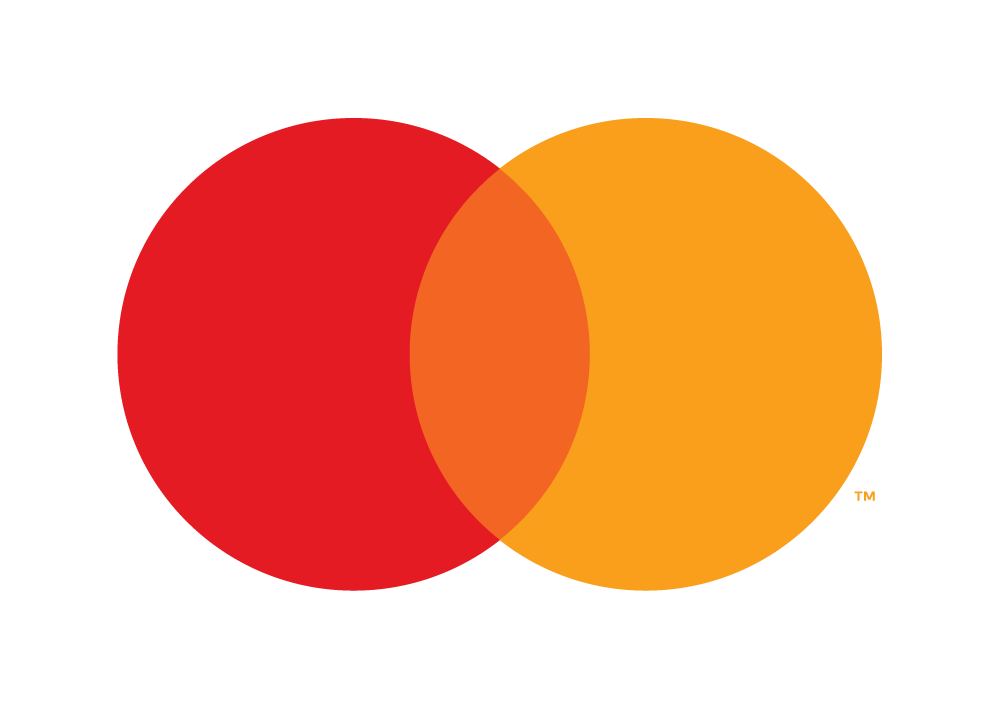