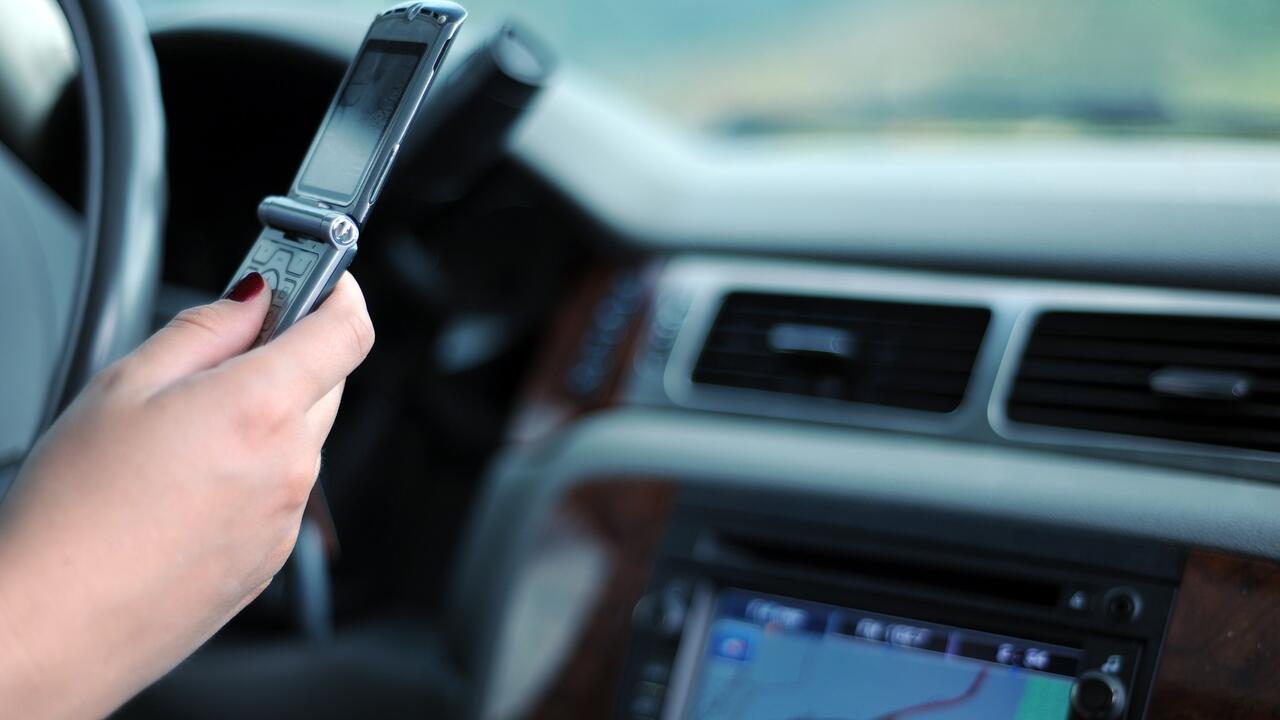
Using artificial intelligence to spot texting behind the wheel
Researchers at the University of Waterloo are developing technology to identify and classify several kinds of distracted driving
Researchers at the University of Waterloo are developing technology to identify and classify several kinds of distracted driving
By Brian Caldwell Faculty of EngineeringAs more and more advanced features are added to the highly automated vehicles of the near future, it won’t be good enough for their computer brains to know what is going on in the world around them.
They will also have to be aware of what is happening inside their own passenger compartments, especially whether their human drivers are paying attention or not.
How to determine that, a key to improving safety and satisfying regulatory authorities, is at the core of ongoing work at Waterloo Engineering involving the use of artificial intelligence (AI).
Researchers are developing technology to identify and classify several kinds of distracted driving, a problem that is to blame for up to 75 per cent of all traffic crashes worldwide.
“It has a huge impact on society,” says Fakhri Karray, director of the Centre for Pattern Analysis and Machine Intelligence (CPAMI) at Waterloo.
One system uses cameras and deep-learning-based AI algorithms to detect hand and arm movements that indicate the driver is engaged in distracting physical activities such as texting, talking on a cellphone, eating or reaching into the backseat to get something.
Also graded in terms of severity based on factors such as duration, Karray says, that information could be used in systems to warn or alert drivers when they are dangerously distracted.
And as more sophisticated self-driving capabilities are added to cars, signs of serious driver distraction could even be employed to trigger intervention for the sake of safety.
“The car could actually take over driving if there was imminent danger, even for a short while, in order to avoid crashes,” says Karray, a University Research Chair and professor of electrical and computer engineering.
That capability would fall into what is known as Level 3 autonomous driving, which is currently being designed and implemented by major car manufacturers around the world.
AI-based computer algorithms at the heart of the system were trained using machine-learning techniques to recognize physical movements deviating from normal driving behavior.
That work built on extensive prior research at CPAMI on the recognition of signs of drowsiness – such as frequent blinking, head position and face position – indicating drivers are in danger of falling asleep at the wheel.
The next step involves work to integrate the detection, processing and grading of several different kinds of distraction within a single system. Included would be cues from the face, head, eyes and hands, plus all of those cues in various combinations.
“We’re putting all the pieces together to make a fully integrated system,” Karray says. “The ultimate goal is designing a cognitive car that is self-aware of its driver, a car that knows when its driver is not doing very well and can make a critical decision to prevent a crash.”
Another research project at CPAMI involves the use of sensors to monitor physiological signals such as eye-blinking rate, pupil size and heart-rate variability as measures of driver inattention.
Read more
A social robot could help children and families stick to a treatment for 'lazy eye'
Read more
Two researchers share their expertise for building better cities for humanity
Read more
The University of Waterloo inspires unconventional and boundary-breaking research to develop future-focused solutions for local and global challenges
Read
Engineering stories
Visit
Waterloo Engineering home
Contact
Waterloo Engineering
The University of Waterloo acknowledges that much of our work takes place on the traditional territory of the Neutral, Anishinaabeg, and Haudenosaunee peoples. Our main campus is situated on the Haldimand Tract, the land granted to the Six Nations that includes six miles on each side of the Grand River. Our active work toward reconciliation takes place across our campuses through research, learning, teaching, and community building, and is co-ordinated within the Office of Indigenous Relations.