Materials informatics tools for supervised and unsupervised machine learning methods to predict crystal structures
Anton Oliynyk
Assistant Professor, Department of Chemistry
Hunter College, City University of New York
Tuesday, September 24, 2024
11 a.m.
In-person: C2-361
Abstract: Machine learning successfully predicts crystal structures with supervised methods, where training data has structure type specified. However, unsupervised learning is challenging, given that crystal structures are related to each other and cannot be easily separated based on chemical parameters calculated from their compositions. We developed software to extract useful descriptors from CIFs in a high-throughput way to make them ready for unsupervised learning, where machine needs to find out the differences between the structures on its own and predict the number of structure clusters. Our software focuses on explainable machine learning, with which we gain chemical insights in contrast to common black-box machine-learning methods. We experimentally validate our models with synthesis of novel intermetallic phases suggested by our machine-learning models:
- To demonstrate unsupervised learning method, a novel PuNi3-type phase, TbIr3, was predicted and synthesized as experimental validation.
- To expand the functionality of materials informatics tools, we automated traditional exploratory synthesis strategies with a recommendation engine that visualizes crystal structure trends and proposes the next best element to try when considering exploratory synthesis. This approach resulted in discovery of a novel Gd–Ru–Cd phase, which is an excellent neutron absorber and a material with a negative thermal expansion.
- To test the prediction of a novel class of materials, we searched for outliers in crystallographic databases. Prediction of a similar material resulted in a series of RE–RE'–M–Ge phases, which feature two RE metals occupying different crystallographic sites, allowing for separation of rare-earth metals at atomic level.
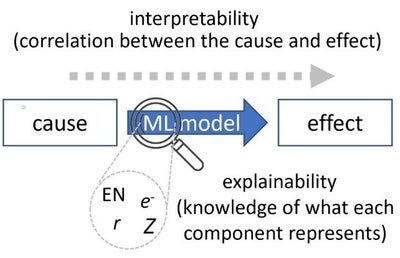
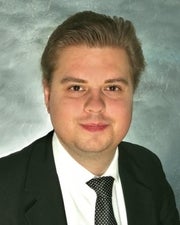
Anton Oliynyk completed his PhD at University of Alberta, postdoctoral studies at University of Houston, and continued as a research associate again at University Alberta. As an independent researcher, he was an assistant professor at a primarily undergraduate institution, Manhattan College (now Manhattan University) in New York City. Currently, appointed as an assistant professor at Hunter College, City University of New York. Anton Oliynyk has 15 years of experience in solid state chemistry synthesis and X-ray crystallography, complemented with 12 years of experience in machine learning. Combining machine learning with experimental work, he synthesized over 400 new intermetallic compounds as exploratory projects or as an experimental validation for machine-learning models. Currently, Anton Oliynyk is working in the area of solid state radiochemistry, with a focus on intermetallic materials for nuclear applications.