Probabilistic models meet computational chemistry
Rodrigo Alejandro Vargas Hernandez
Assistant Professor, Department of Chemistry & Chemical Biology
McMaster University
Friday, November 29, 2024
11 a.m.
In-person: C2-361
Abstract: Machine learning tools provides innovative approaches to longstanding challenges in computational chemistry. This talk will explore several pivotal technologies, starting with automatic differentiation, an algorithm that enhances the accuracy and efficiency of gradient calculations, crucial for molecular inverse design and swiftly tuning semi-empirical models. Additionally, I will discuss the integration of generative models, specifically normalizing flows, with advanced variational inference methods. These are employed as novel ansatzes for solving the electron density problem in orbital-free density functional theory. If time allows it, I will also talk about the use of GFlowNets for the optimization of graph problems for the electronic structure problem in quantum computing.
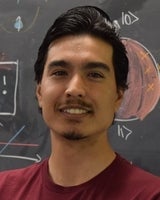