The University of Waterloo Cybersecurity and Privacy Institute was proud to host its Annual Conference on Thursday October 10, from 8:30 AM to 5:30 PM, at Federation Hall on the University of Waterloo Campus.
This event centred on our theme, “TACKLING CANADA’S CYBERSECURITY CHALLENGES", which highlighted current and future efforts within the cybersecurity and privacy sphere, with keynote speakers, panel discussions, and industry talks. This conference was open to undergraduate and graduate students, faculty, entrepreneurs, start ups, government, sponsors, and businesses.
Another primary objective of the conference was to shine a light on Waterloo graduate students, future leaders of cybersecurity research, through a poster session competition generously sponsored and judged by Rogers at the event. Out of 15 impactful research project submissions, the top prize of $1,000 was awarded to Abdulrahman Diaa, a PhD student in computer science, for his work on Optimizing Adaptive Attacks Against Content Watermarks for Language Models. Second place went to Sina Kamali ($500) and third place ($300) was shared by Yuzhe You and Jarvis Tse with prizes funded by CPI.
In the opinion of CPI’s acting executive director, Dr. Anindya Sen: "This conference brought together different societal stakeholders in discussing not only new academic research and the implications of technologies such as Large Language Models and other AI developments, but also the ramifications with respect to individual privacy. I am especially happy to see how successful the conference was in highlighting the outstanding research being done by our graduate students."
To view all of the 2024 poster session submissions and related abstracts, please visit the CPI website.
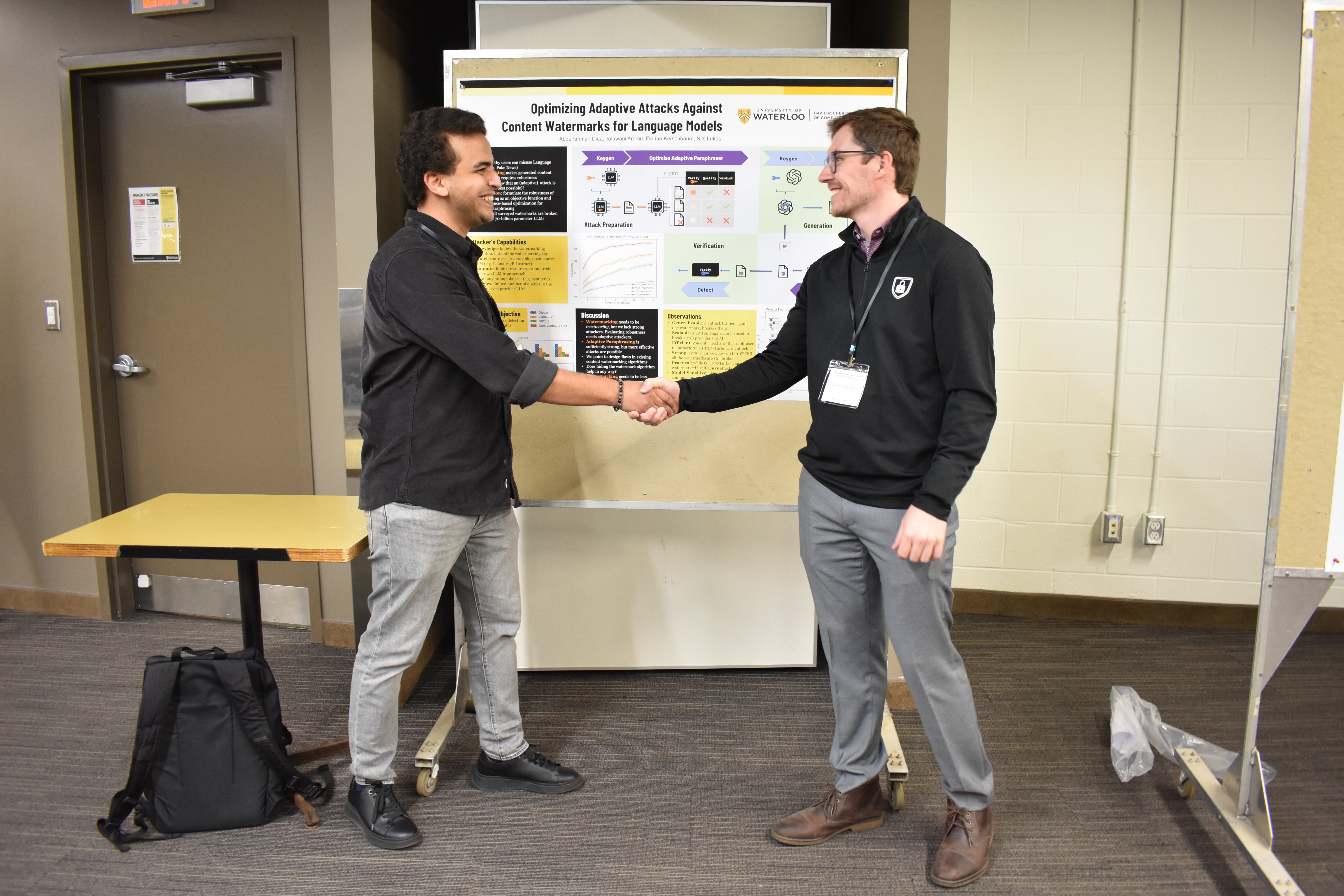
Congratulations to the following students for their respective wins:
First Place worth $1,000: Abdulrahman Diaa
Second Place worth $500: Sina Kamali
Third Place worth $300: Yuzhe You and Jarvis Tse
This year's first place prize money was generously donated by Rogers with second and third place being funded by CPI.
Abdulrahman Diaa - Optimizing Adaptive Attacks Against Content Watermarks for Language Models Supervisor: Florian Kerschbaum CS
Large Language Models (LLMs) can be \emph{misused} to spread online spam and misinformation. Content watermarking deters misuse by hiding a message in model-generated outputs, enabling their detection using a secret watermarking key. Robustness is a core security property, stating that evading detection requires (significant) degradation of the content's quality. Many LLM watermarking methods have been proposed, but robustness is tested only against \emph{non-adaptive} attackers who lack knowledge of the watermarking method and can find only suboptimal attacks. We formulate the robustness of LLM watermarking as an objective function and propose preference-based optimization to tune \emph{adaptive} attacks against the specific watermarking method. Our evaluation shows that (i) adaptive attacks substantially outperform non-adaptive baselines. (ii) Even in a non-adaptive setting, adaptive attacks optimized against a few known watermarks remain highly effective when tested against other unseen watermarks, and (iii) optimization-based attacks are practical and require less than seven GPU hours. Our findings underscore the need to test robustness against adaptive attackers.

Sina Kamali - Nika: Anonymous Blackout-Resistant Microblogging with Message Endorsing Supervisor: Diogo Barradas CS
Repressive governments are increasingly resorting to Internet shutdowns to control the flow of information during political unrest. In response, messaging apps built on top of mobile-based mesh networks have emerged as important communication tools for citizens and activists. While different flavors of these apps exist, those featuring microblogging functionalities are attractive for swiftly informing and mobilizing individuals. However, most apps fail to simultaneously uphold user anonymity while providing safe ways for users to build trust in others and the messages flowing through the mesh. We introduce Nika, a blackout-resistant app with two novel features: remote trust establishment and anonymous message endorsing. Nika also leverages a set of identity revocation primitives for the fine-grained management of trust relationships and to provide enhanced anonymity. Our evaluation of Nika through comprehensive micro-benchmarks and simulations showcases its practicality and resilience in shutdown scenarios.
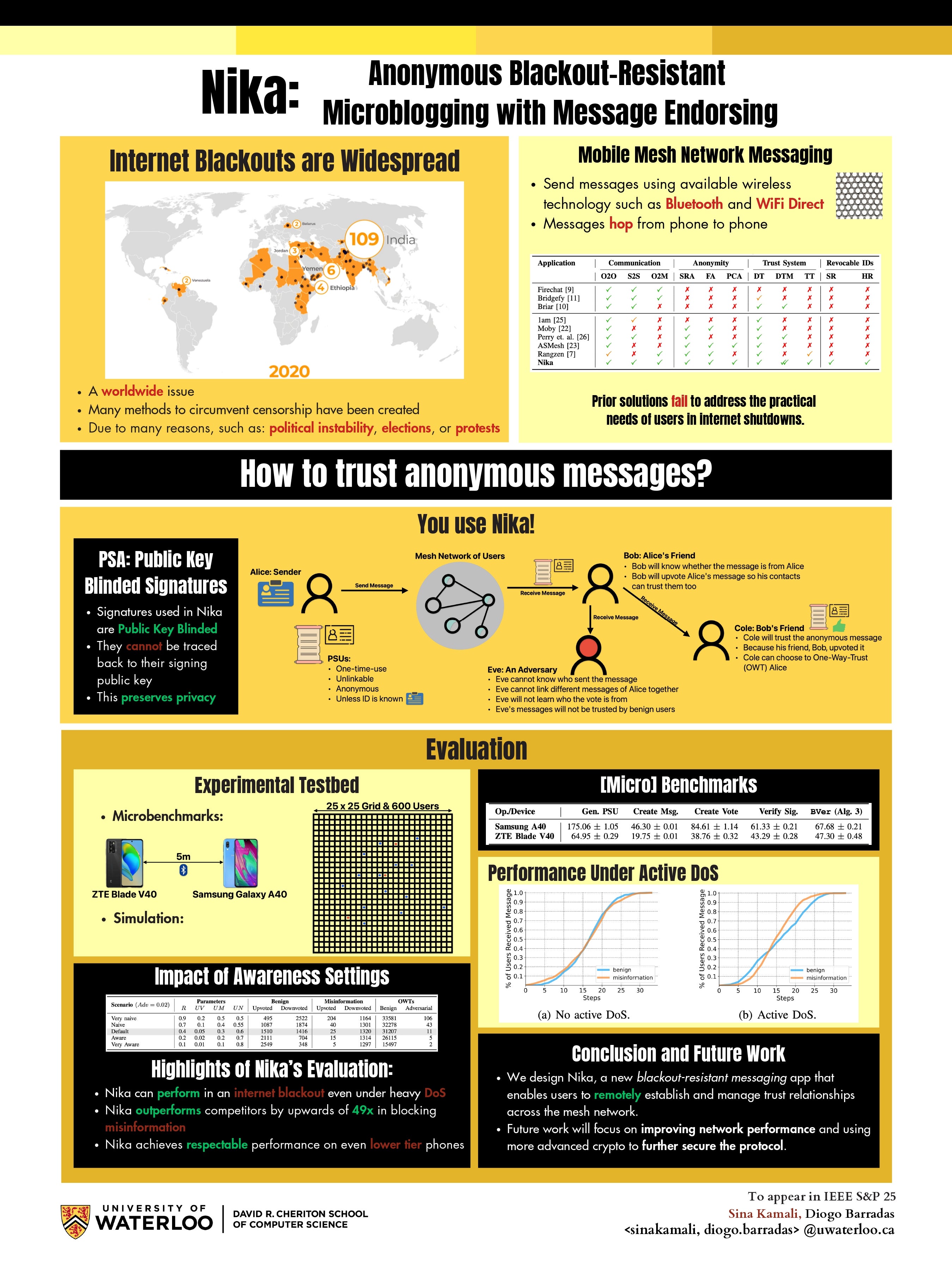
Yuzhe You and Jarvis Tse - AdvEx: Understanding Adversarial Attacks with Interactive Visualizations Supervisor: Jian Zhao CS
Adversarial machine learning (AML) focuses on studying attacks that can fool machine learning algorithms into generating incorrect outcomes as well as the defenses against worst-case attacks to strengthen the adversarial robustness of machine learning models. Specifically for image classification tasks, it is difficult to comprehend the underlying logic behind adversarial attacks due to two key challenges: 1) the attacks exploiting “non-robust” features that are not human-interpretable and 2) the perturbations applied being almost imperceptible to human eyes. We propose an interactive visualization system, AdvEx, that presents the properties and consequences of evasion attacks as well as provides data and model performance analytics on both instance and population levels. We quantitatively and qualitatively assessed AdvEx in a two-part evaluation including user studies and expert interviews. Our results show that AdvEx is effective both as an educational tool for understanding AML mechanisms and a visual analytics tool for inspecting machine learning models, which can benefit both AML learners and experienced practitioners.
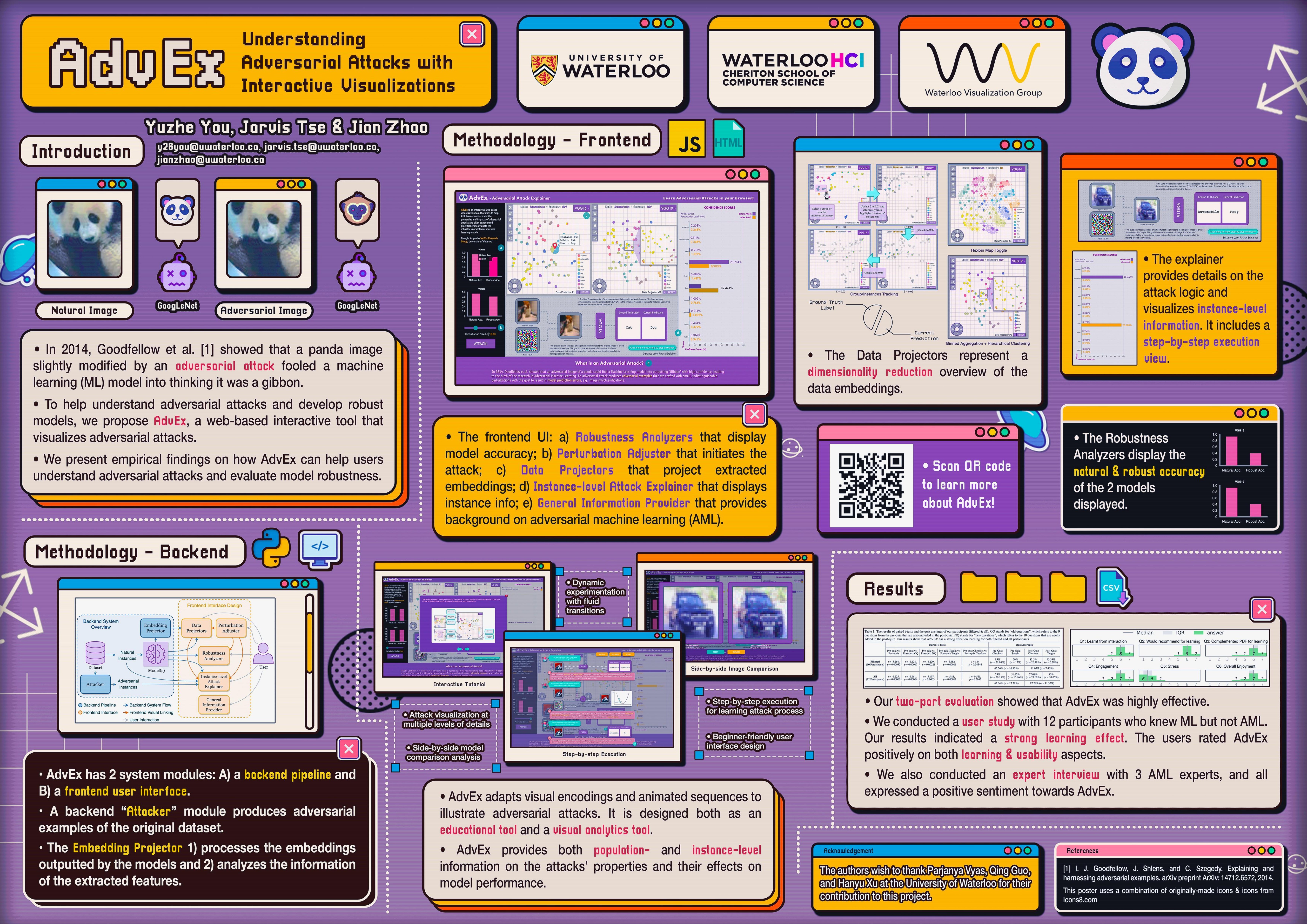